Watermarking and Attribution for LLM Content
Research on embedding identifiable markers in LLM outputs for content attribution, detection of AI-generated content, and resistance to watermarking attacks
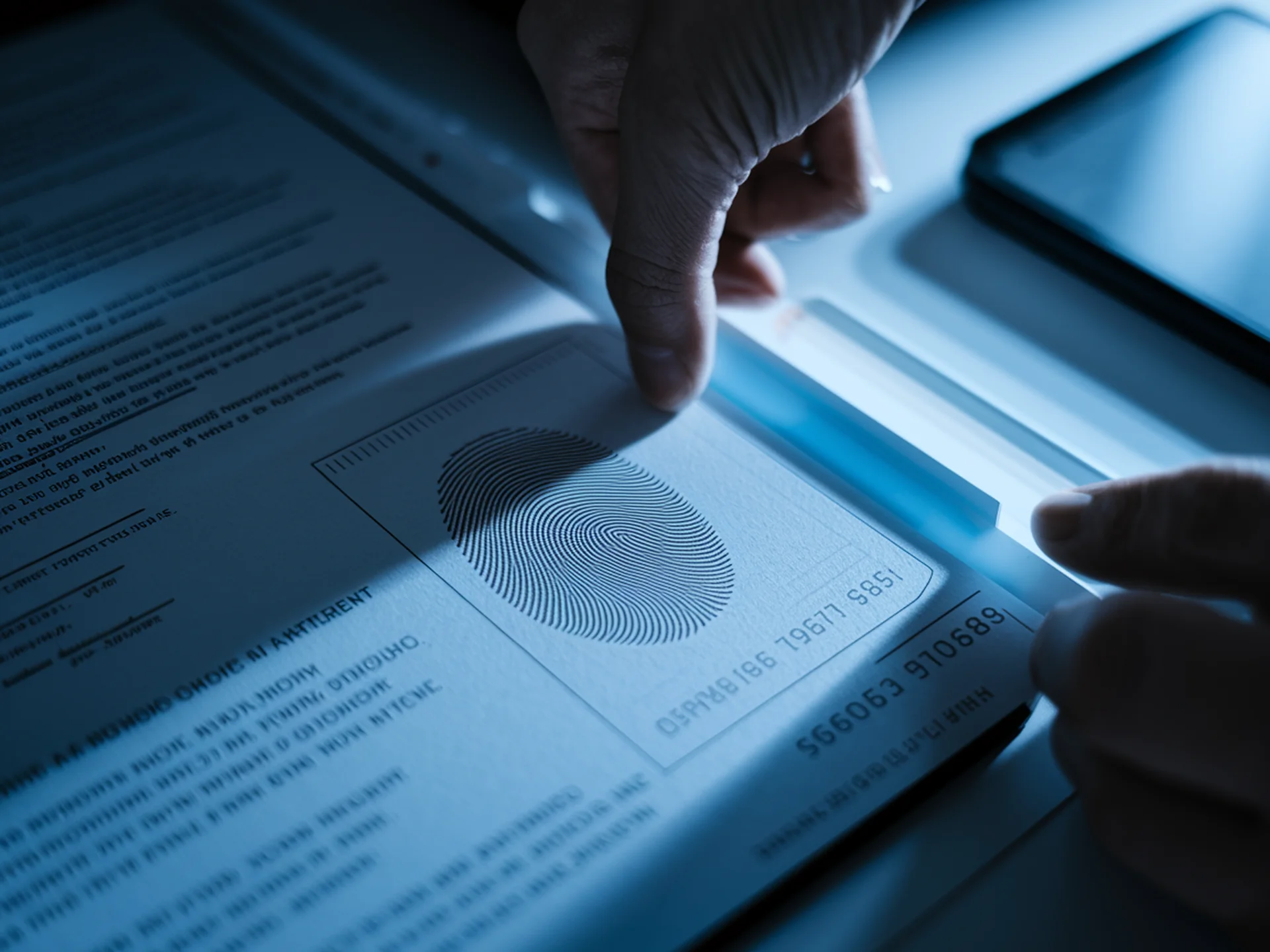
Watermarking and Attribution for LLM Content
Research on Large Language Models in Watermarking and Attribution for LLM Content
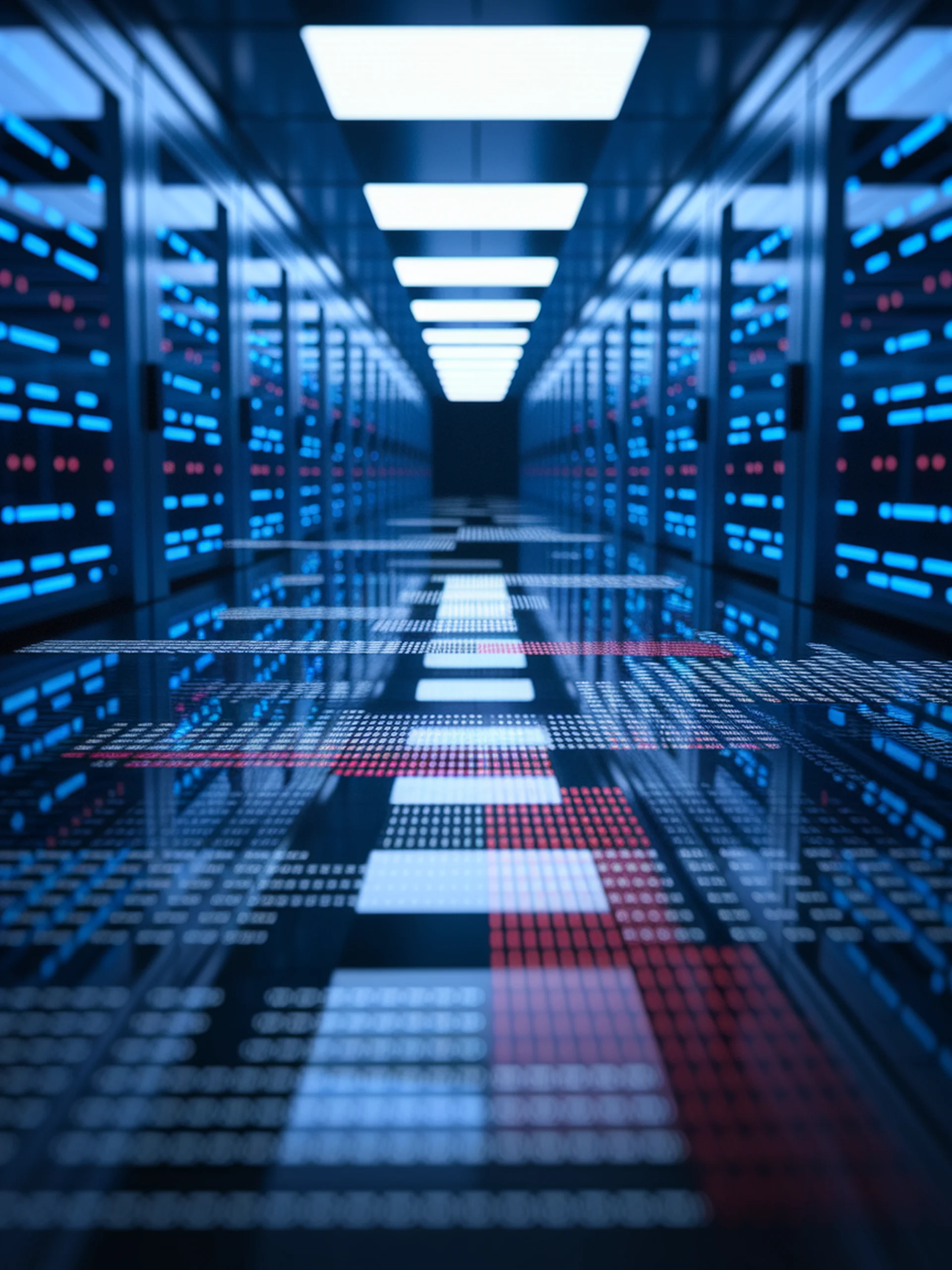
Watermark Collisions in LLMs
Uncovering Vulnerabilities in Text Copyright Protection Systems
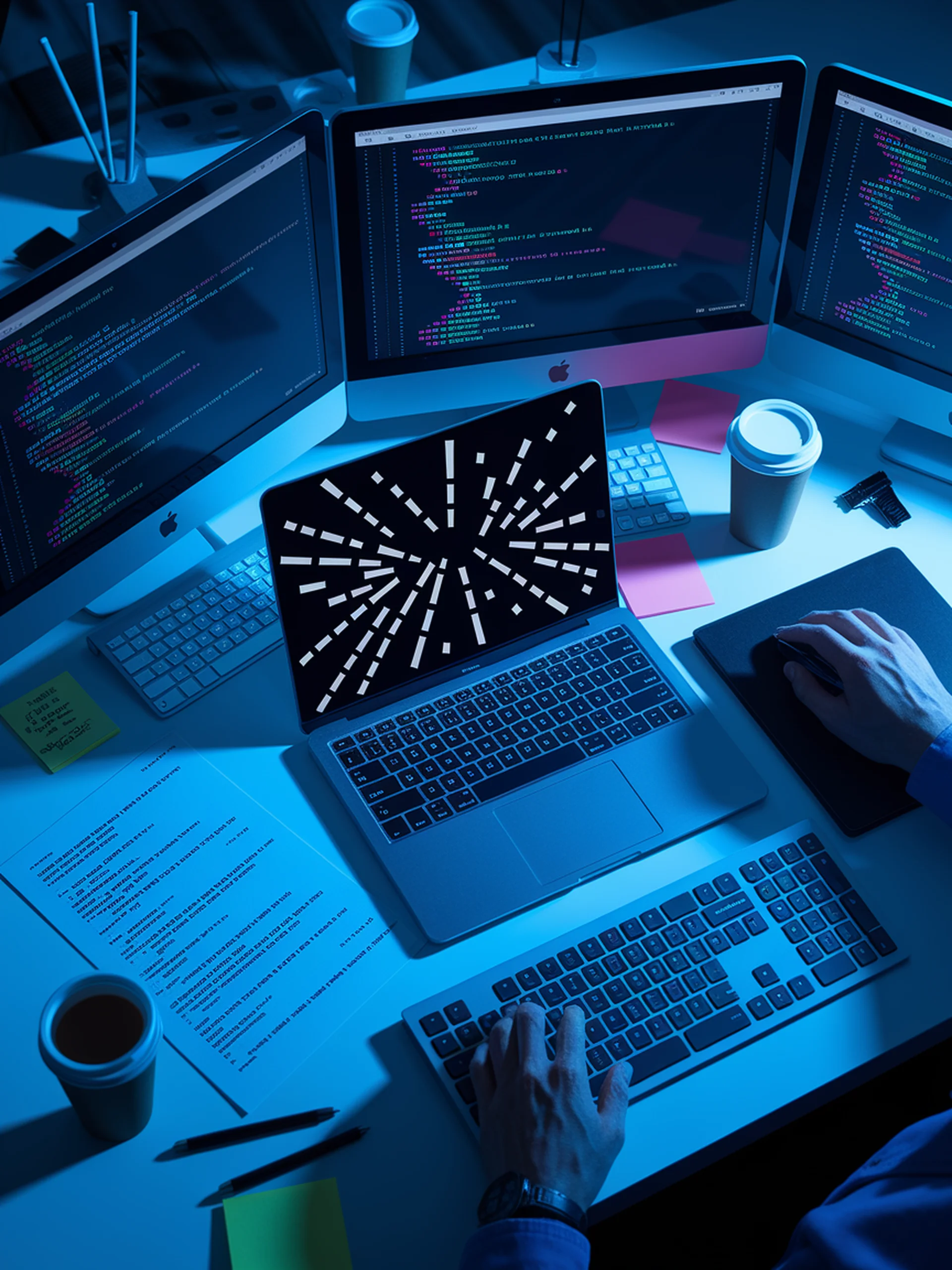
Cracking the Code Watermark
Why current LLM code watermarking fails under attack
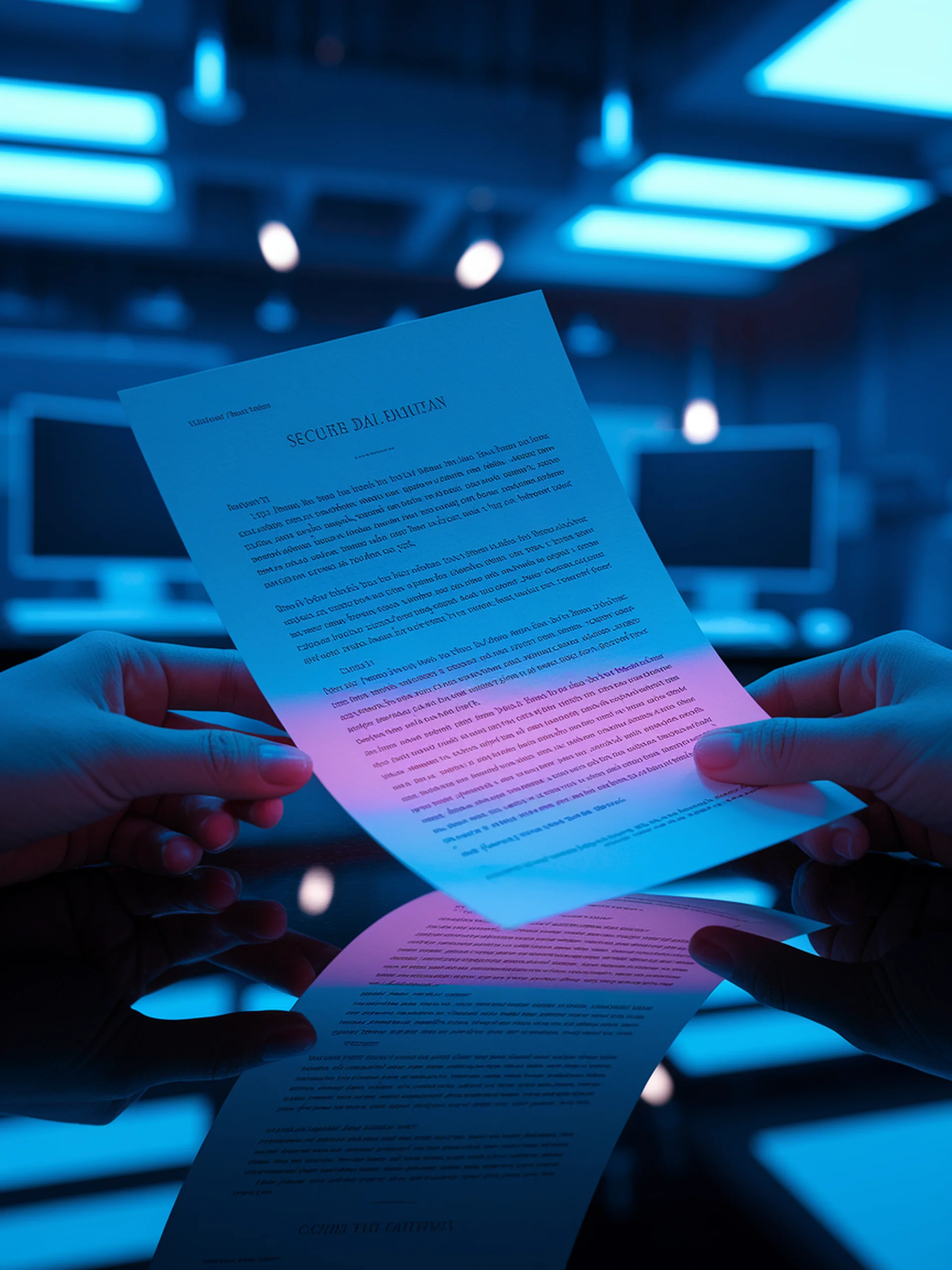
Securing AI Content with Topic-Based Watermarking
