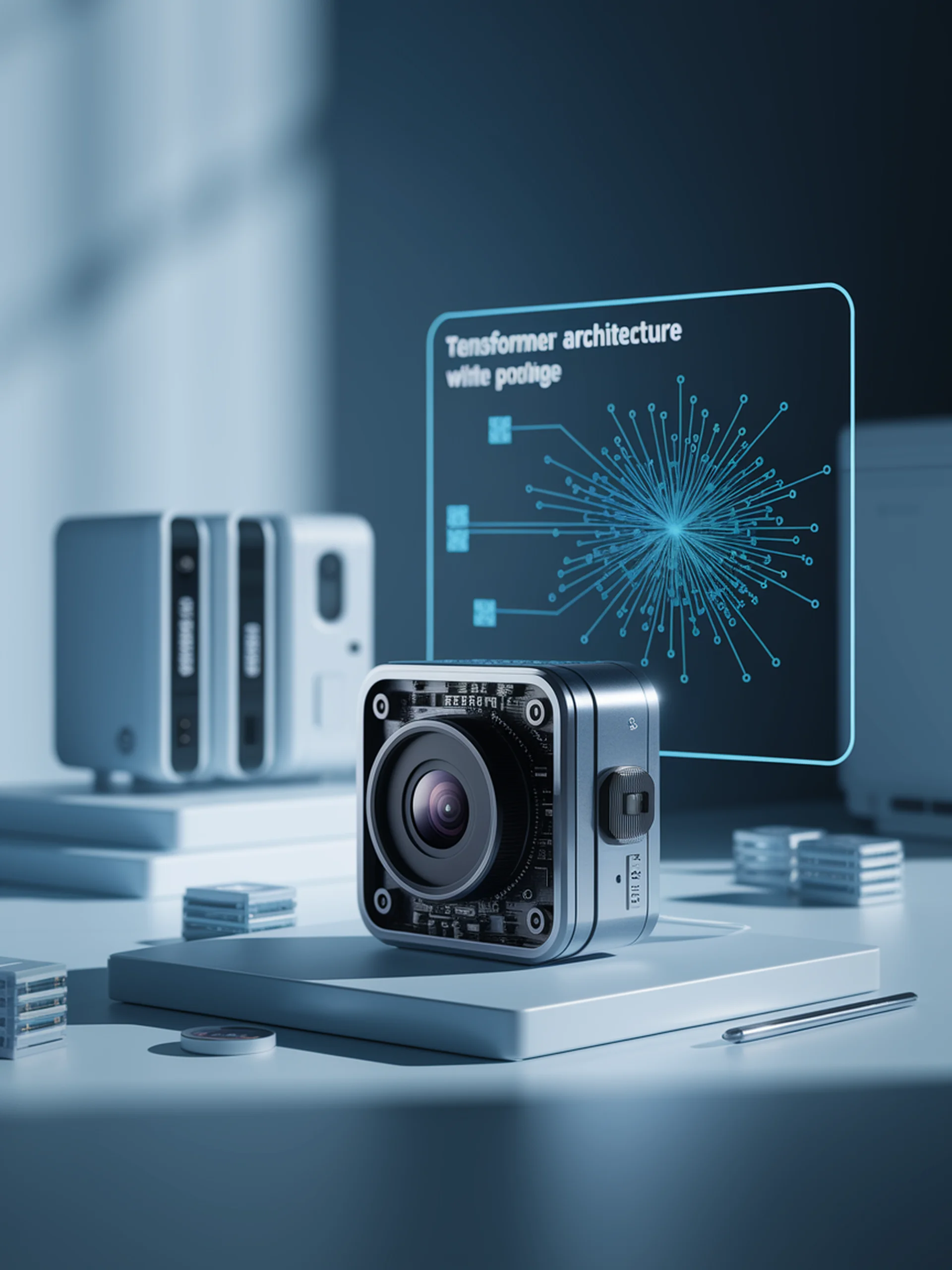
Smarter, Smaller Vision Transformers
Strategic Pruning for Domain Generalization and Resource Efficiency
This research introduces a grouped structural pruning method for vision transformers that significantly reduces model size while maintaining performance across different domains.
- Analyzes dependency graphs to identify and remove redundant components
- Evaluated on ViT, BeiT, and DeiT models across PACS and Office-Home benchmarks
- Enables deployment on resource-constrained devices without sacrificing accuracy
- Particularly valuable for domain generalization tasks
The engineering significance lies in enabling powerful vision models to run efficiently on edge devices, addressing the growing challenge of deploying increasingly large AI models in resource-limited environments.
The Effects of Grouped Structural Global Pruning of Vision Transformers on Domain Generalisation