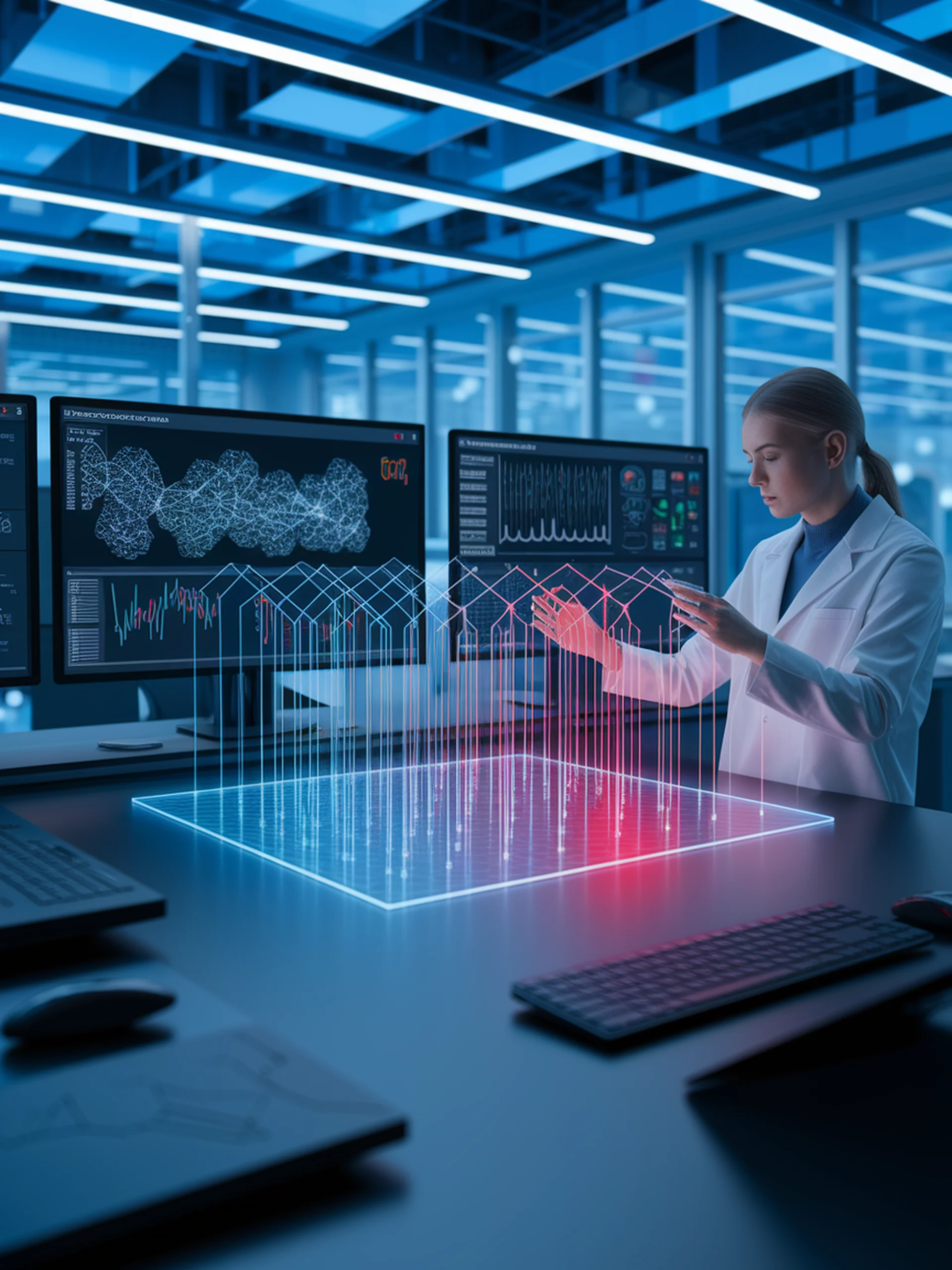
Detecting AI Hallucinations with Semantic Volume
A new method to measure both internal and external uncertainty in LLMs
This research introduces Semantic Volume, a novel metric that quantifies uncertainty in large language models by measuring the semantic space of model outputs.
- Dual uncertainty detection: Uniquely identifies both internal uncertainty (model knowledge gaps) and external uncertainty (ambiguous inputs)
- Outperforms existing methods: Shows superior performance in hallucination detection across multiple benchmarking datasets
- Low computational overhead: Requires only a small set of output samples to effectively quantify uncertainty
- Model-agnostic approach: Works across different LLM architectures without requiring model modifications
For security professionals, this research provides a practical technique to detect potentially harmful or misleading AI outputs before deployment in critical systems, significantly reducing risks associated with AI hallucinations.
Semantic Volume: Quantifying and Detecting both External and Internal Uncertainty in LLMs