A novel approach to authenticate LLM-generated text with minimal quality impact
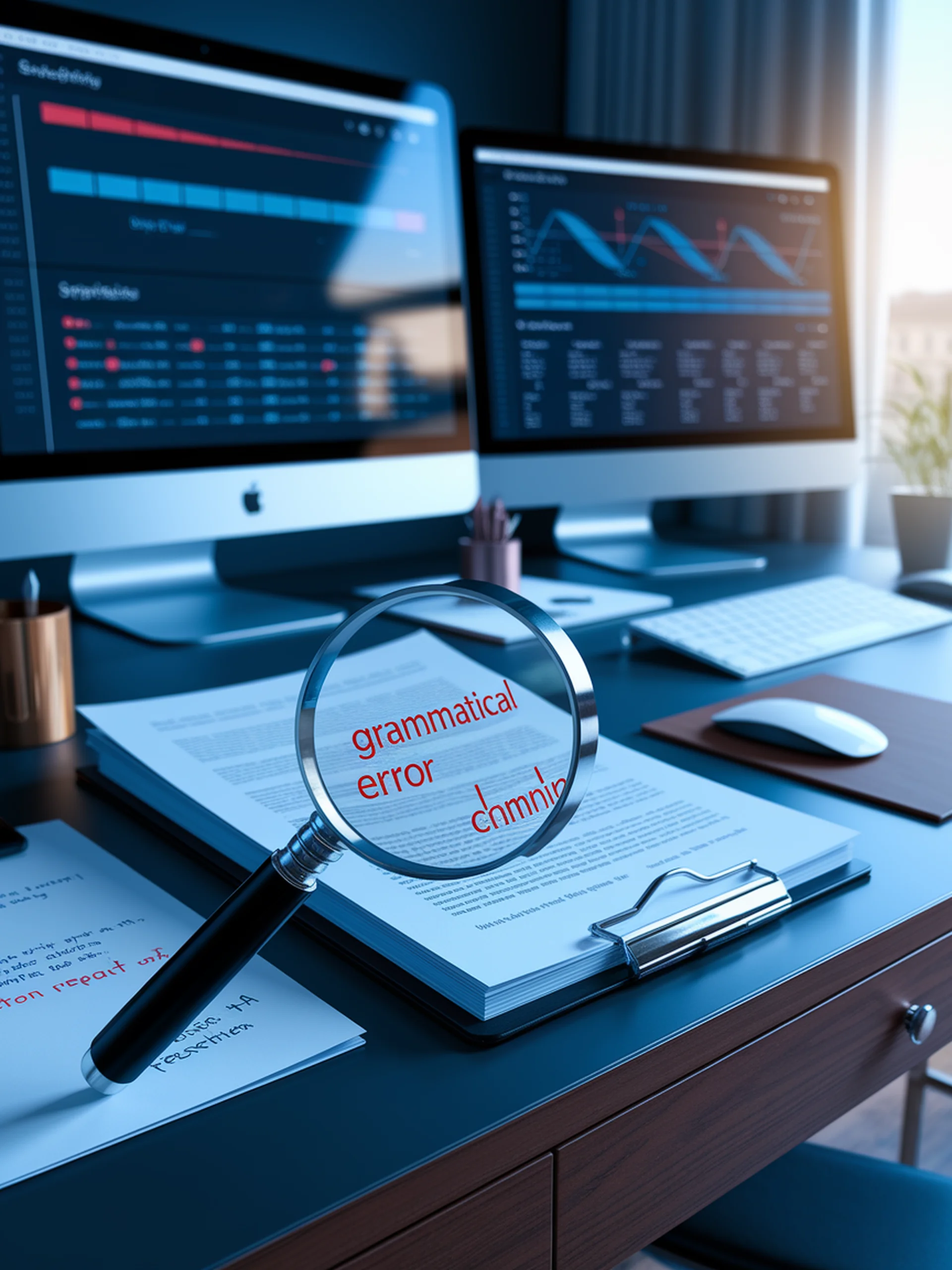
Spotting AI-Written Text Without Training Data
Using grammatical error patterns for zero-shot LLM text detection
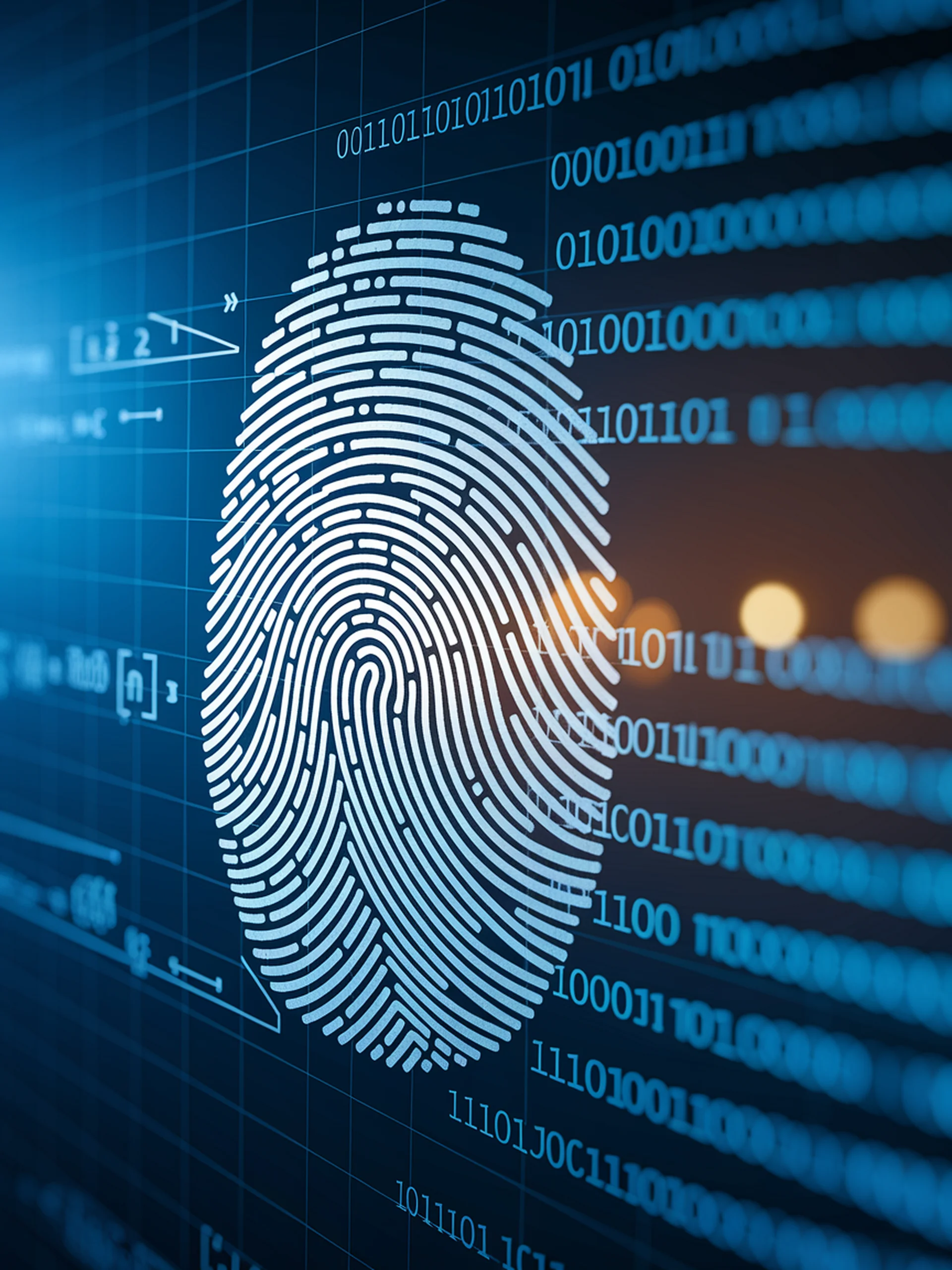
The Fingerprint of AI Text
Mathematical proof reveals statistical pattern in all AI-generated content
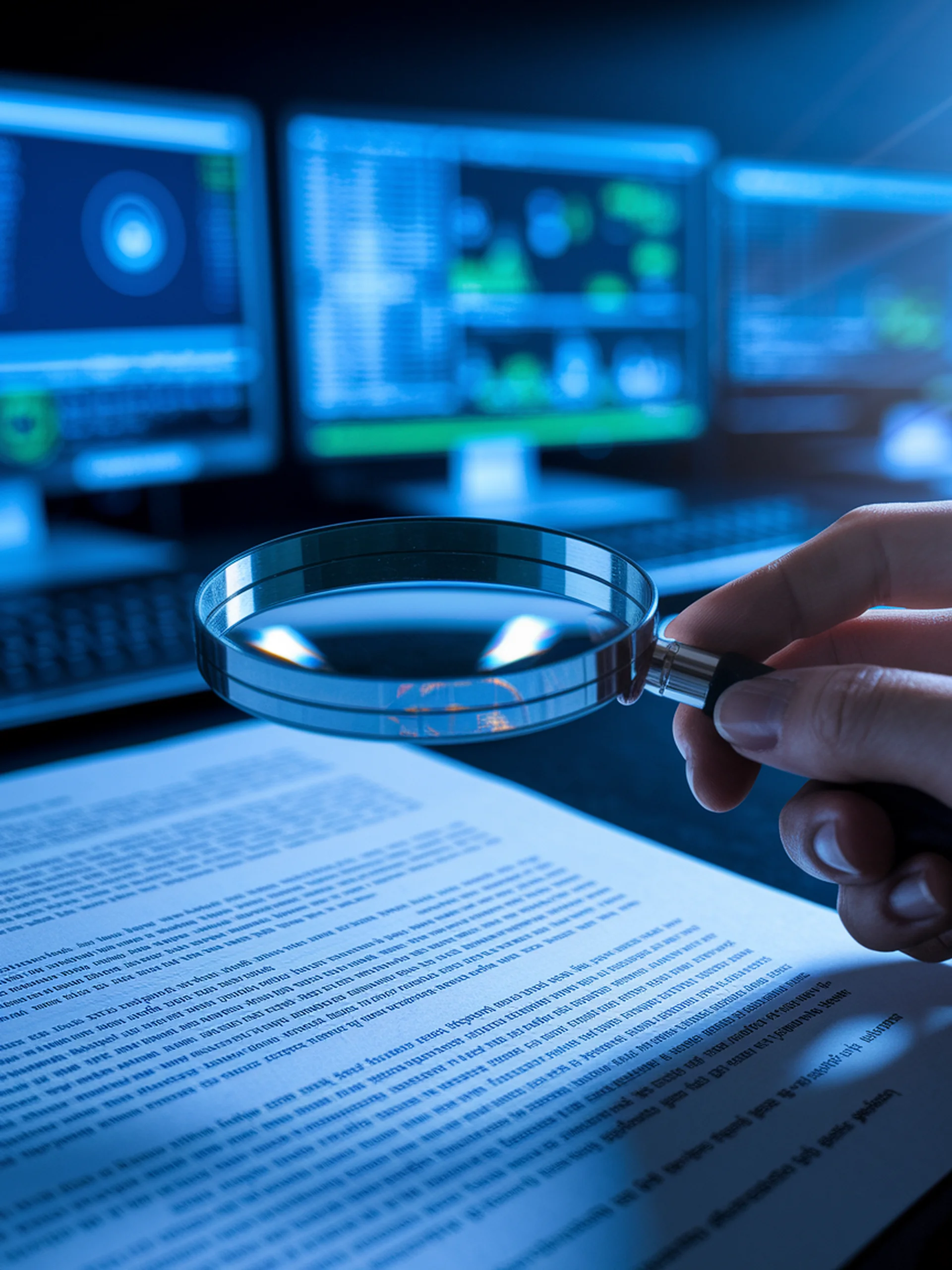
Secure Watermarking for AI Content Detection
An unbiased approach to identifying LLM-generated text
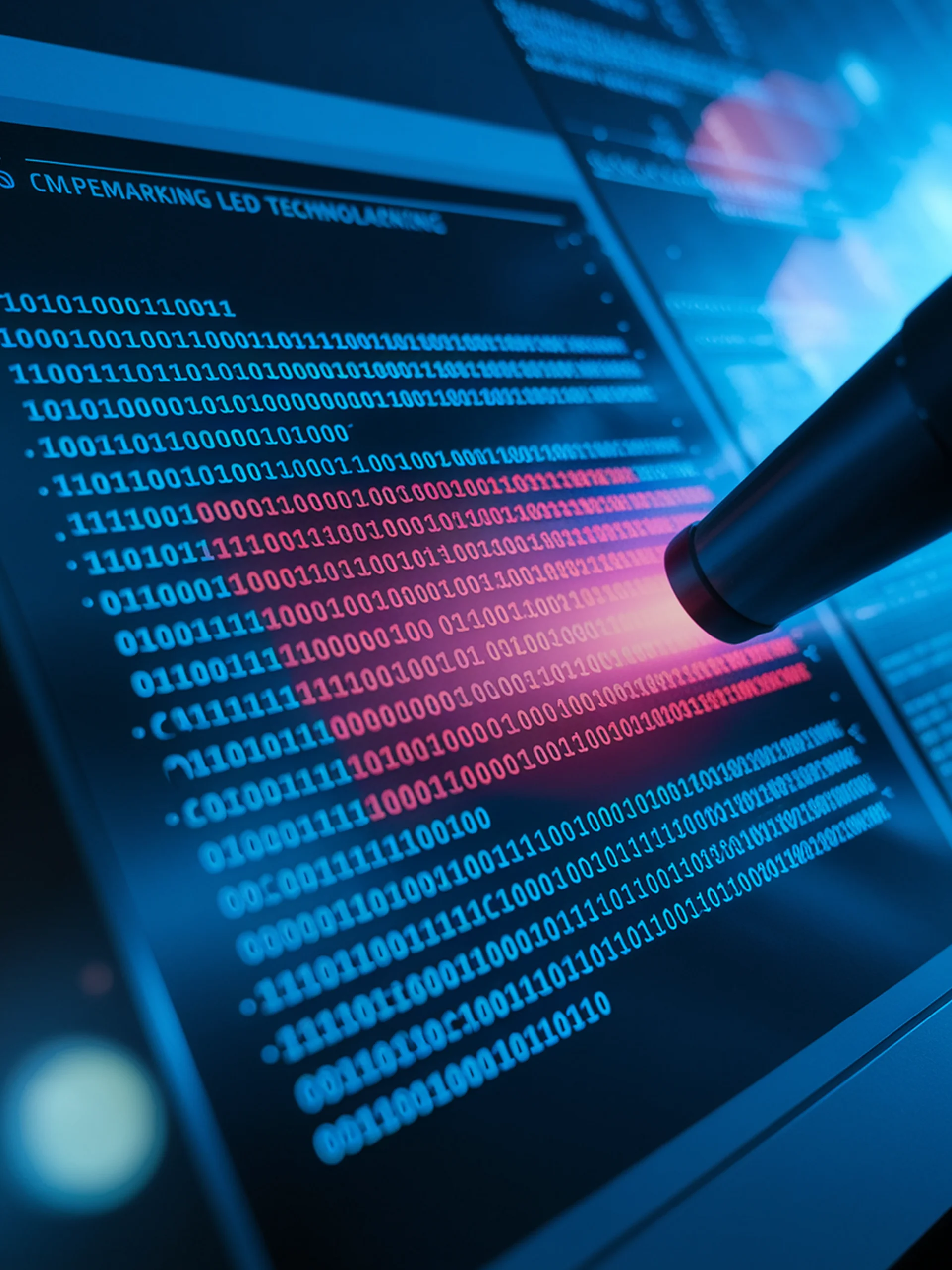
Invisible Fingerprints for AI Text
Watermarking LLMs with Error Correcting Codes
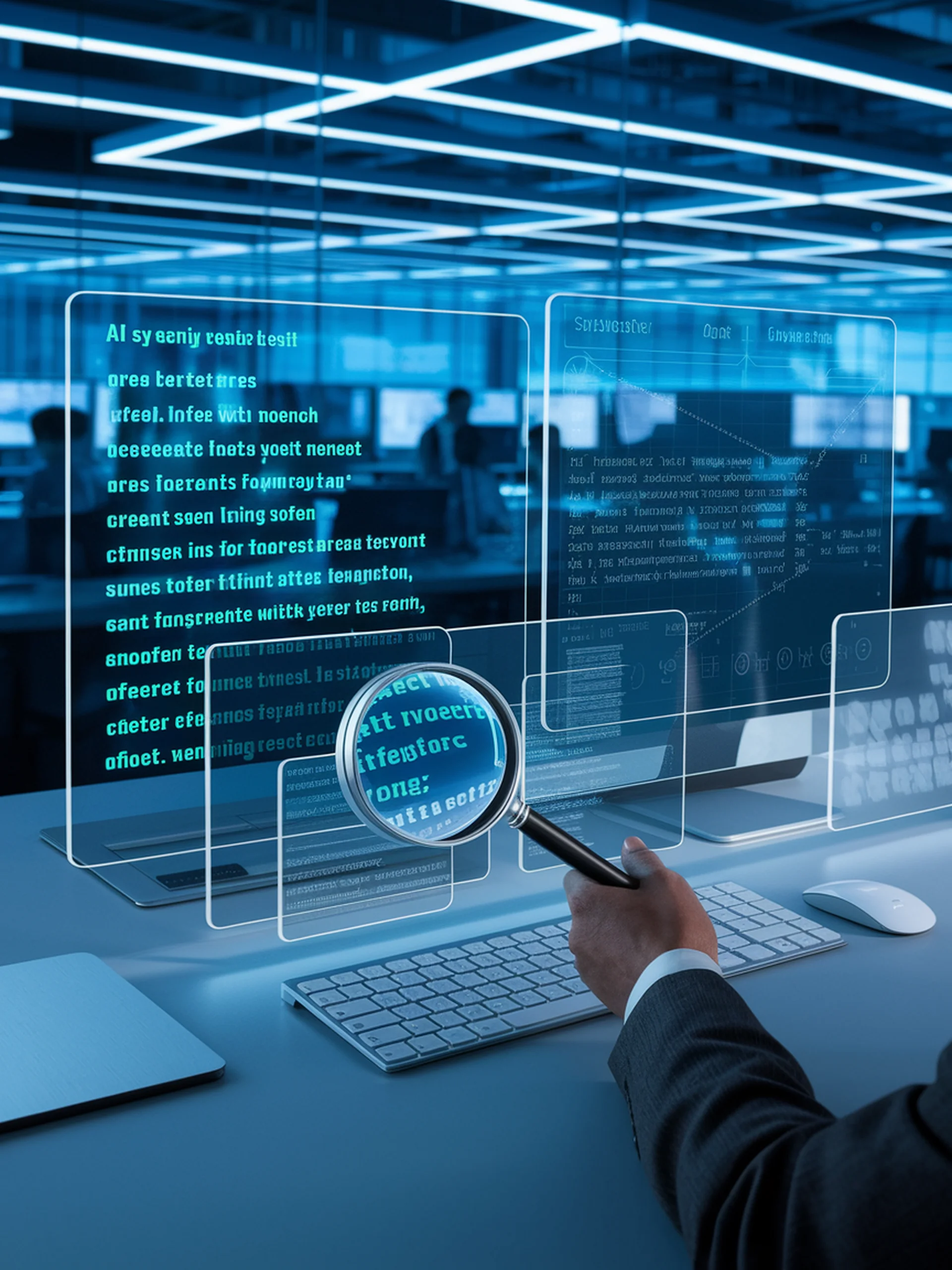
The Challenge of Detecting AI-Written Content
Factors that impact our ability to identify machine-generated text
