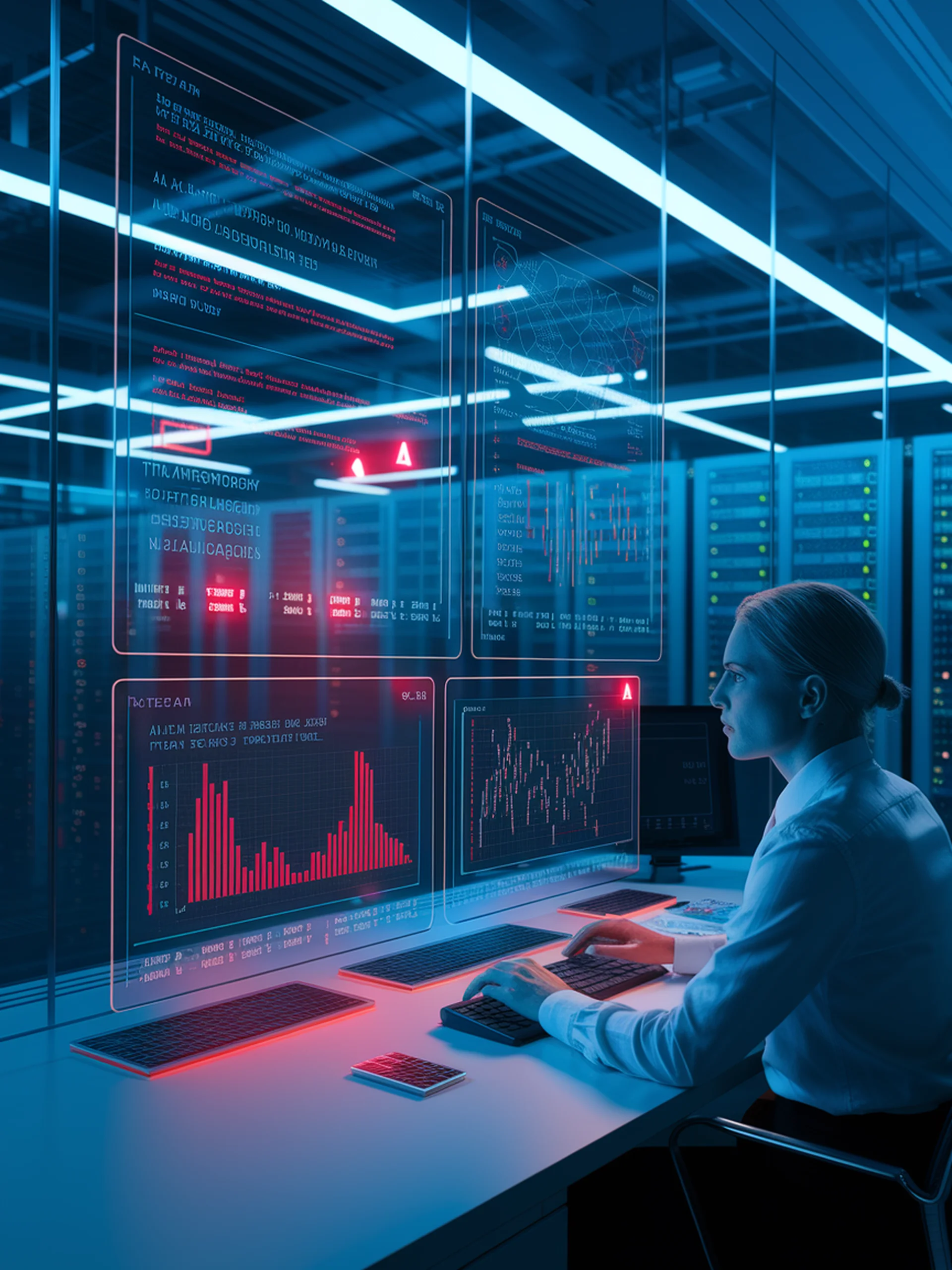
Smarter Hallucination Detection in LLMs
Enhancing AI safety through adaptive token analysis
This research introduces a robust hallucination detection method that dynamically analyzes LLM outputs to identify false information, significantly improving security and trustworthiness.
- Develops an adaptive token selection strategy that flexibly identifies the most relevant tokens for analysis regardless of text length or structure
- Achieves superior performance across various LLM architectures, outperforming previous detection methods
- Demonstrates consistent reliability when detecting hallucinations in diverse, free-form text generations
- Addresses critical safety concerns that currently limit broader LLM deployment in high-stakes applications
This advancement enables more confident use of LLMs in sensitive domains like healthcare, finance, and legal applications by providing a reliable mechanism to flag potentially hallucinated content.
Robust Hallucination Detection in LLMs via Adaptive Token Selection