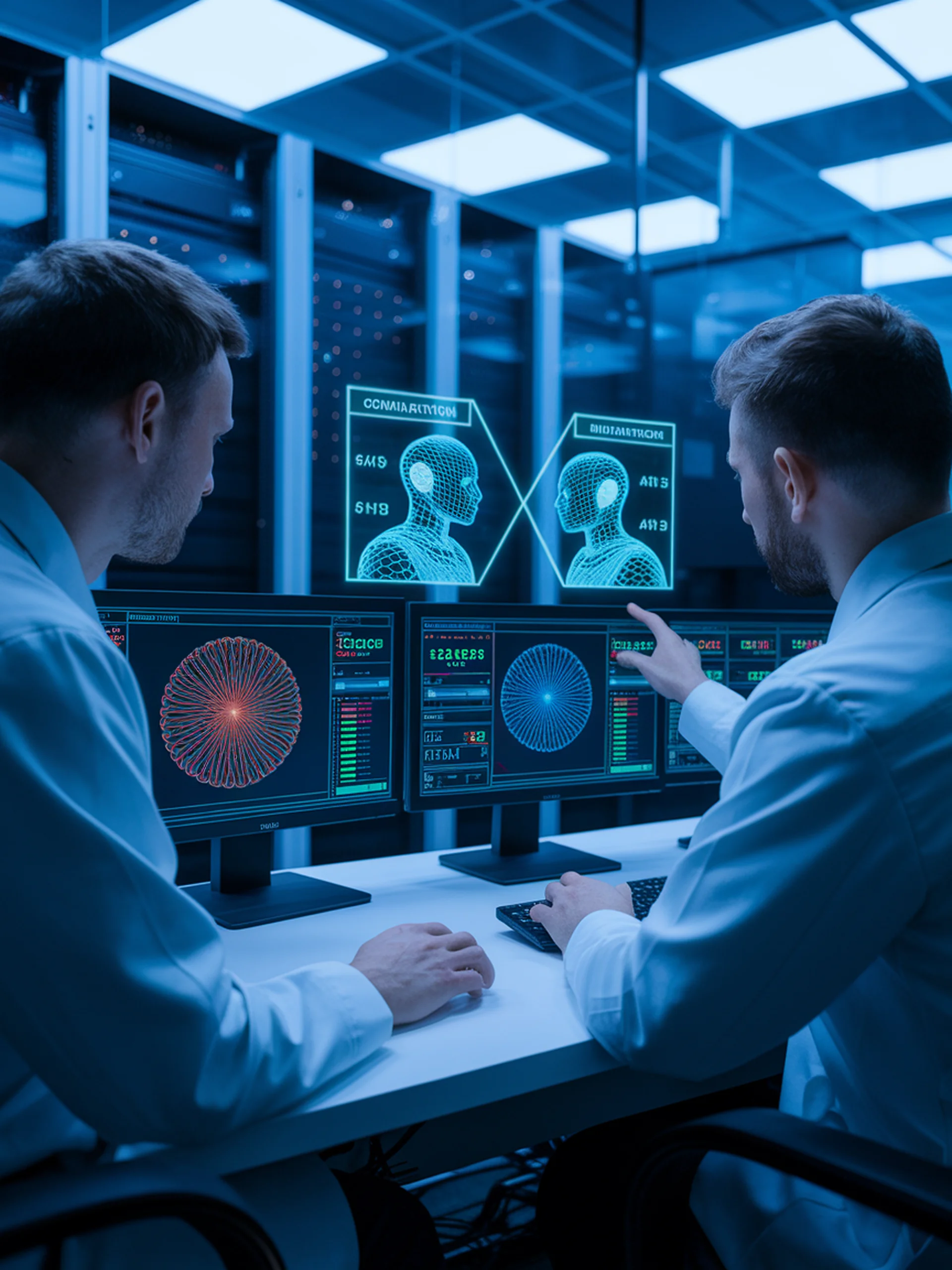
Beyond Self-Consistency: Detecting LLM Hallucinations
Leveraging cross-model verification to improve hallucination detection
This research addresses a critical security challenge in AI by developing more effective methods to detect hallucinations in Large Language Models (LLMs).
- Current self-consistency techniques for hallucination detection are approaching their performance ceiling
- The paper introduces cross-model verification as a more reliable approach
- Demonstrates significant improvement in hallucination detection through model collaboration
- Particularly valuable for sensitive applications where reliability is critical
Verify when Uncertain: Beyond Self-Consistency in Black Box Hallucination Detection