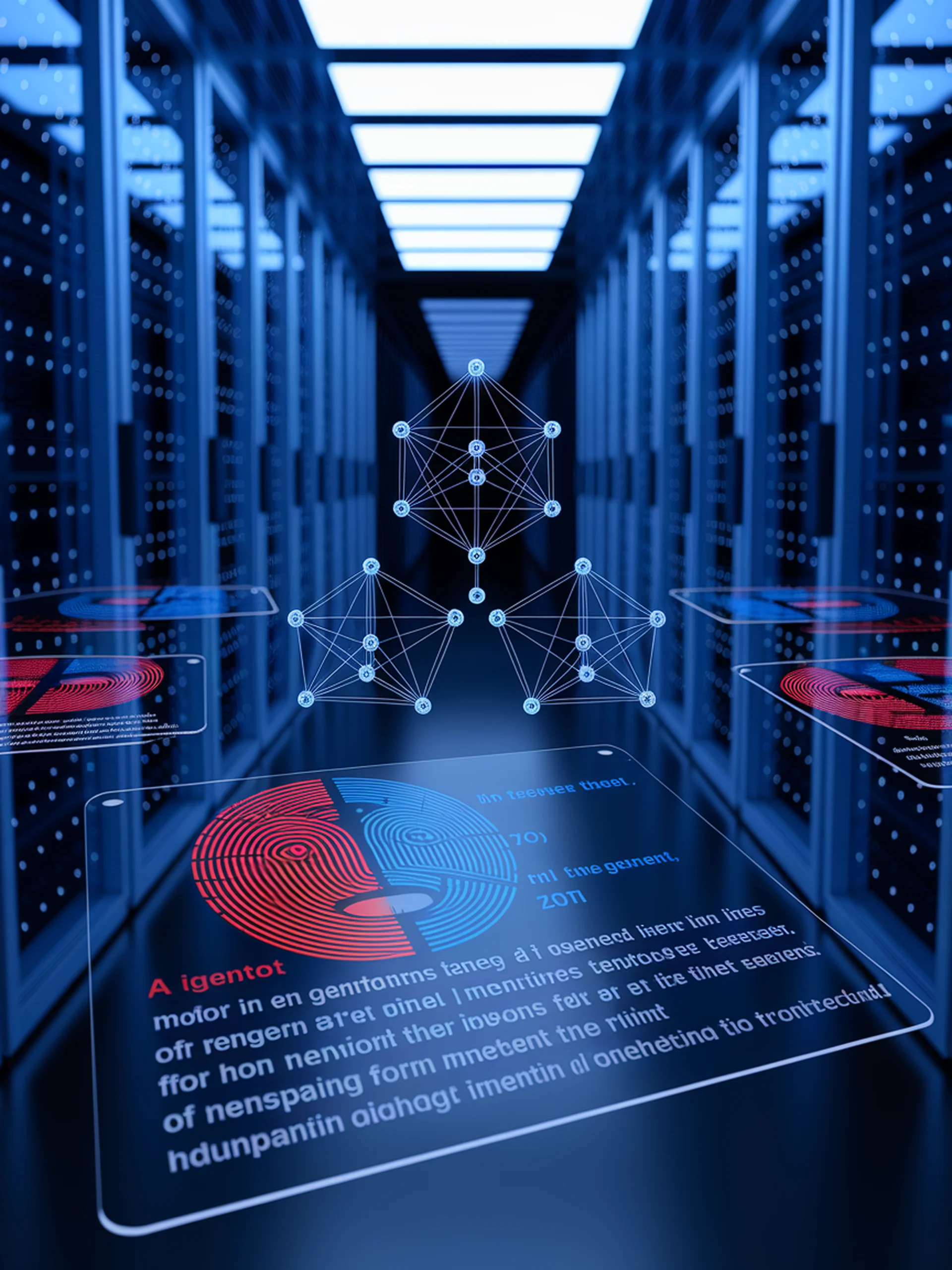
Detecting AI-Generated Text
A novel contrastive learning approach for identifying LLM outputs
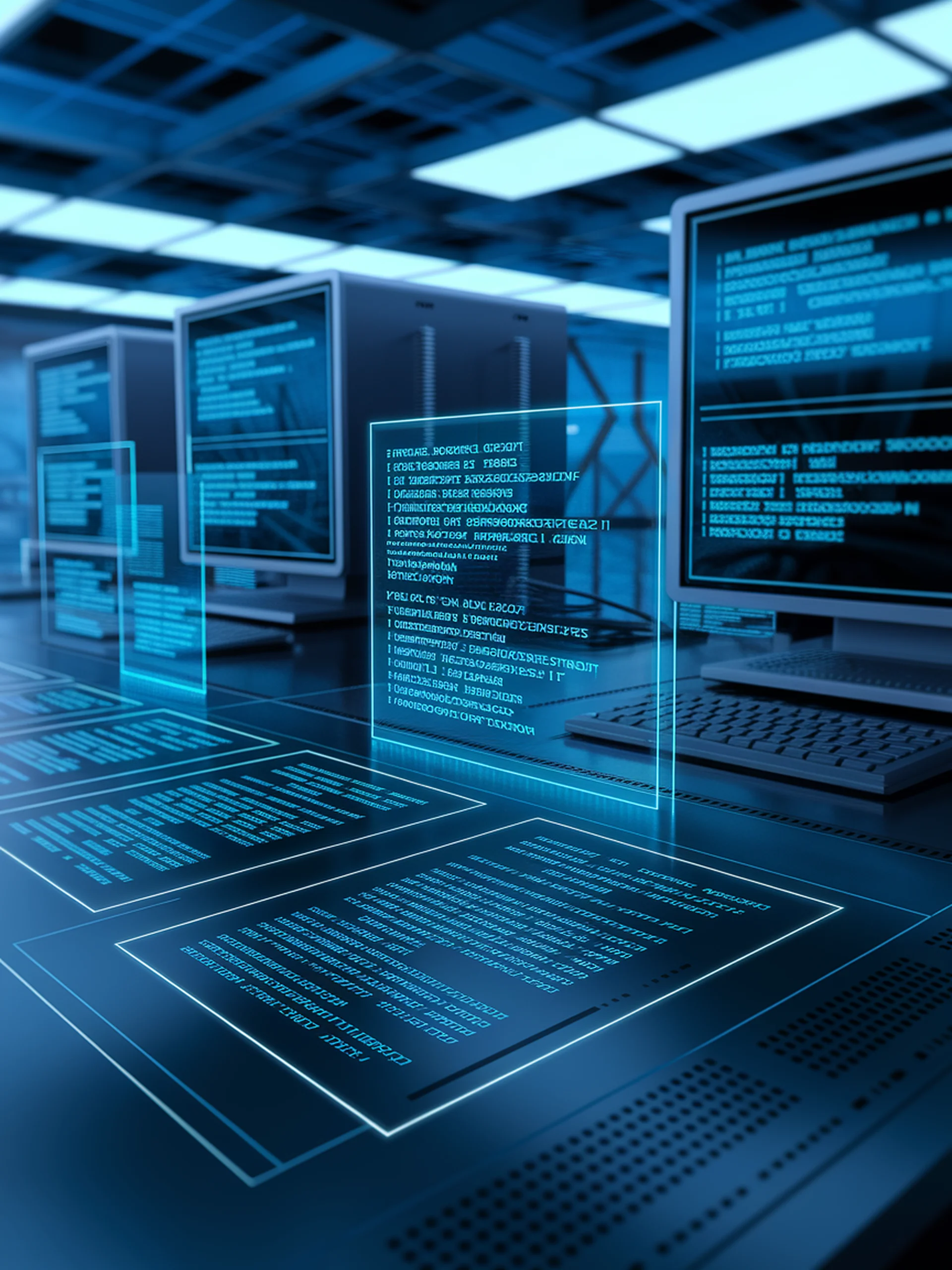
Watermarking LLMs for Copyright Protection
Preventing unauthorized content generation while maintaining security
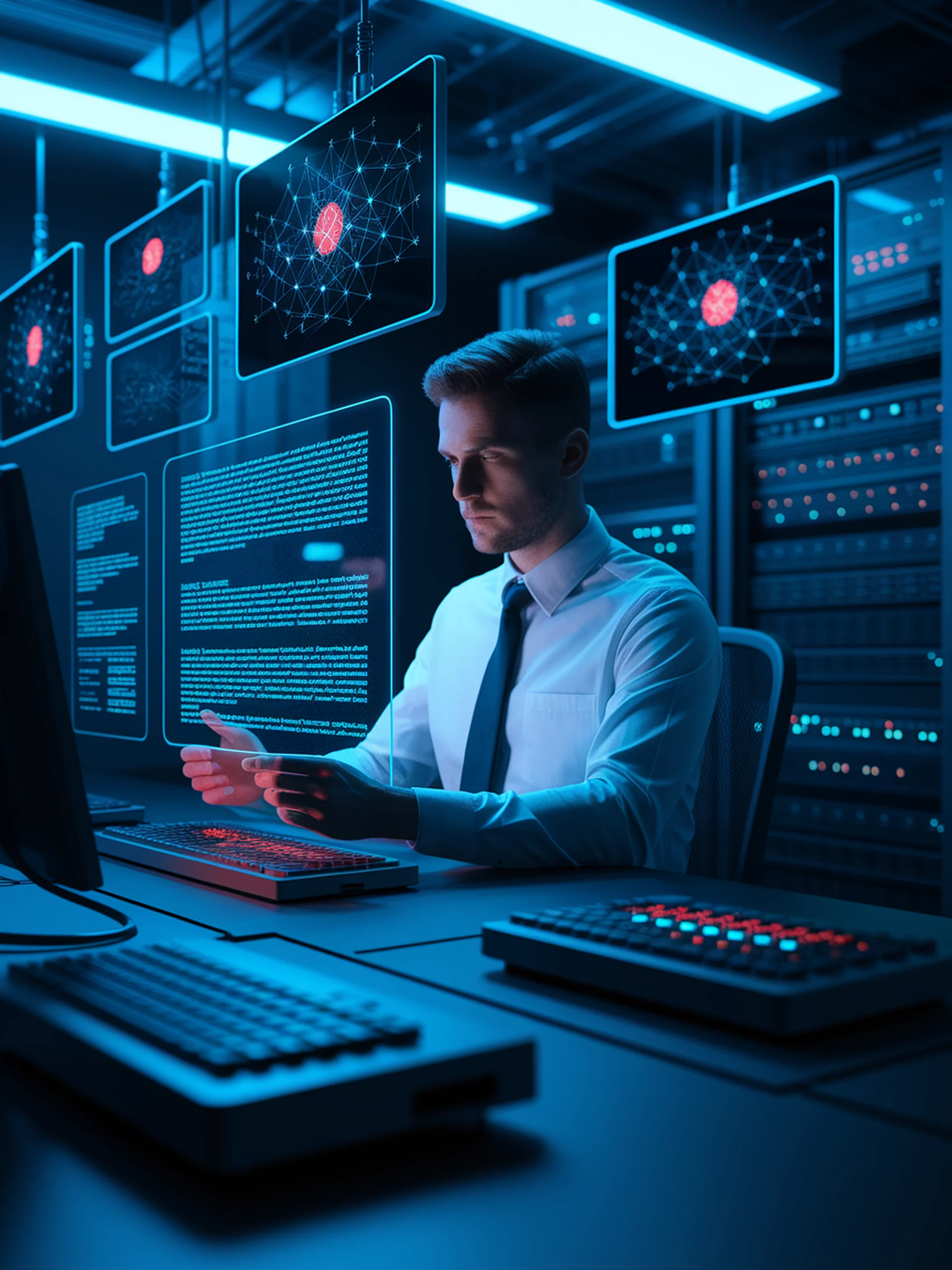
Detecting AI Deception
A breakthrough approach to identify LLM-generated content across domains
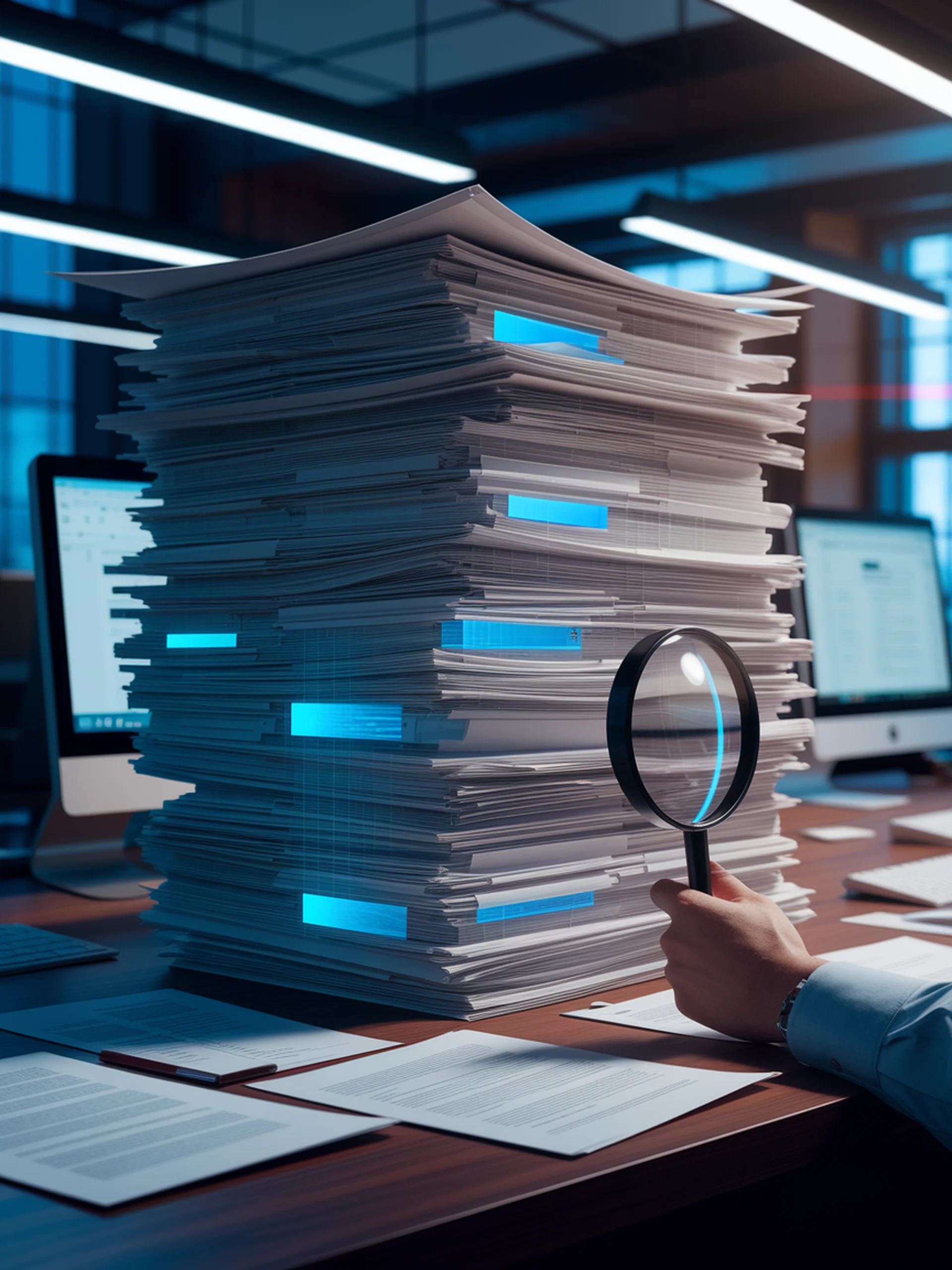
Finding the Needle in the Haystack
Efficiently detecting AI-generated content within large documents
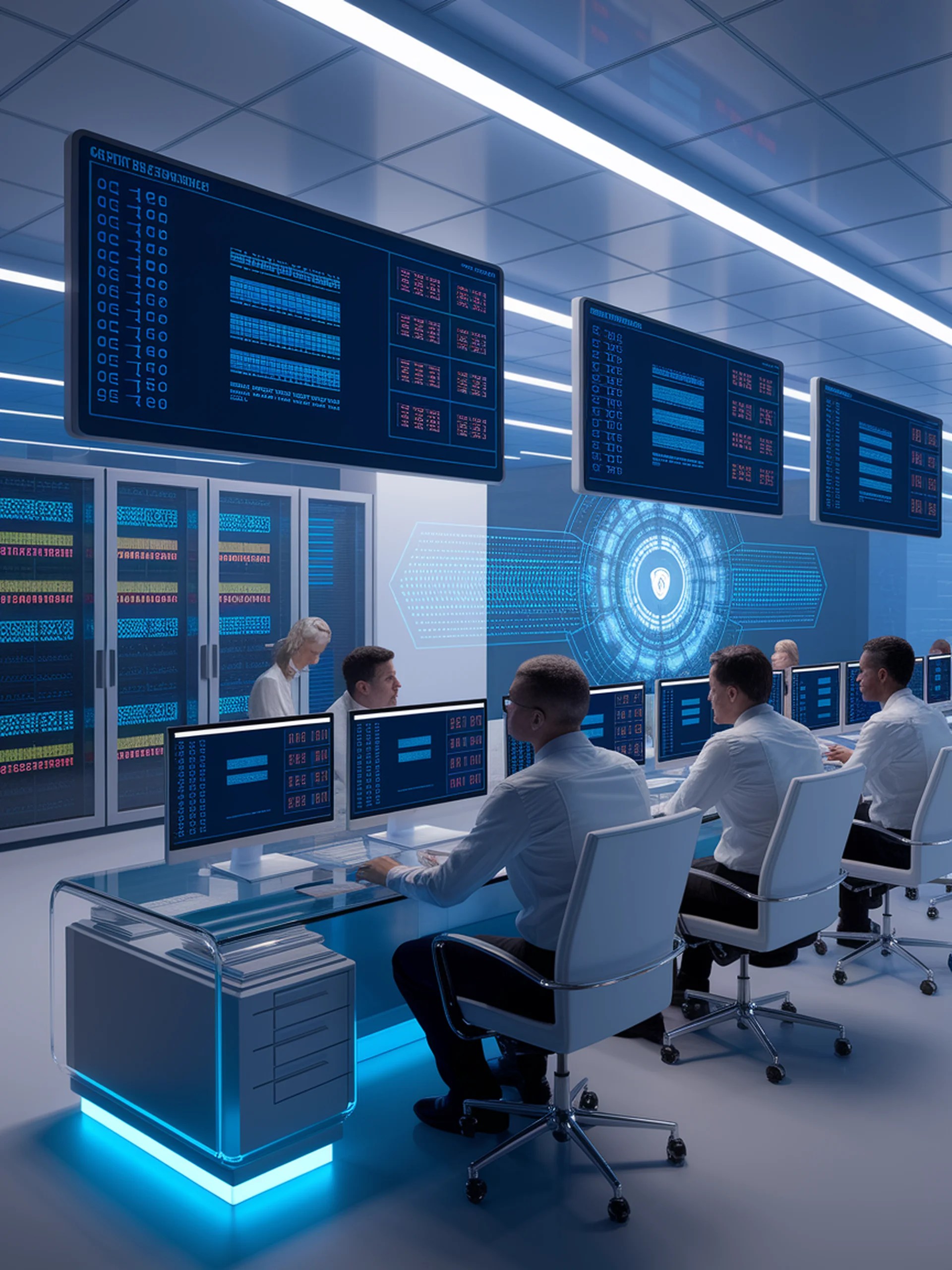
Detecting AI-Generated Content: A Robust Approach
Multi-observer methodology enhances machine text detection
