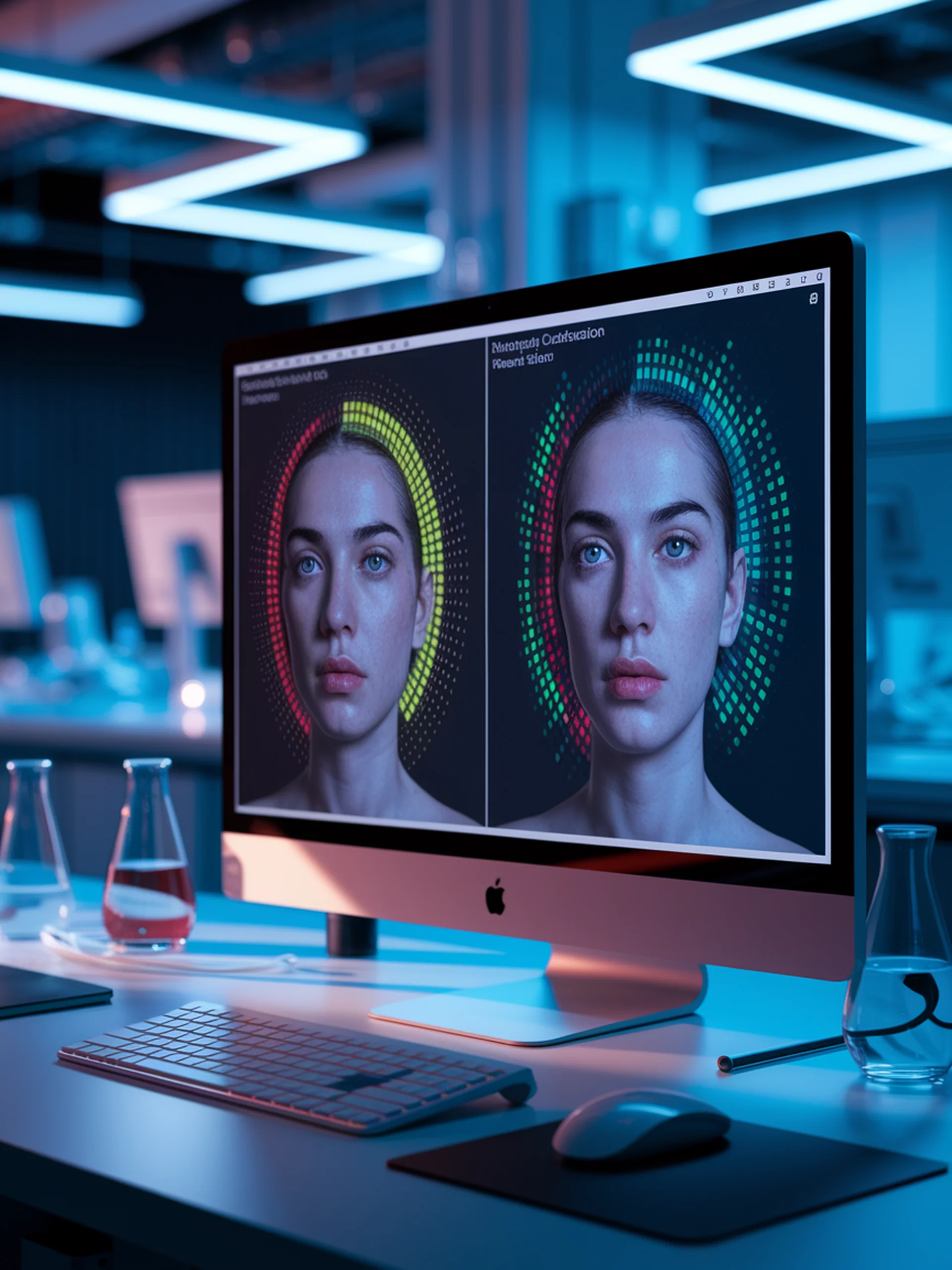
Explaining the Uncertainty Gap in AI Vision
Revealing why image classifiers lack confidence through counterfactuals
This research introduces an innovative approach to understand and explain why AI vision models lack confidence in certain predictions, addressing a critical knowledge gap in explainable AI.
- Develops counterfactual images to visualize what changes would increase model confidence
- Provides a framework for interpreting model uncertainty beyond simple confidence scores
- Enables better detection of when and why vision systems might fail
- Creates foundations for more transparent and reliable AI systems
From a security perspective, this work helps identify potential weak points in vision systems where misclassification risks exist, allowing for targeted improvements and safer deployment in critical applications.
Explaining Low Perception Model Competency with High-Competency Counterfactuals