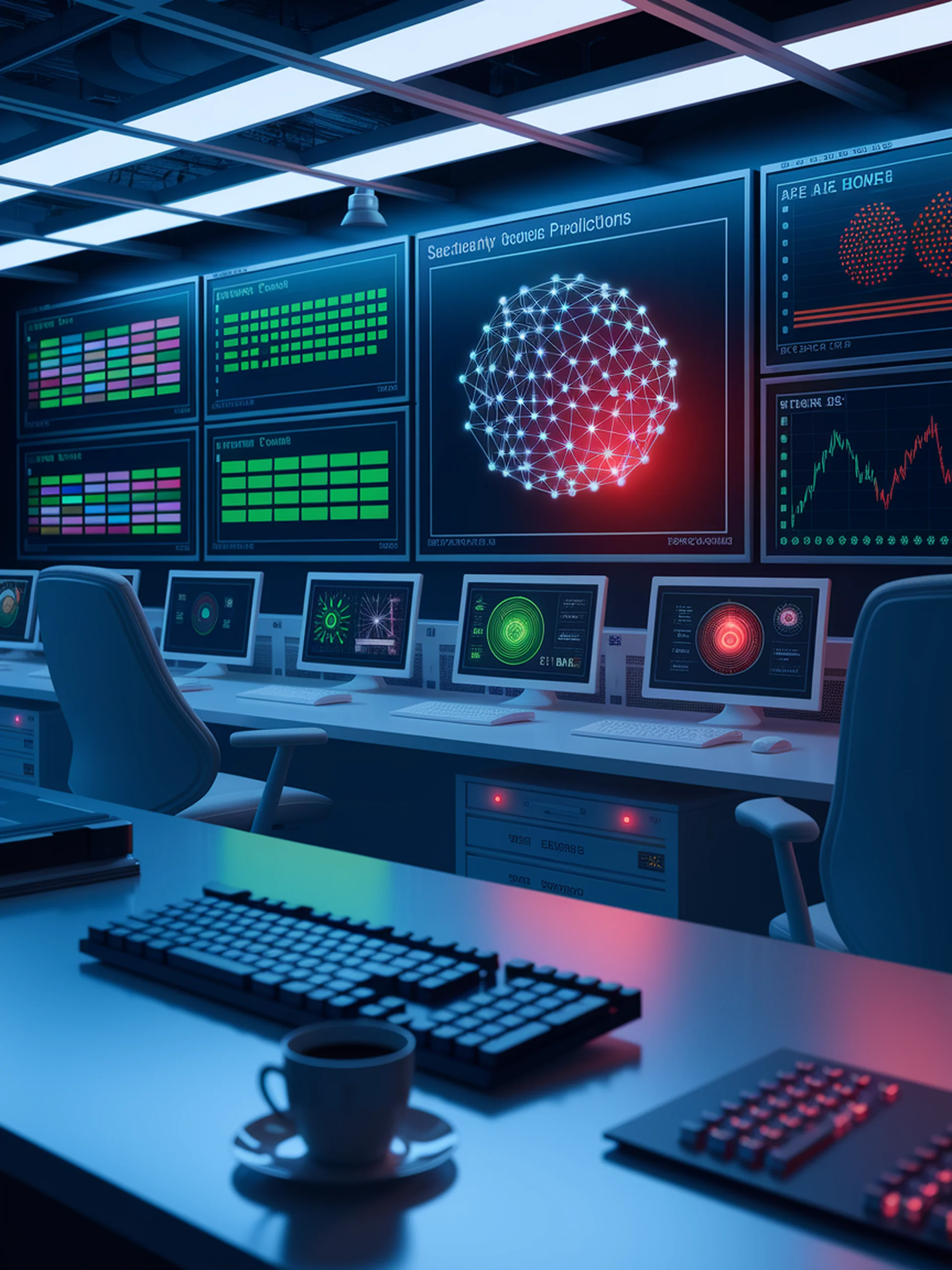
Predicting When LLMs Will Fail
A Framework for Safer AI by Making Failures Predictable
PredictaBoard introduces a novel benchmarking framework for evaluating how accurately we can predict when large language models will succeed or fail at tasks.
- Addresses unpredictable failures in LLMs, even on simple reasoning tasks
- Creates metrics to evaluate the reliability of score predictors
- Enables the identification of "safe zones" for LLM operation
- Provides a foundation for developing more reliable AI systems
This research is critical for security applications where unpredictable AI failures could have serious consequences. By improving our ability to anticipate when LLMs might fail, organizations can establish safer operational boundaries and implement appropriate safeguards.