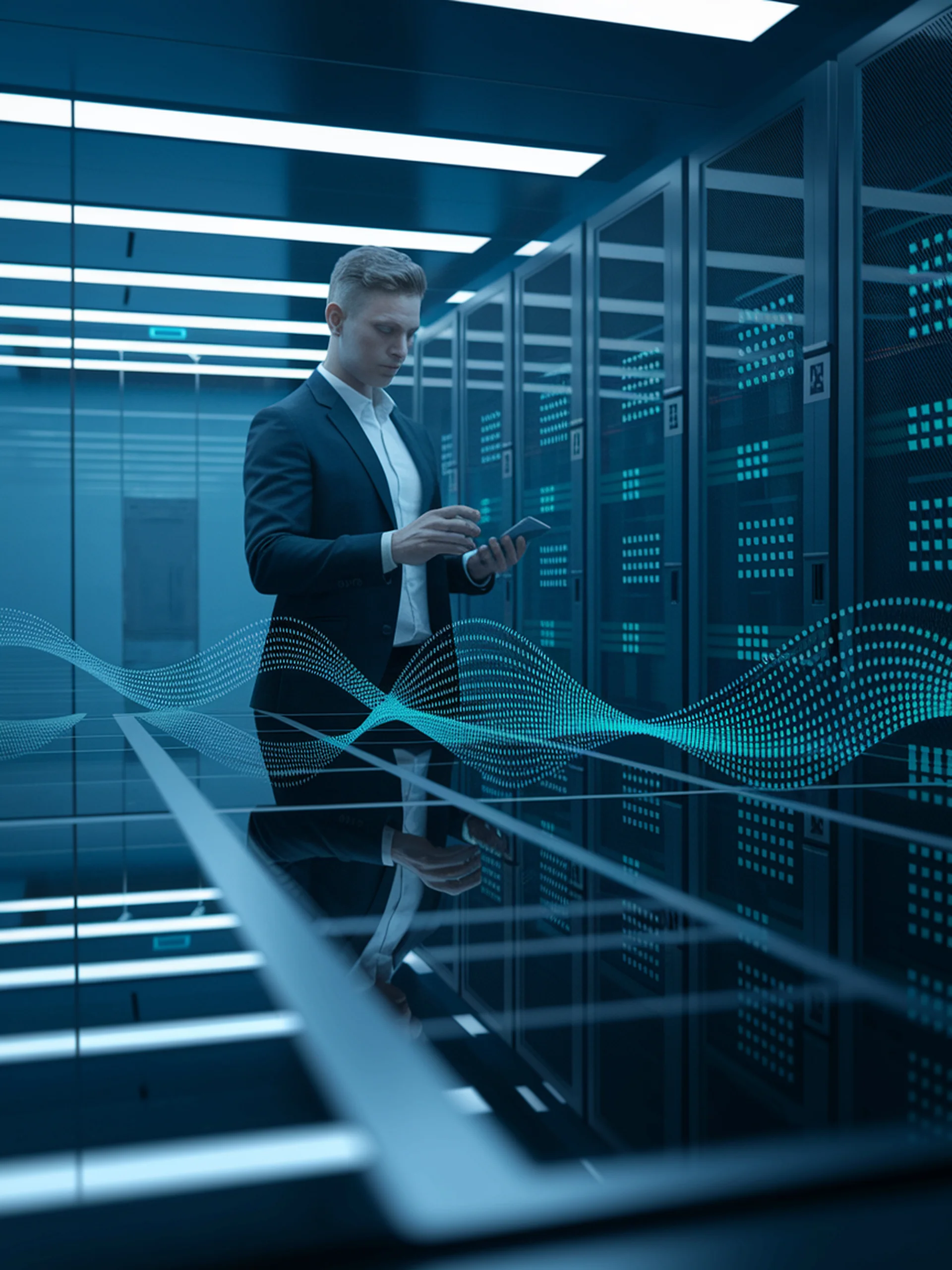
Invisible Fingerprints: Black-Box Watermarking for LLMs
Detecting AI-generated text without access to model internals
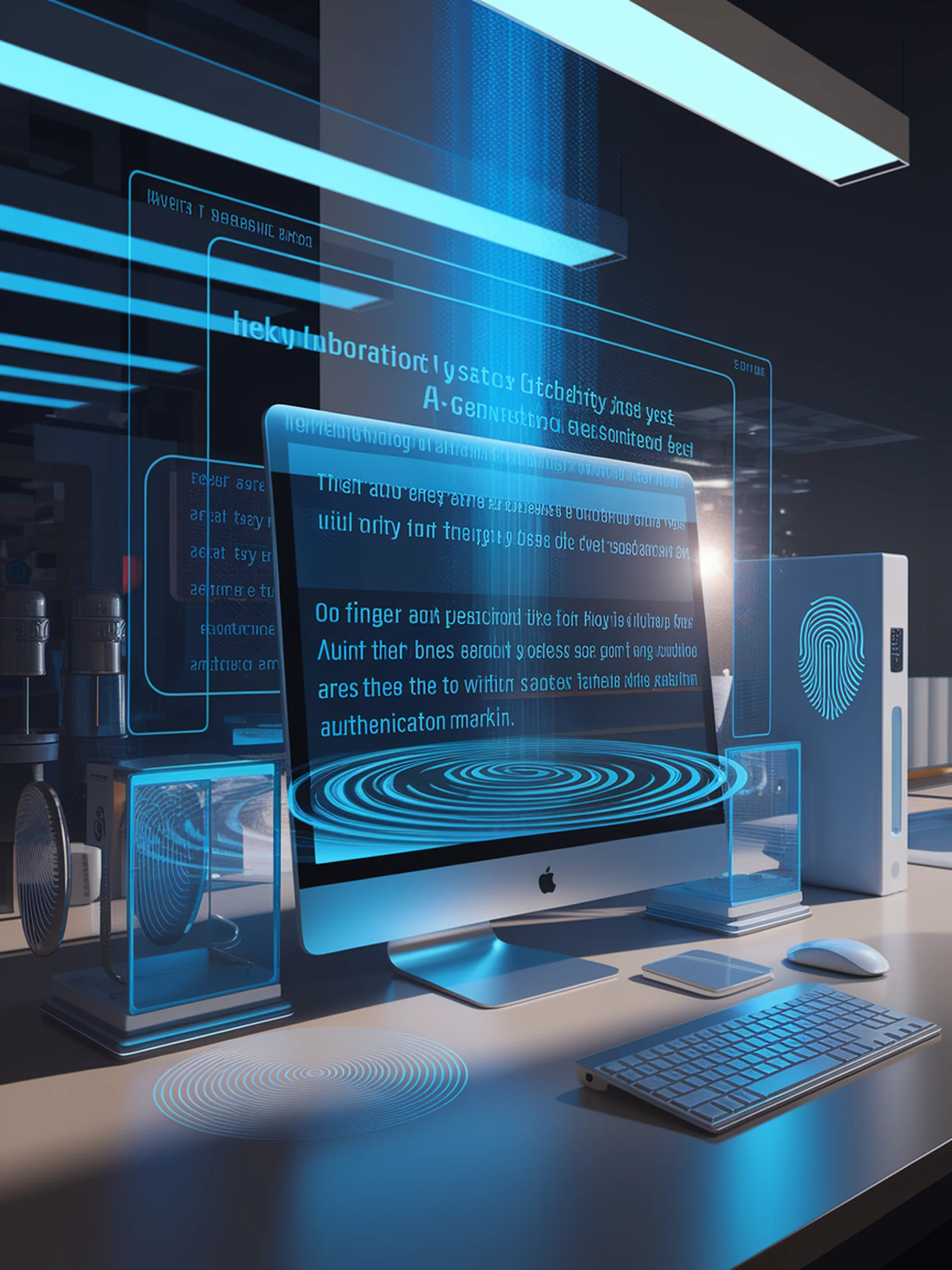
Advancing LLM Watermarking
A Distribution-Adaptive Framework for AI Text Authentication
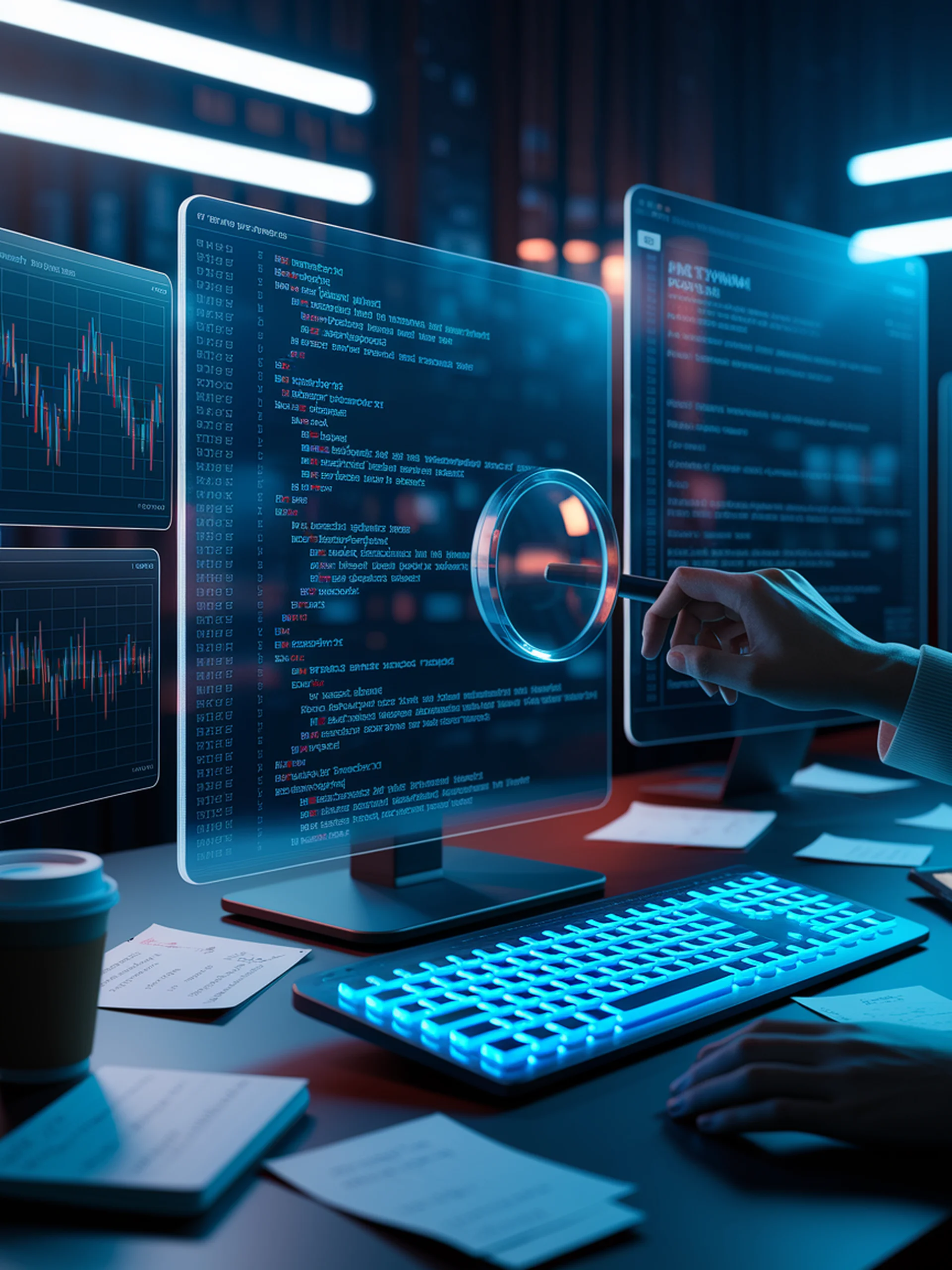
Uncovering LLM Watermarks
How users can detect hidden watermarking in AI language models
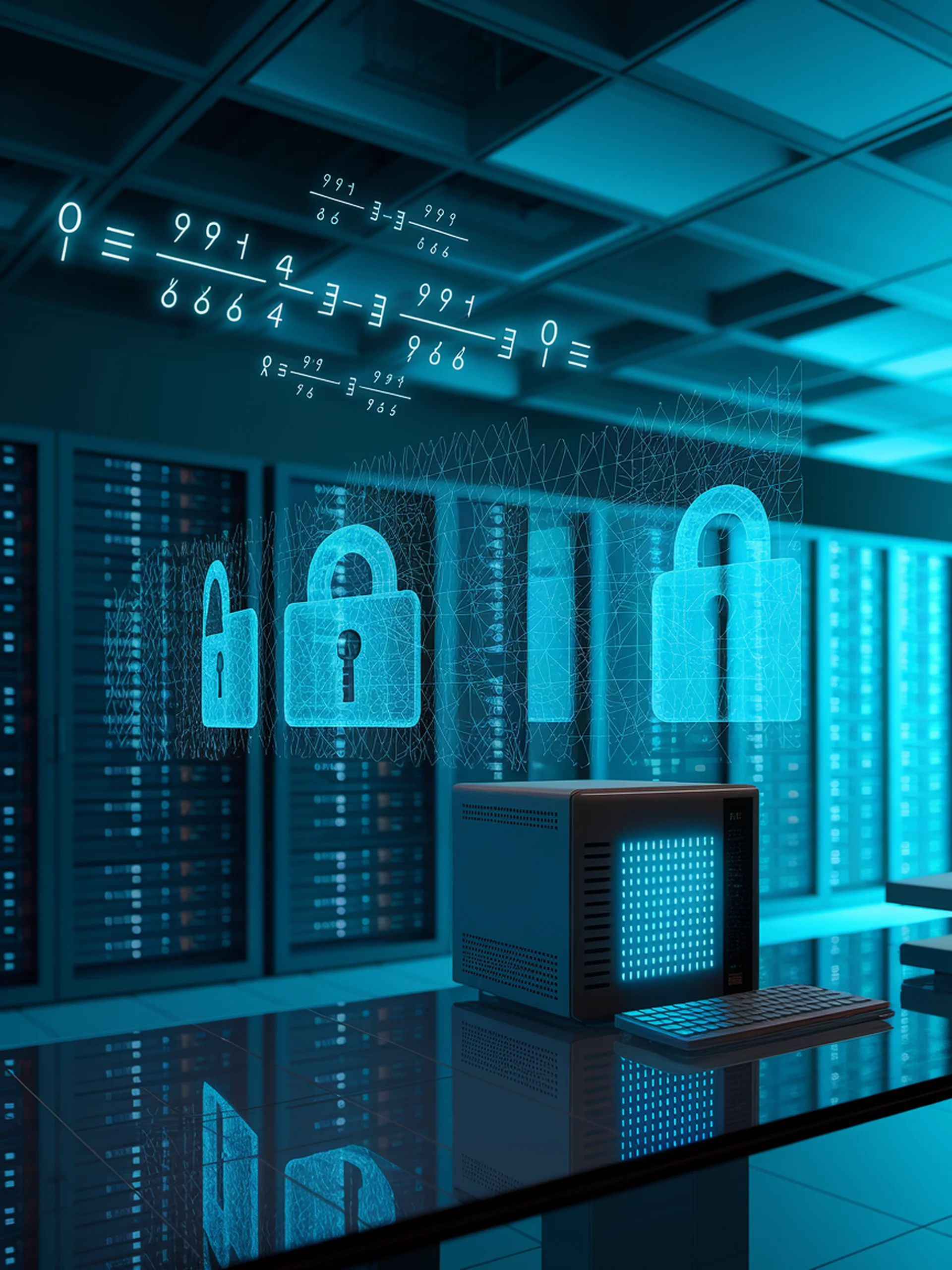
Securing LLMs with Taylor Expansion
A novel approach to protect ownership while enabling model sharing
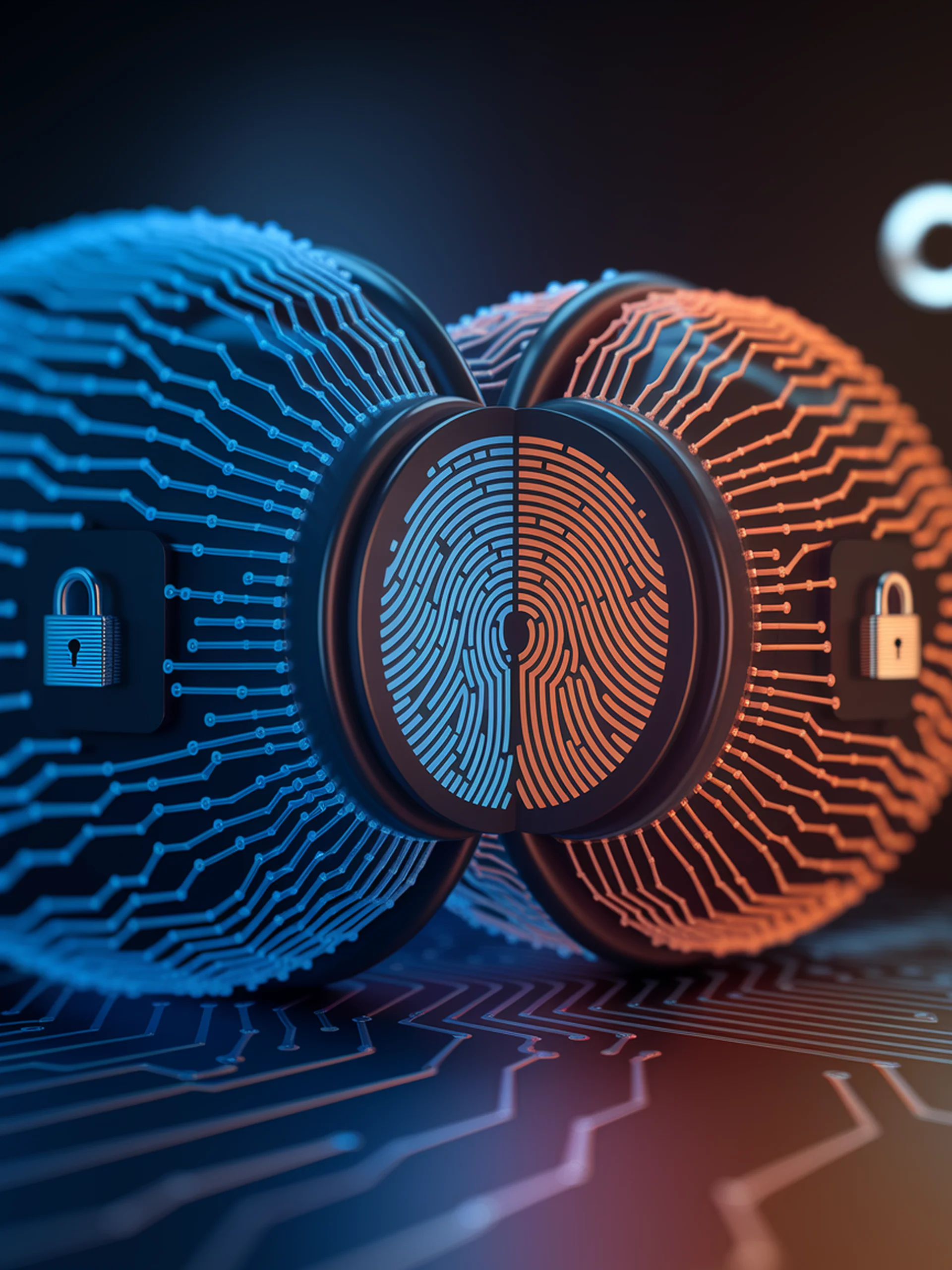
Securing LLM Ownership in the Era of Model Merging
Novel fingerprinting technique resists unauthorized model merging
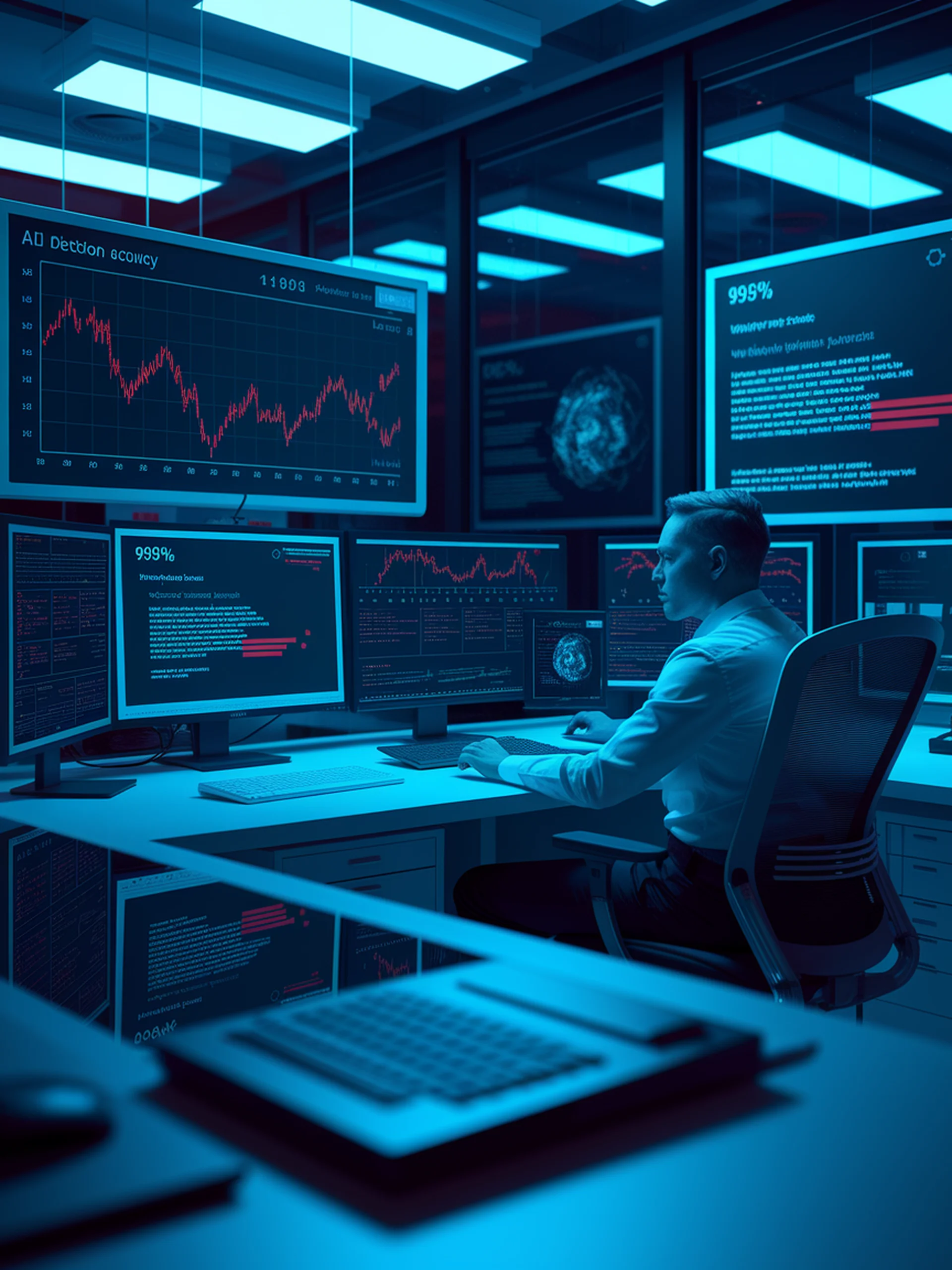
The Reality Gap in AI Detection
Why top AI detectors fail in real-world scenarios
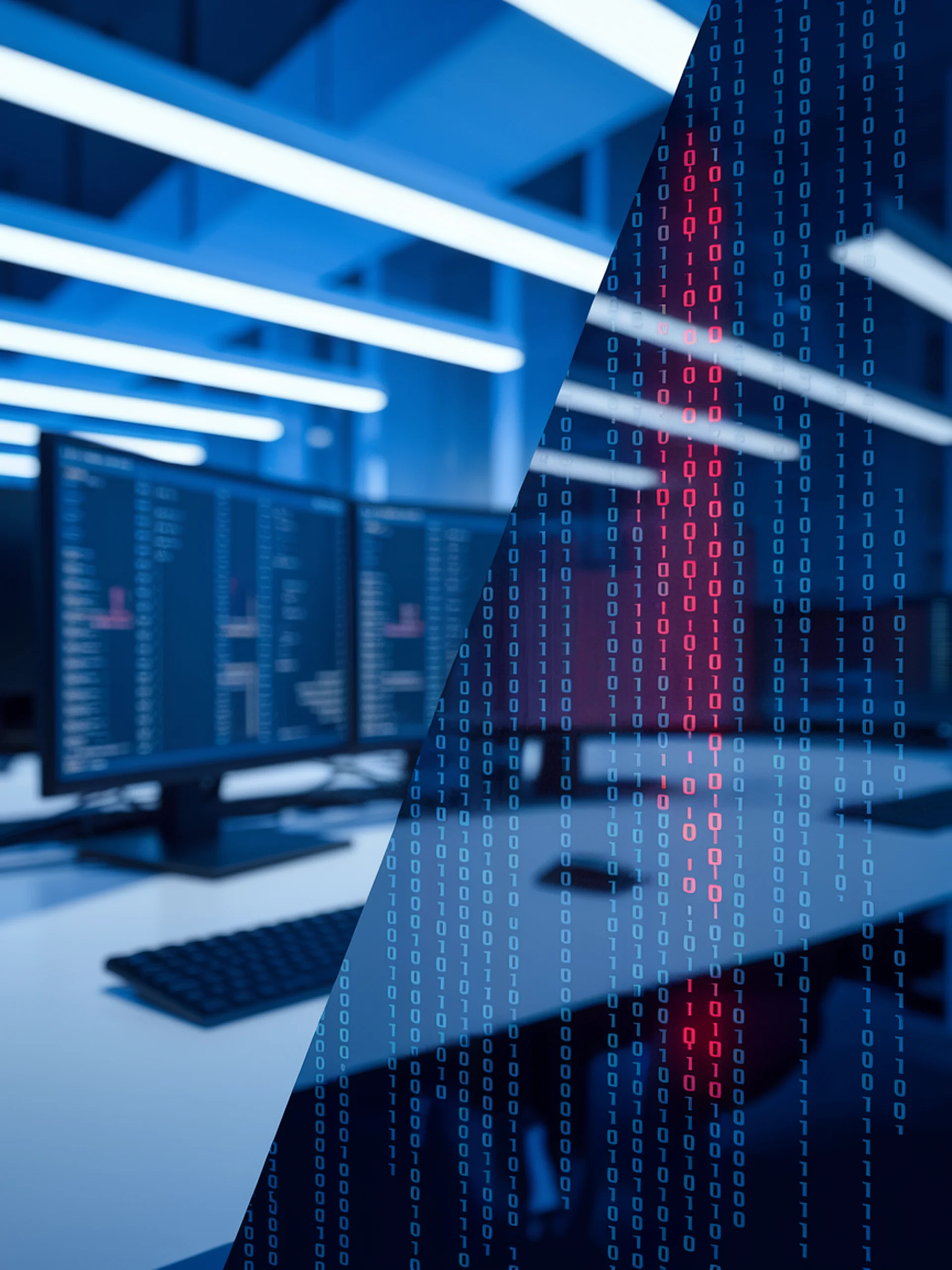
Bipolar Watermarking for LLMs
Advanced detection of AI-generated text with reduced false positives
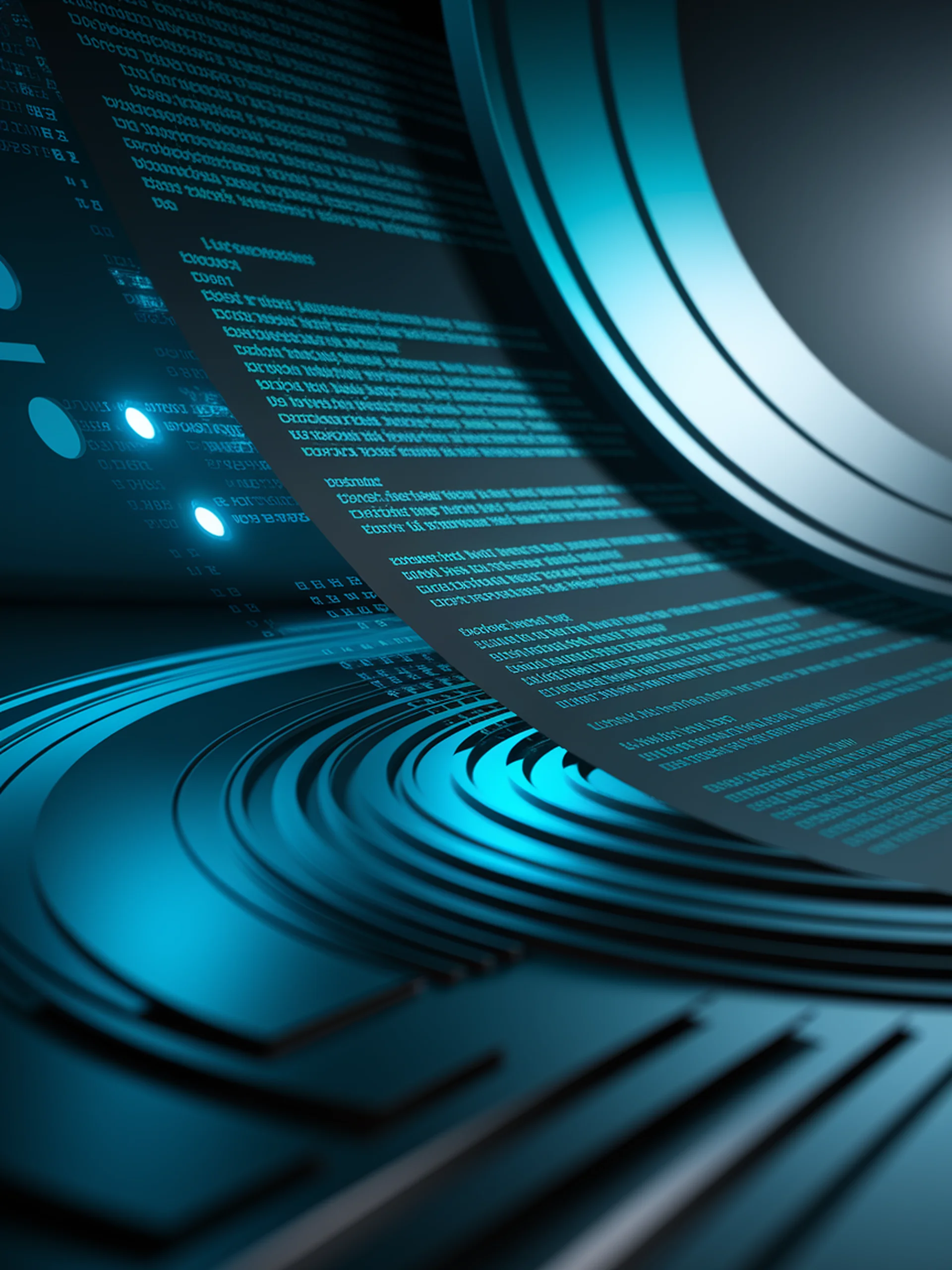
Watermarking for AI: A New Framework
Advancing Multi-bit Watermarking for Large Language Models
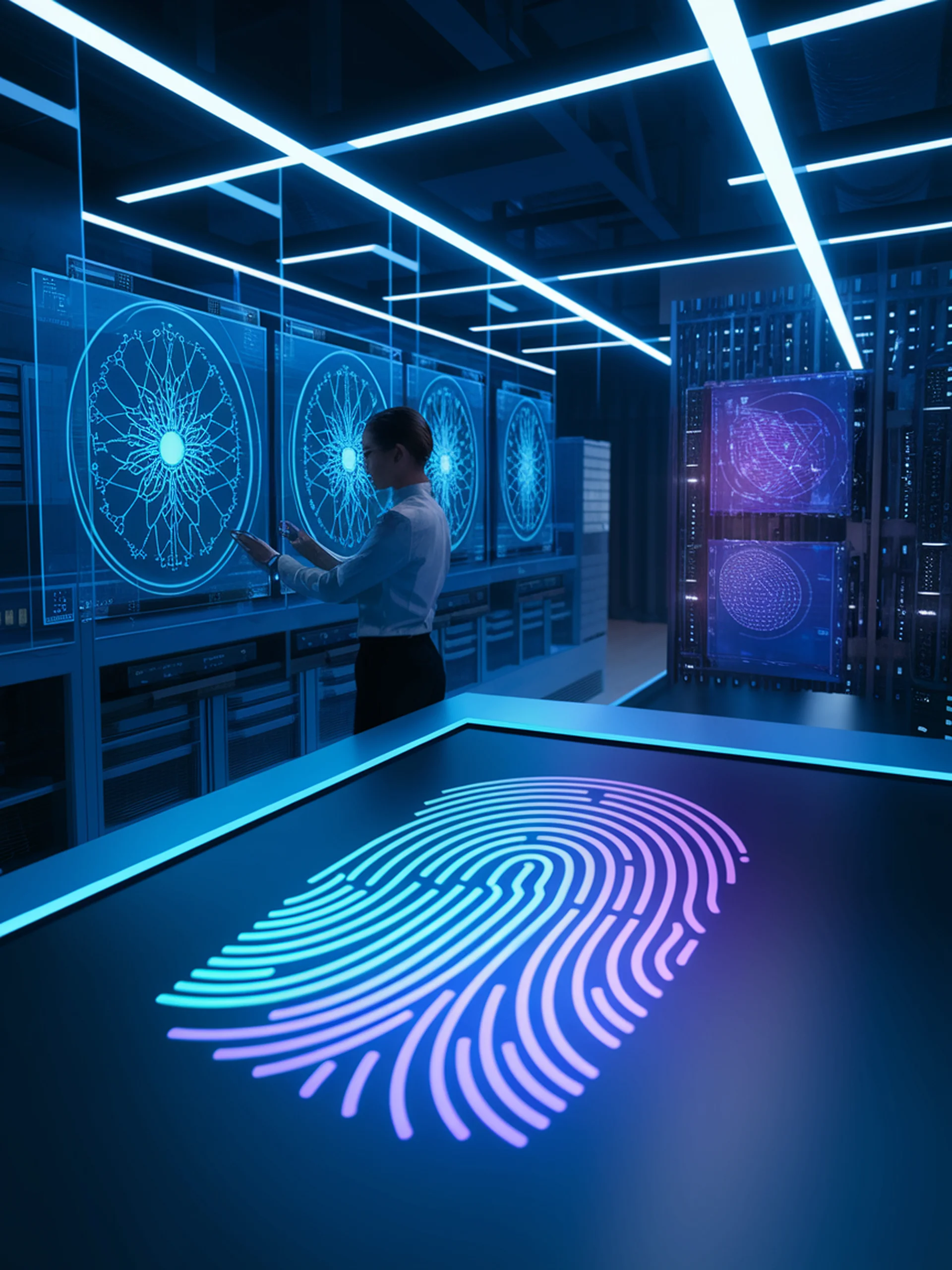
Unmasking AI Systems
New Hybrid Fingerprinting Techniques for Identifying Hidden LLMs
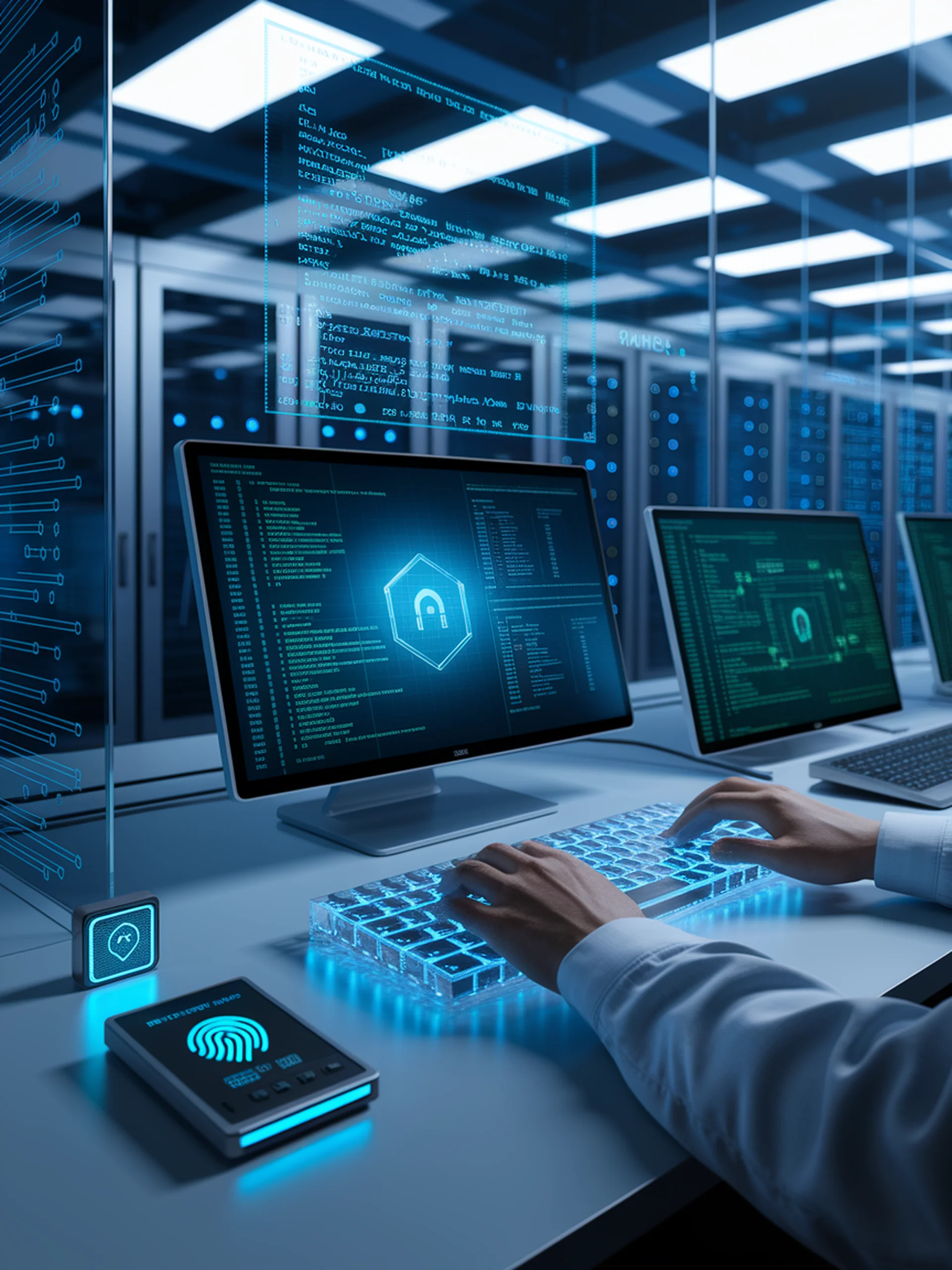
Securing AI-Generated Code
RoSeMary: A Crypto-ML Watermarking Framework for LLMs
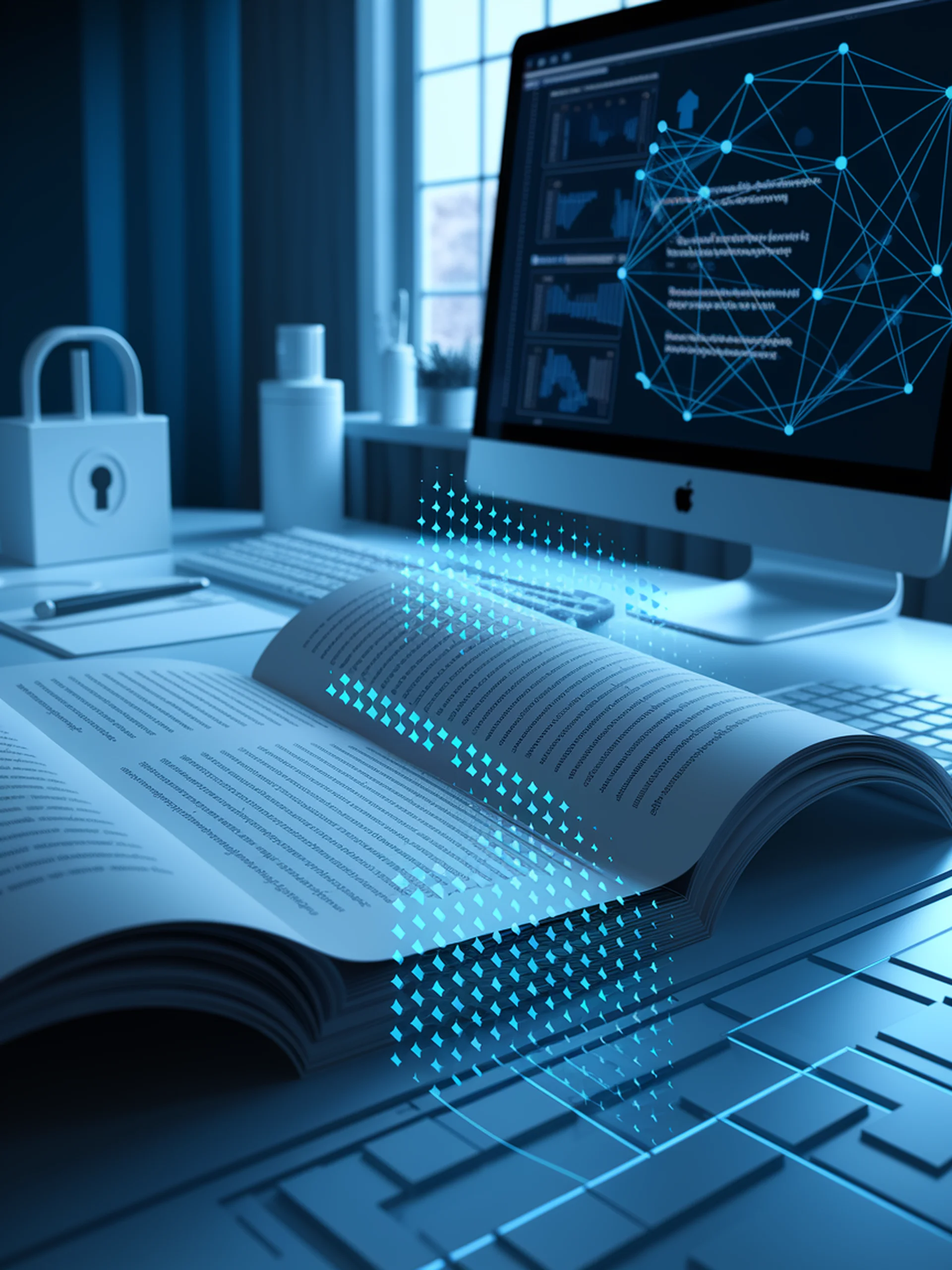
Watermarking LLM Outputs
A robust method to trace AI-generated content without model access
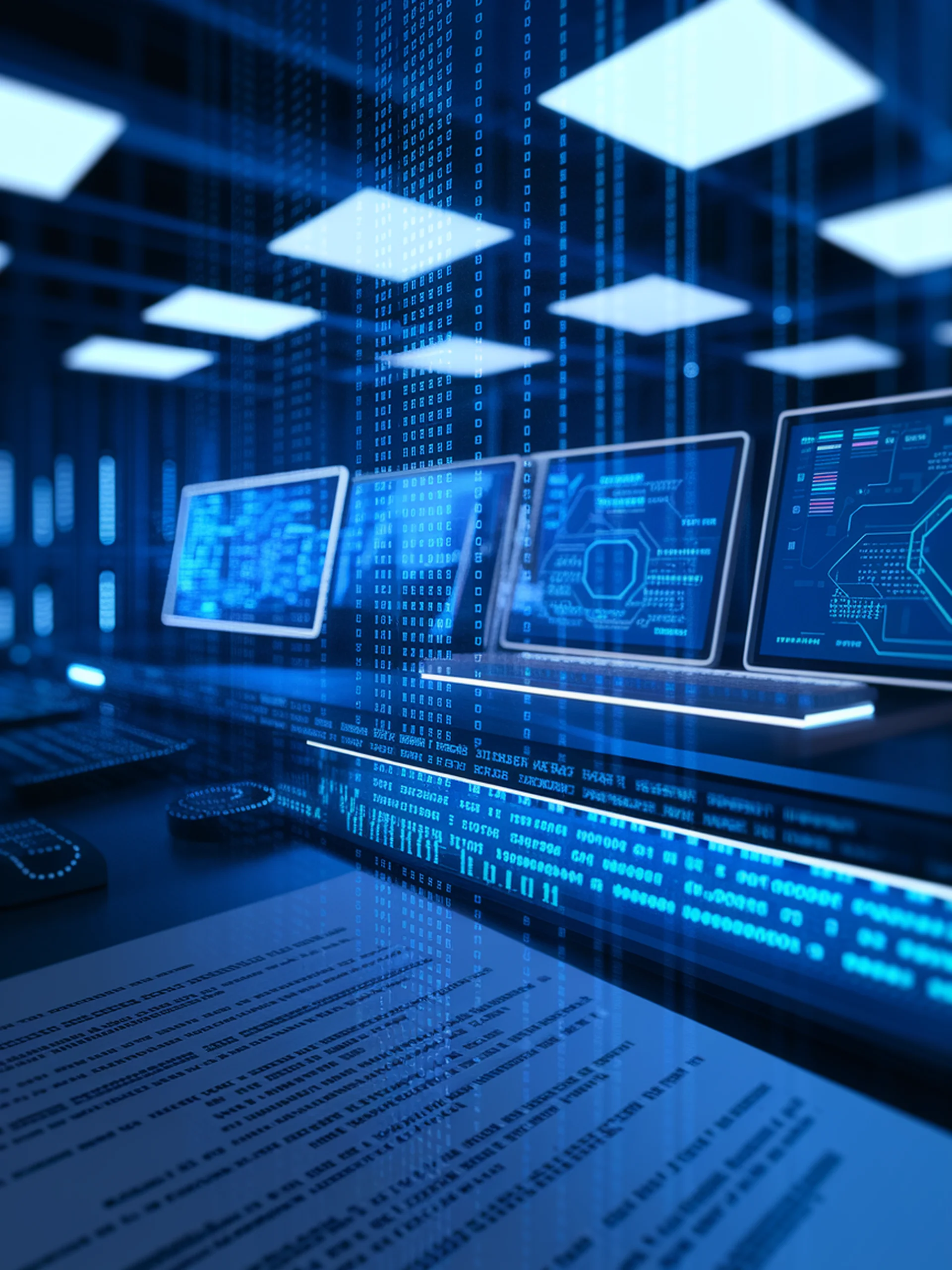
Securing AI: Robust Watermarking for LLMs
A dynamic multi-bit watermarking technique to protect AI-generated content
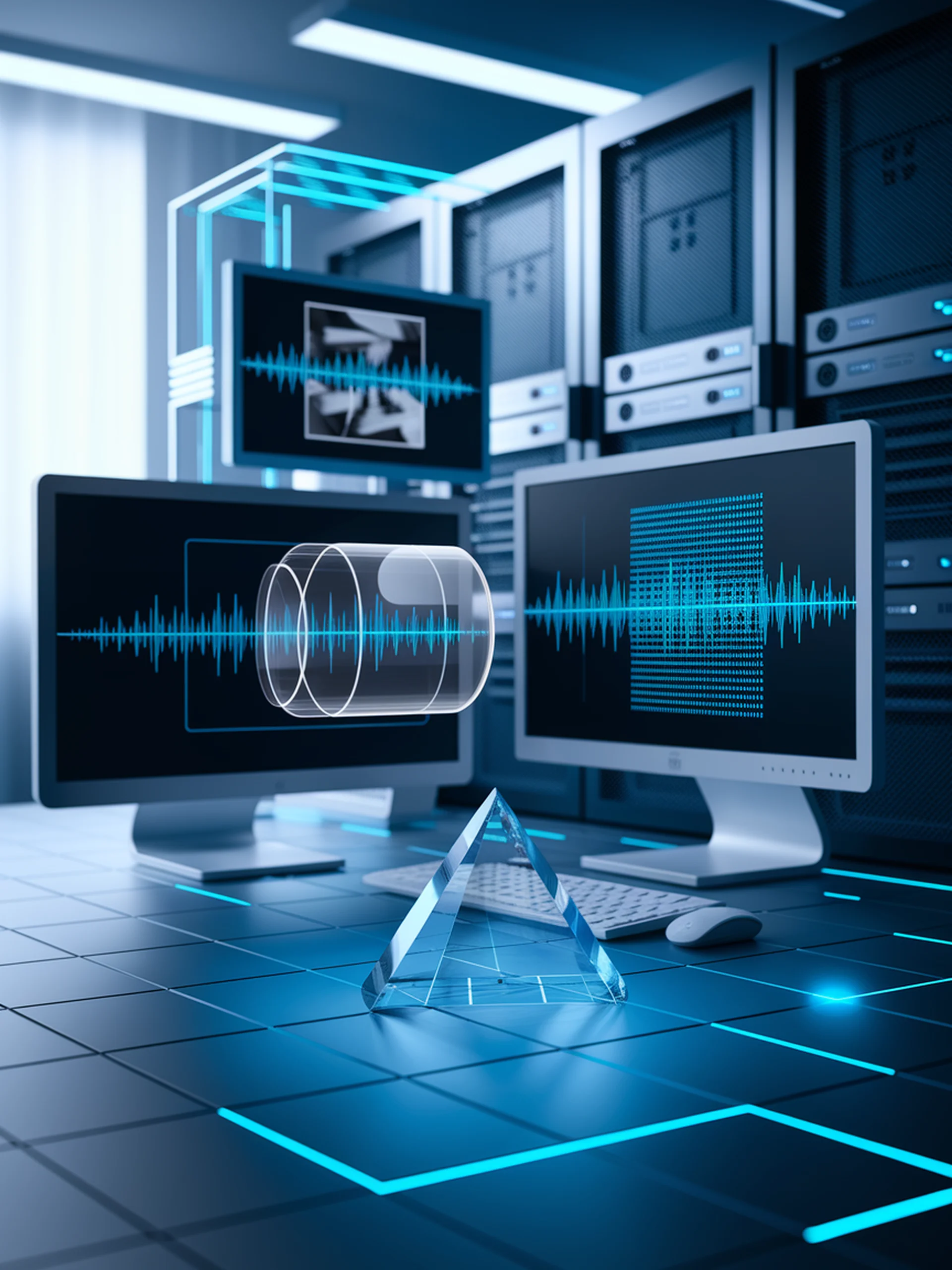
Invisible Guardians: AI Content Watermarking
Novel watermarking techniques across images, audio, and text
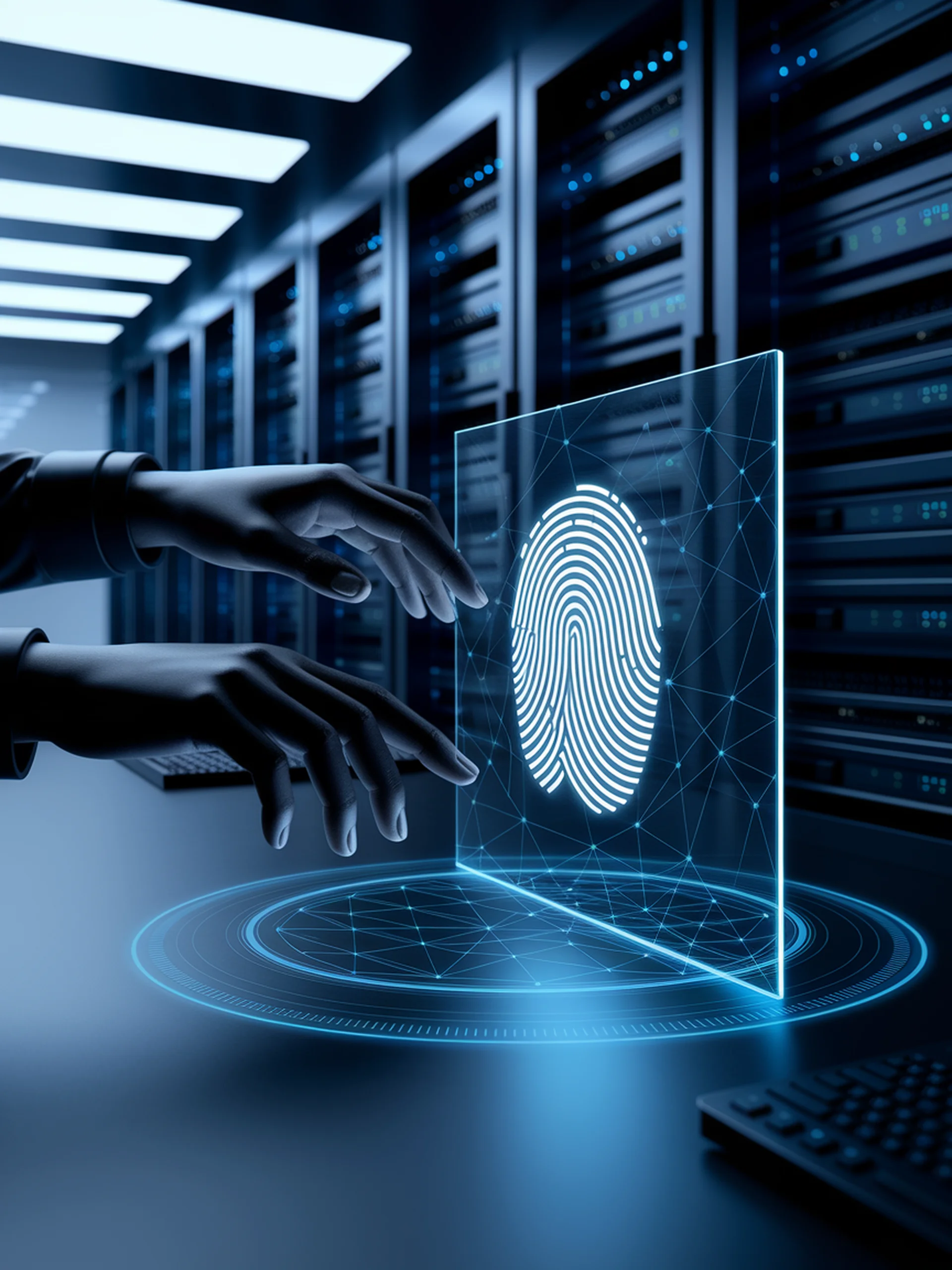
Securing AI Assets with Scalable Fingerprints
Advanced techniques to protect LLM ownership at scale
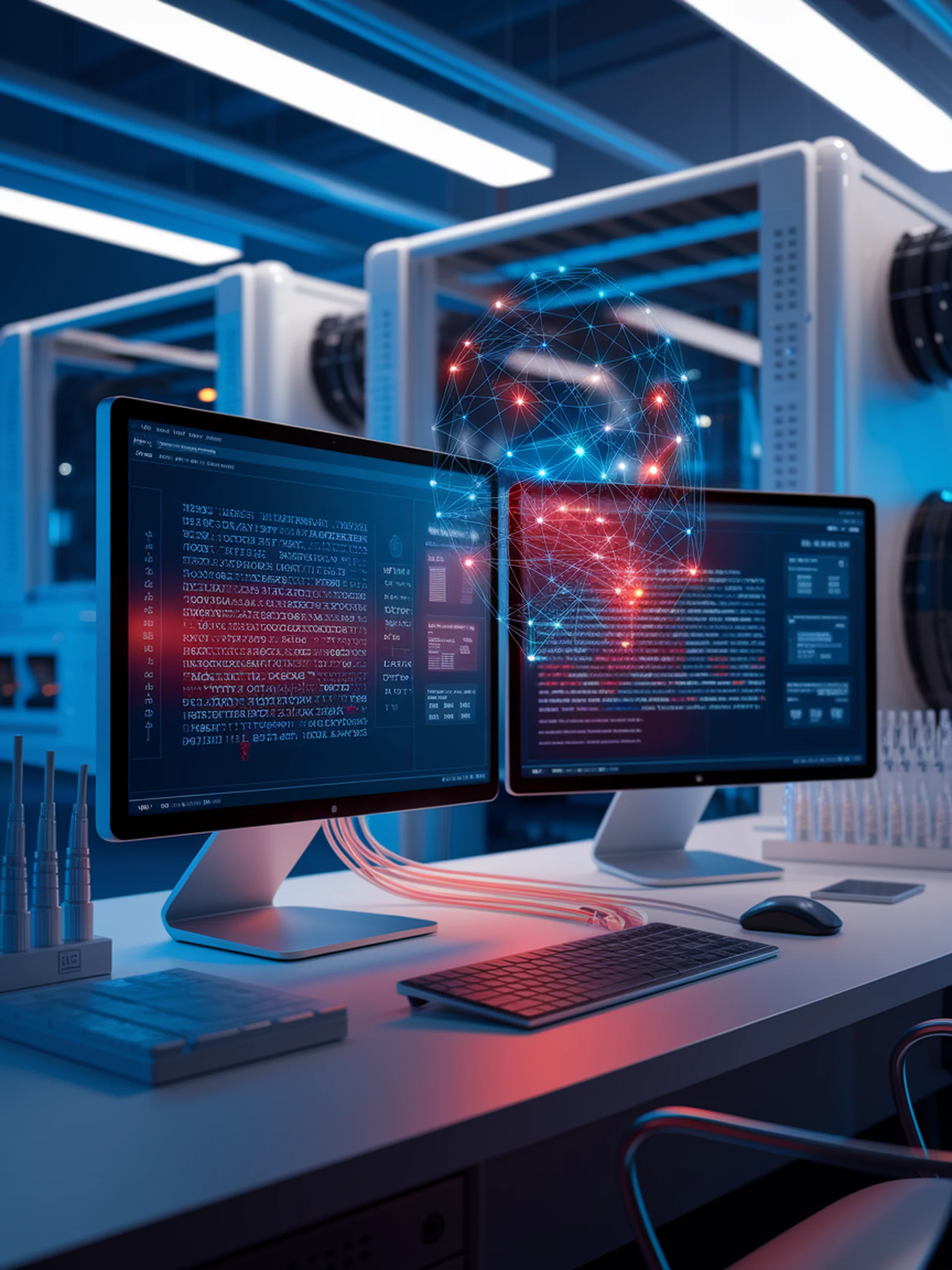
Dual-Detection Watermarking for LLM Outputs
Protecting AI-generated content from modification attacks and detecting spoofing
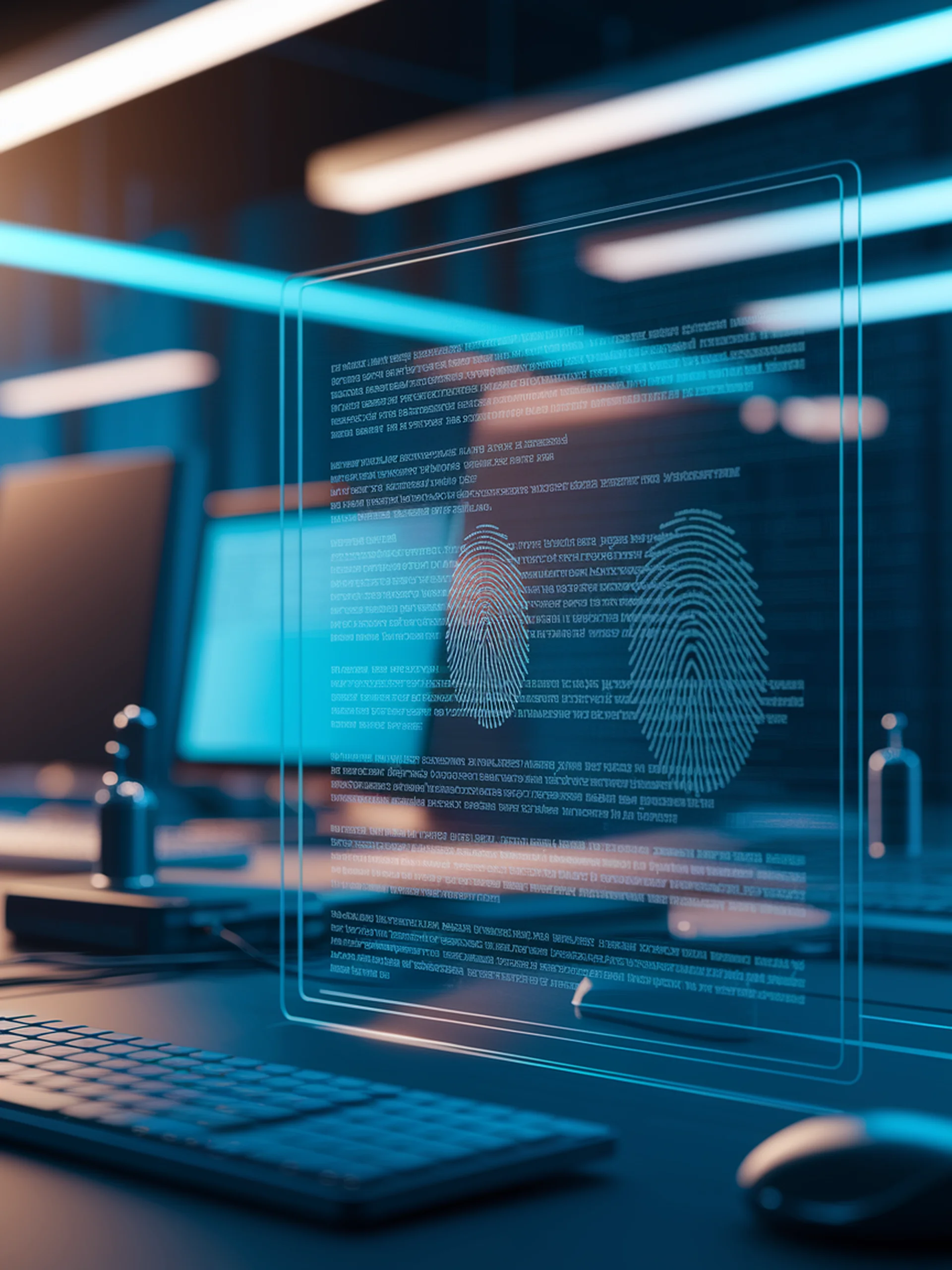
Invisible Fingerprints: Next-Gen AI Watermarking
Enhancing content authentication without sacrificing quality
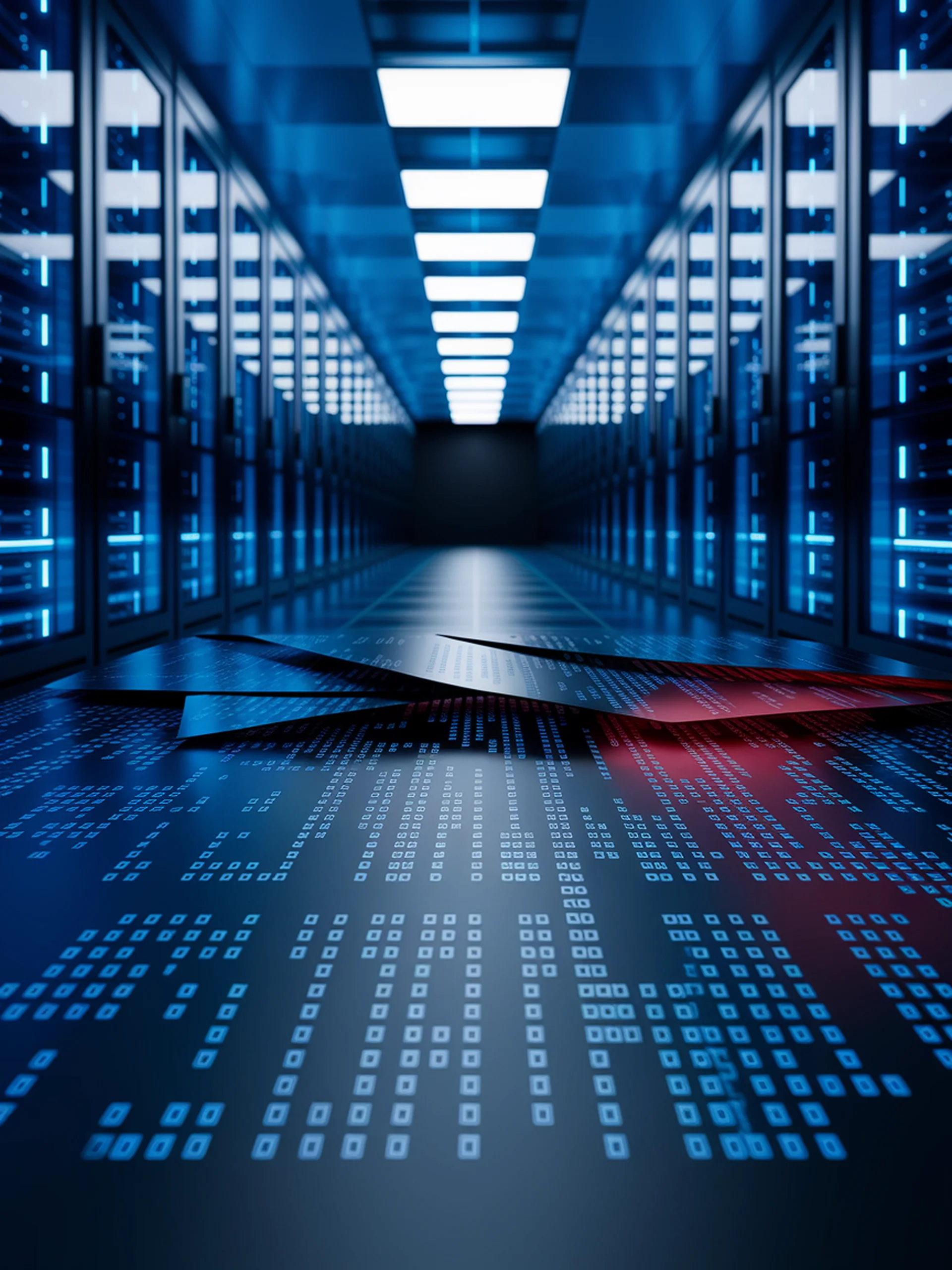
Breaking the Watermark Shield
How attackers can evade LLM watermarking protections
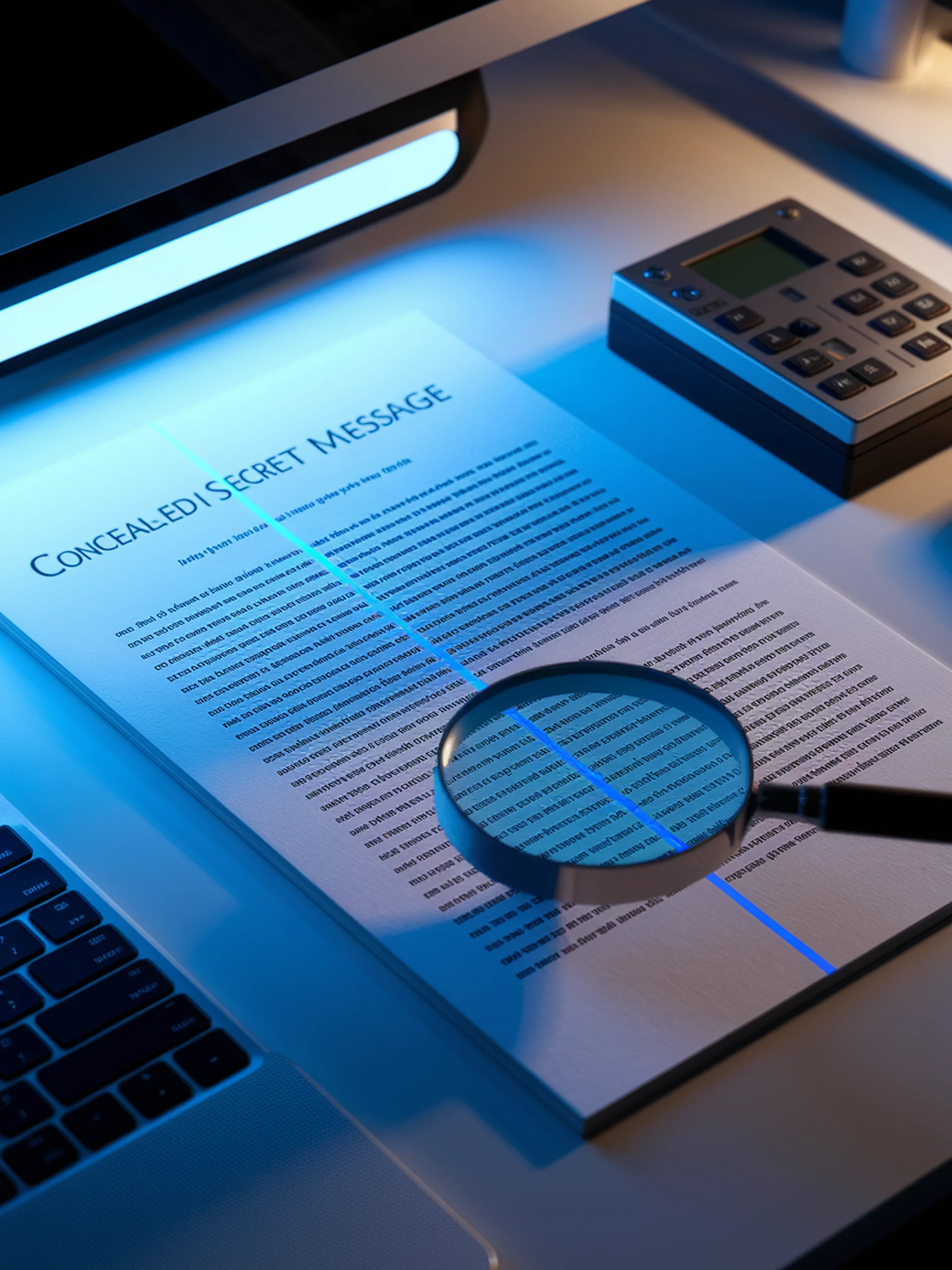
Hiding in Plain Text
A Novel Whitespace-Based Steganography Method for Text Authentication
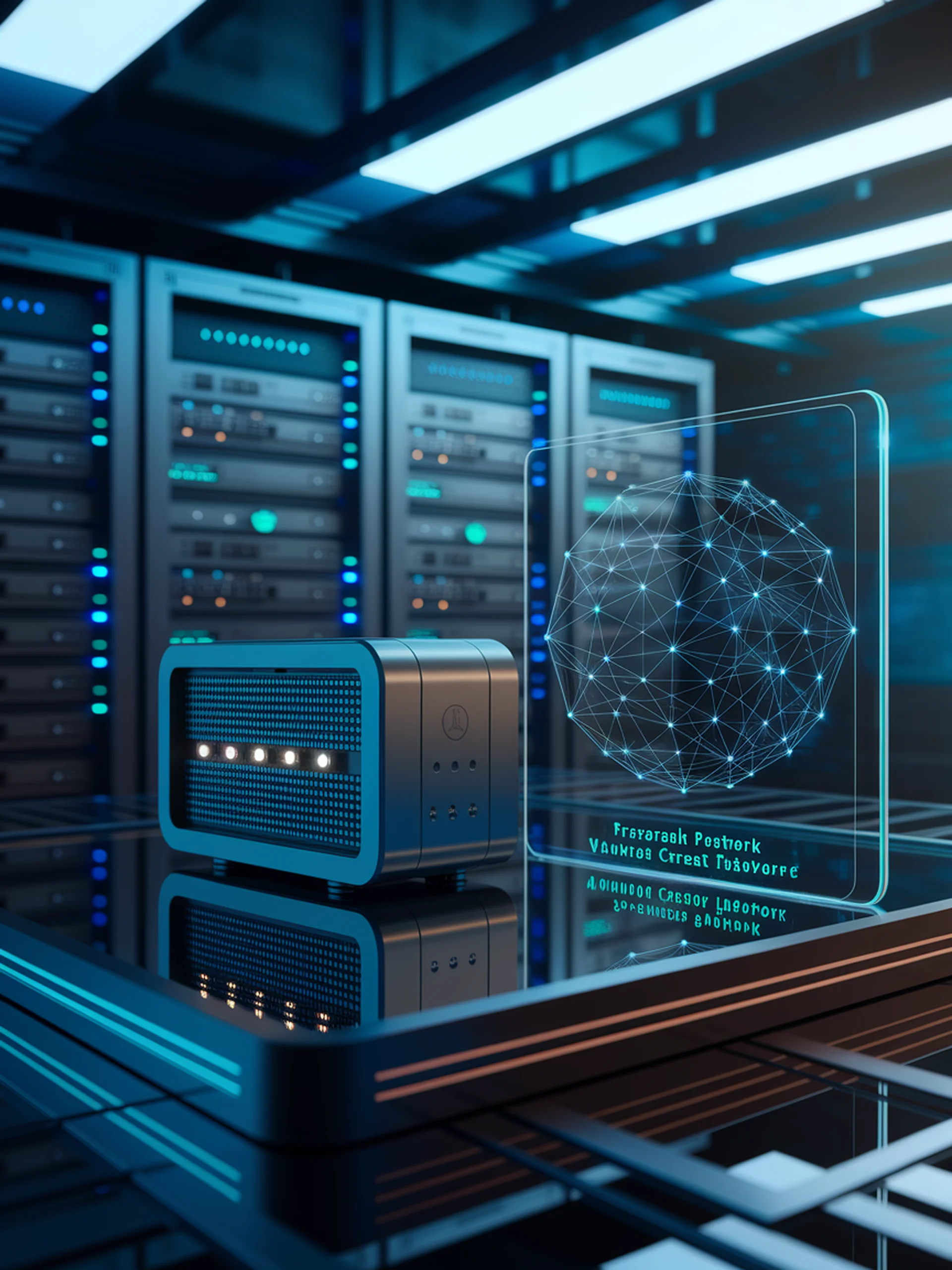
Protecting AI Vision Models: Digital Copyright
Novel approach to track unauthorized usage of vision-language models
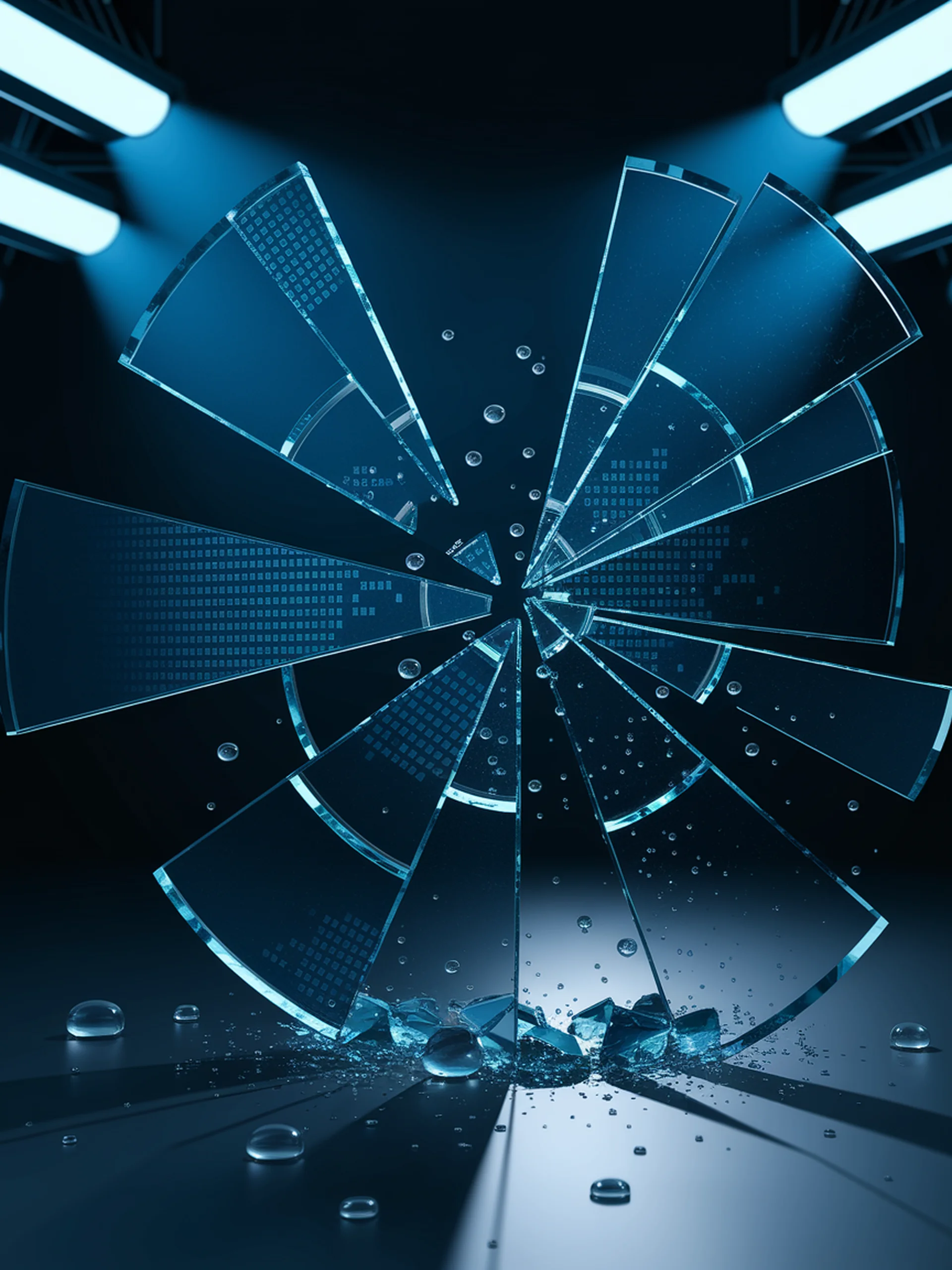
Breaking the Watermark Barrier
Vulnerabilities in LLM Watermarking Security Systems
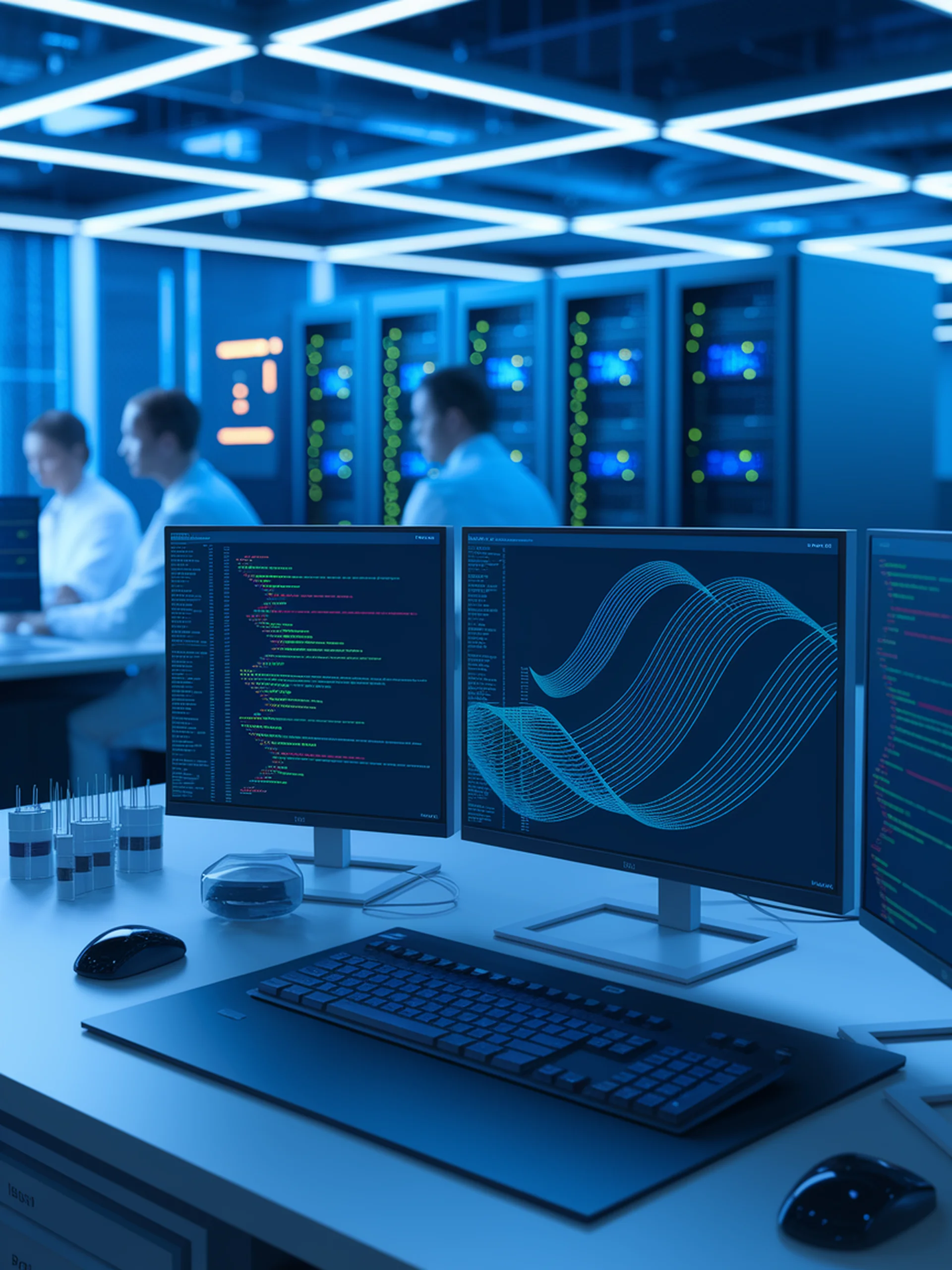
Protecting Open-Source LLMs from Misuse
Novel Watermarking Techniques for LLM Security
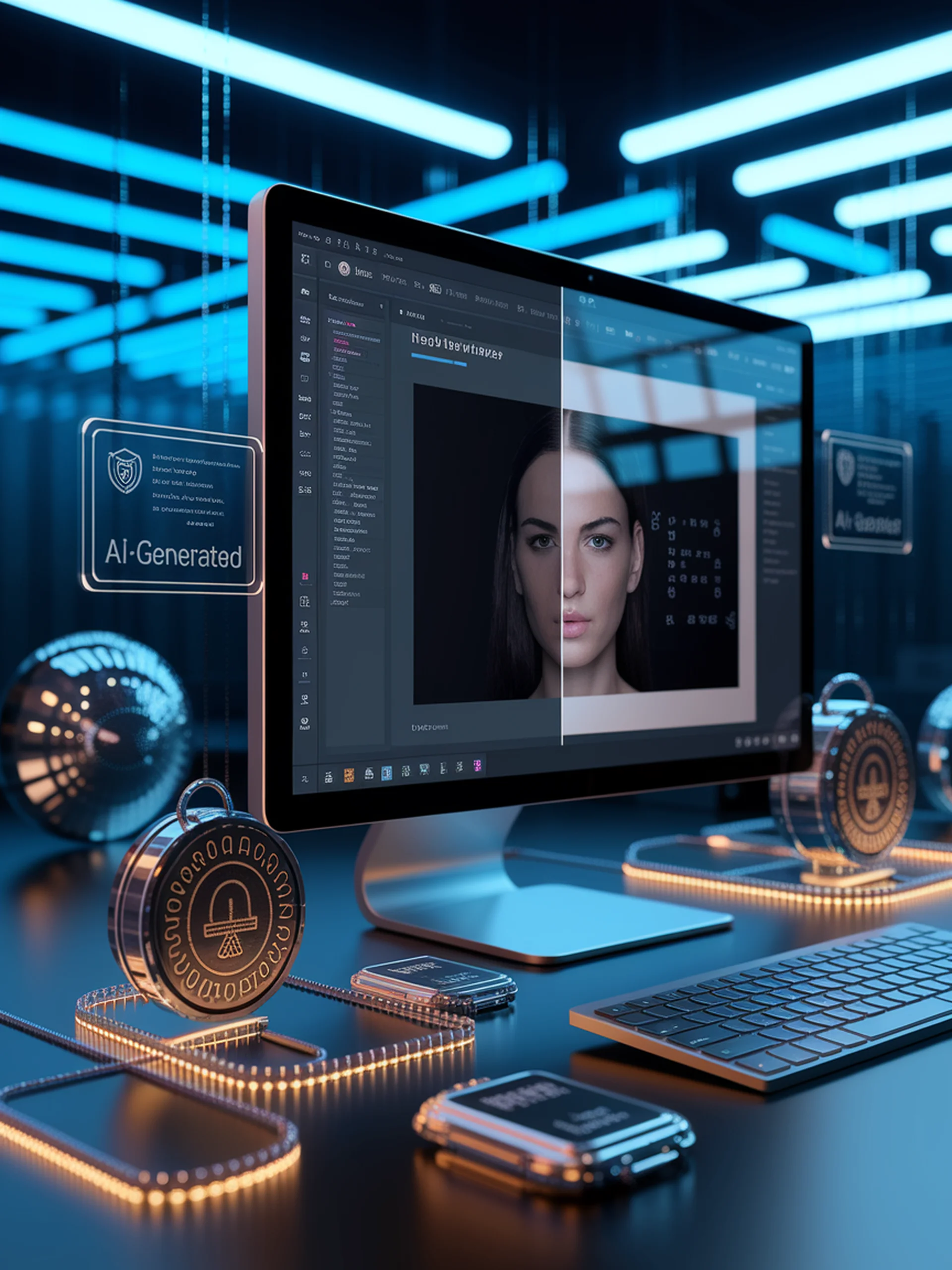
Securing AI-Generated Images
First Watermarking Solution for Visual Autoregressive Models
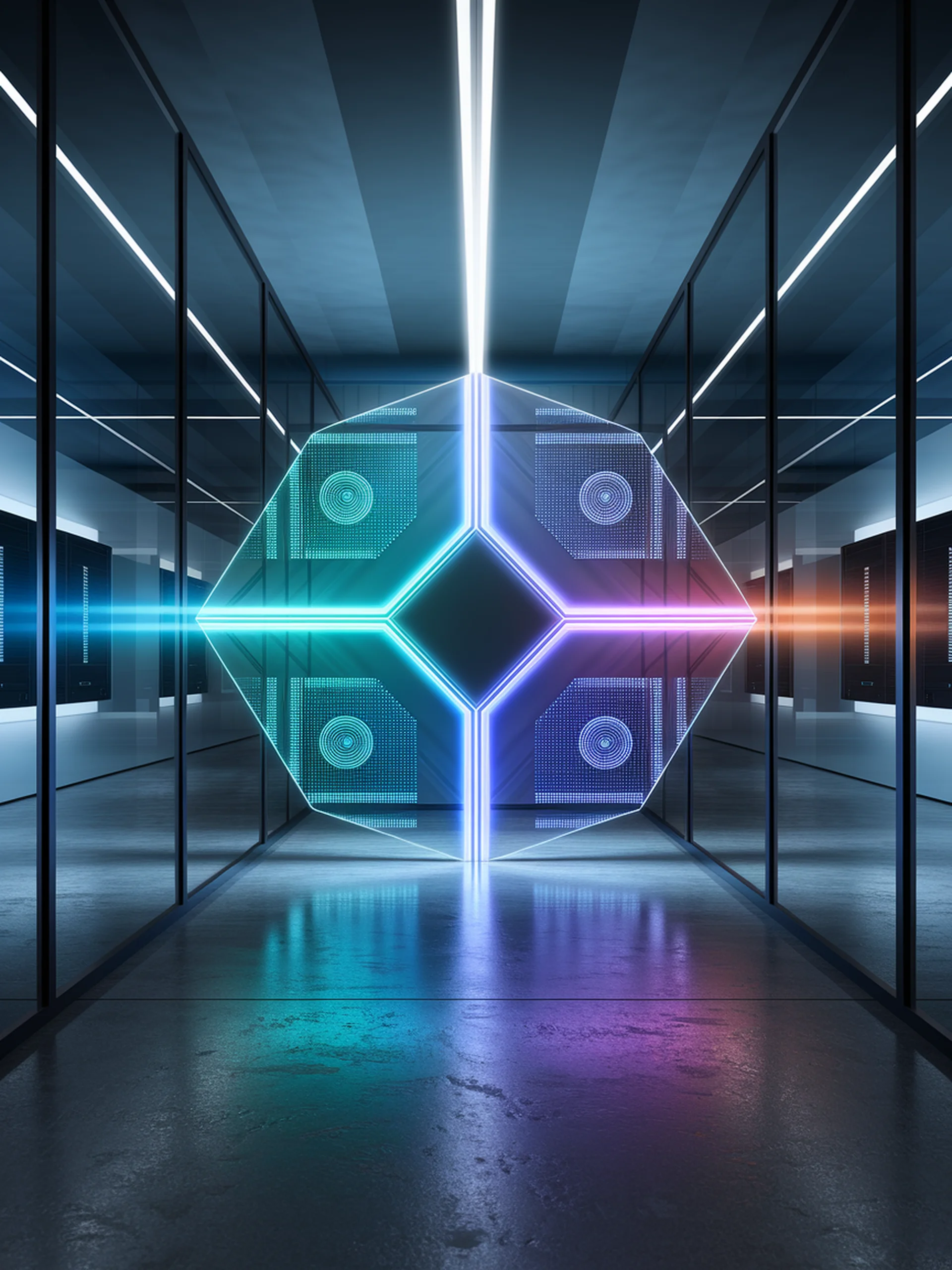
A Complete Framework for LLM Watermarking
Evaluating text watermarks across five critical dimensions
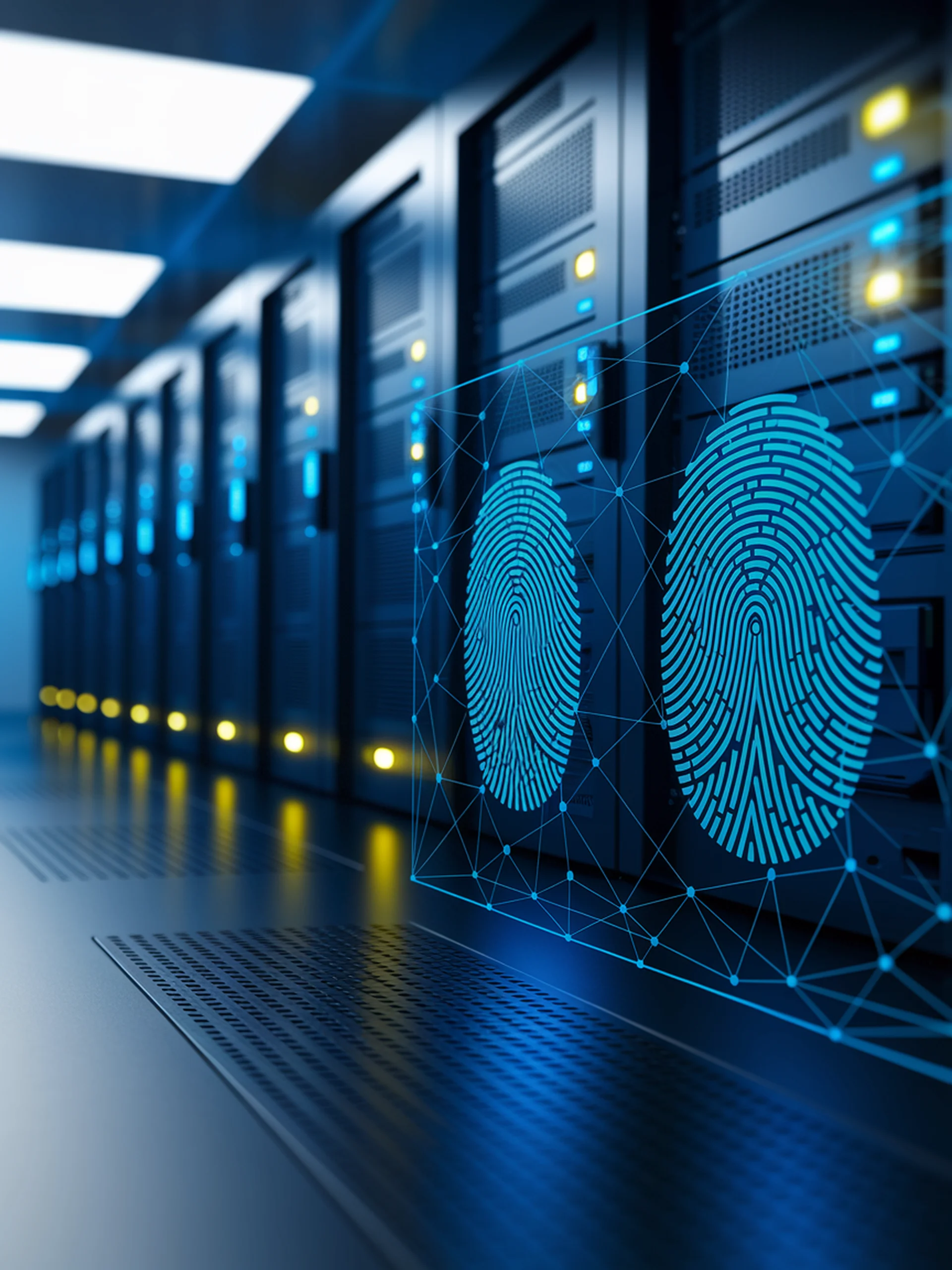
Securing LLM Intellectual Property
Introducing ImF: A Robust Fingerprinting Technique for Model Ownership Protection
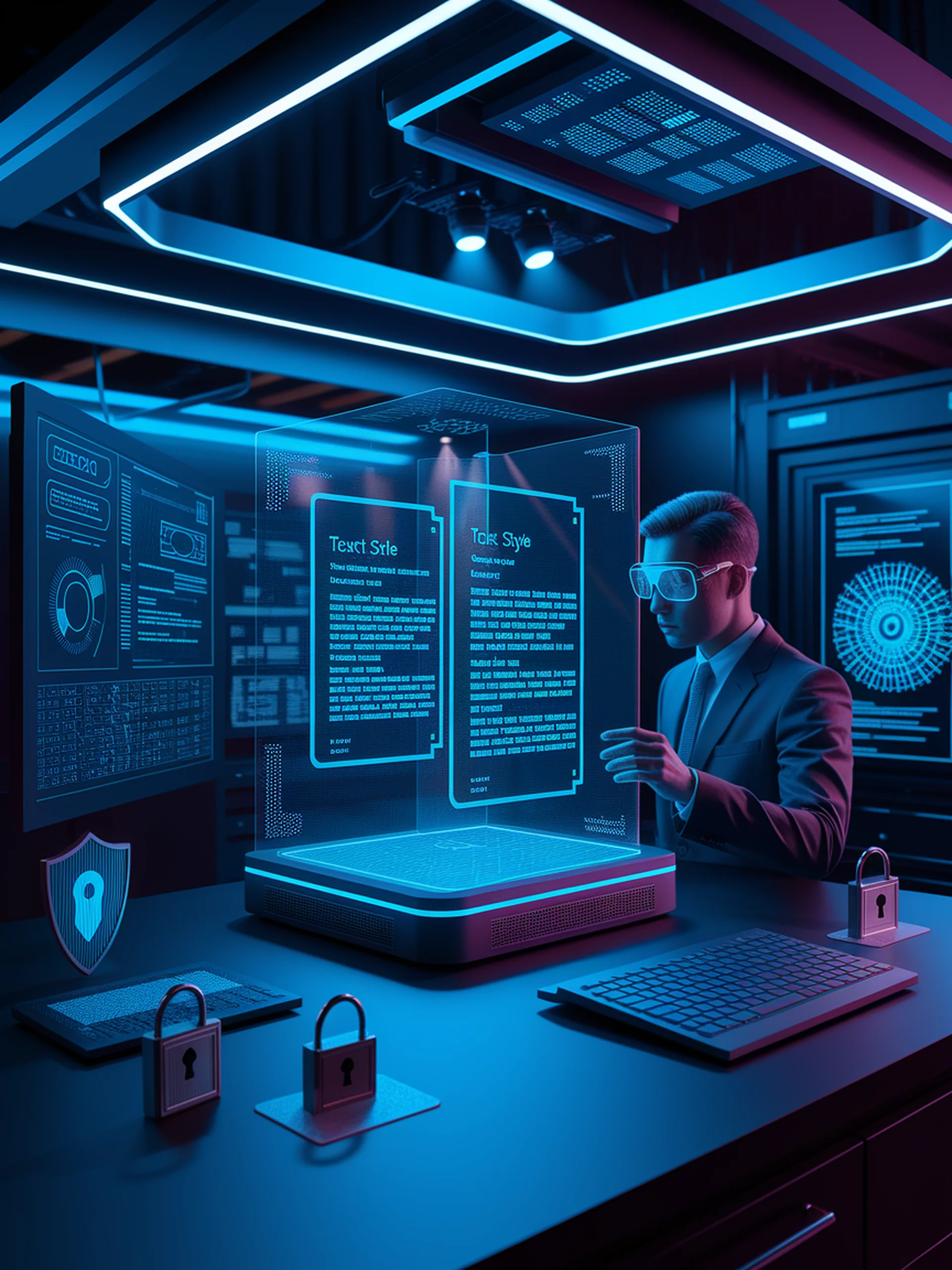
Protecting Text Style Copyright
Introducing MiZero: An Implicit Zero-Watermarking Solution
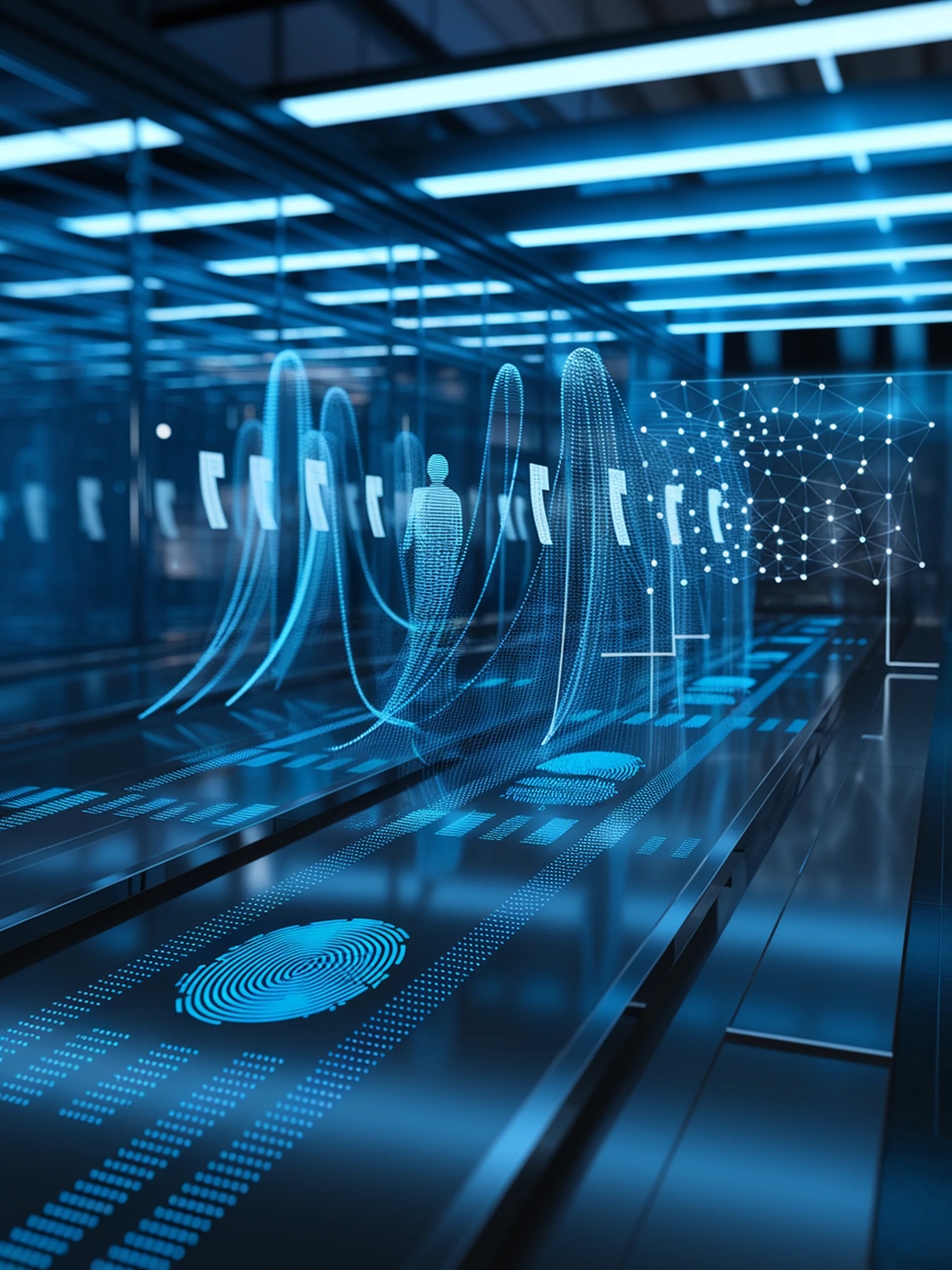
Watermarking for AI Agents
A Novel Framework for Tracing and Securing Intelligent Agents
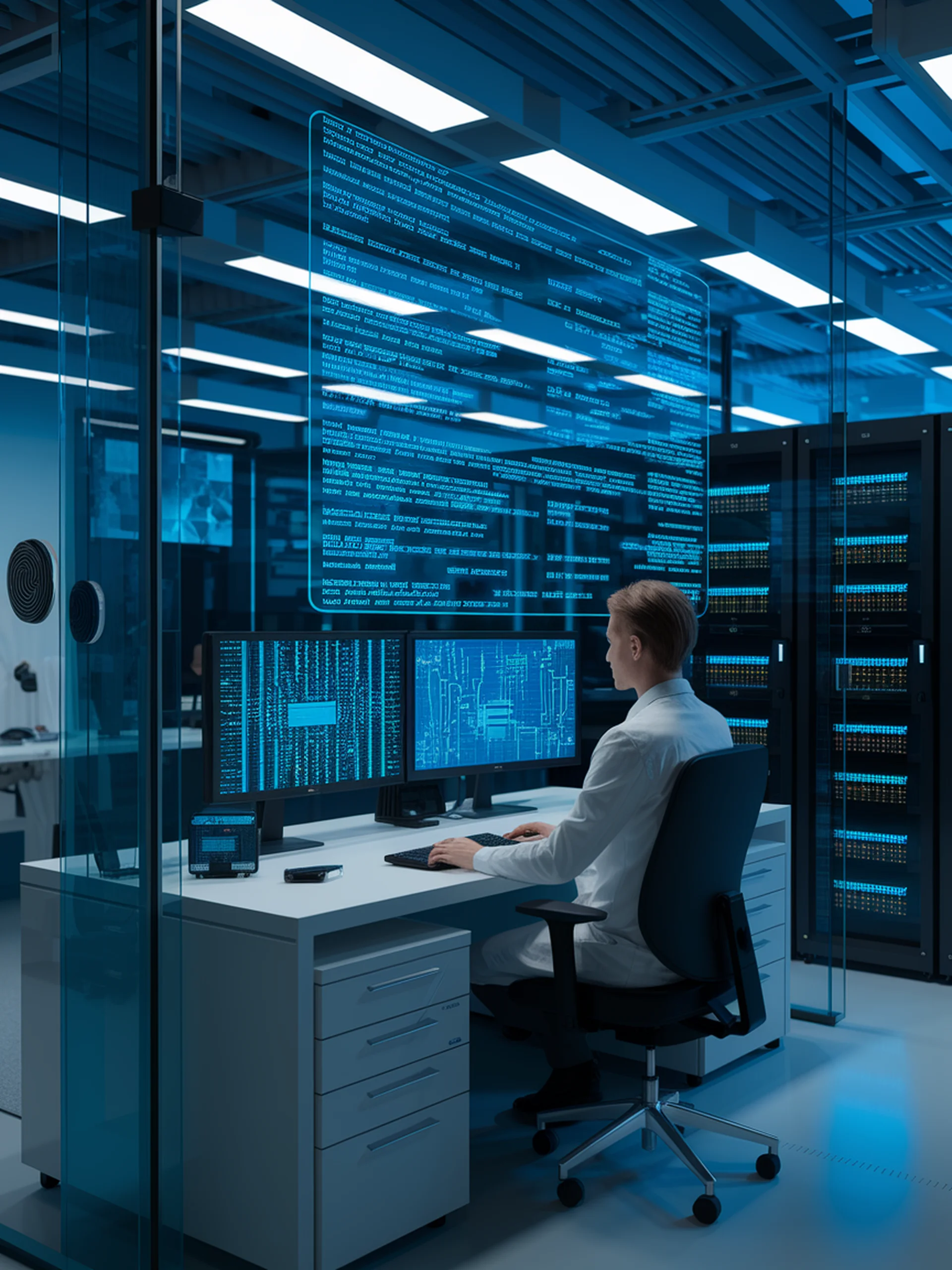
Embedded Watermarks for LLMs
Finetuning models to secretly mark AI-generated content
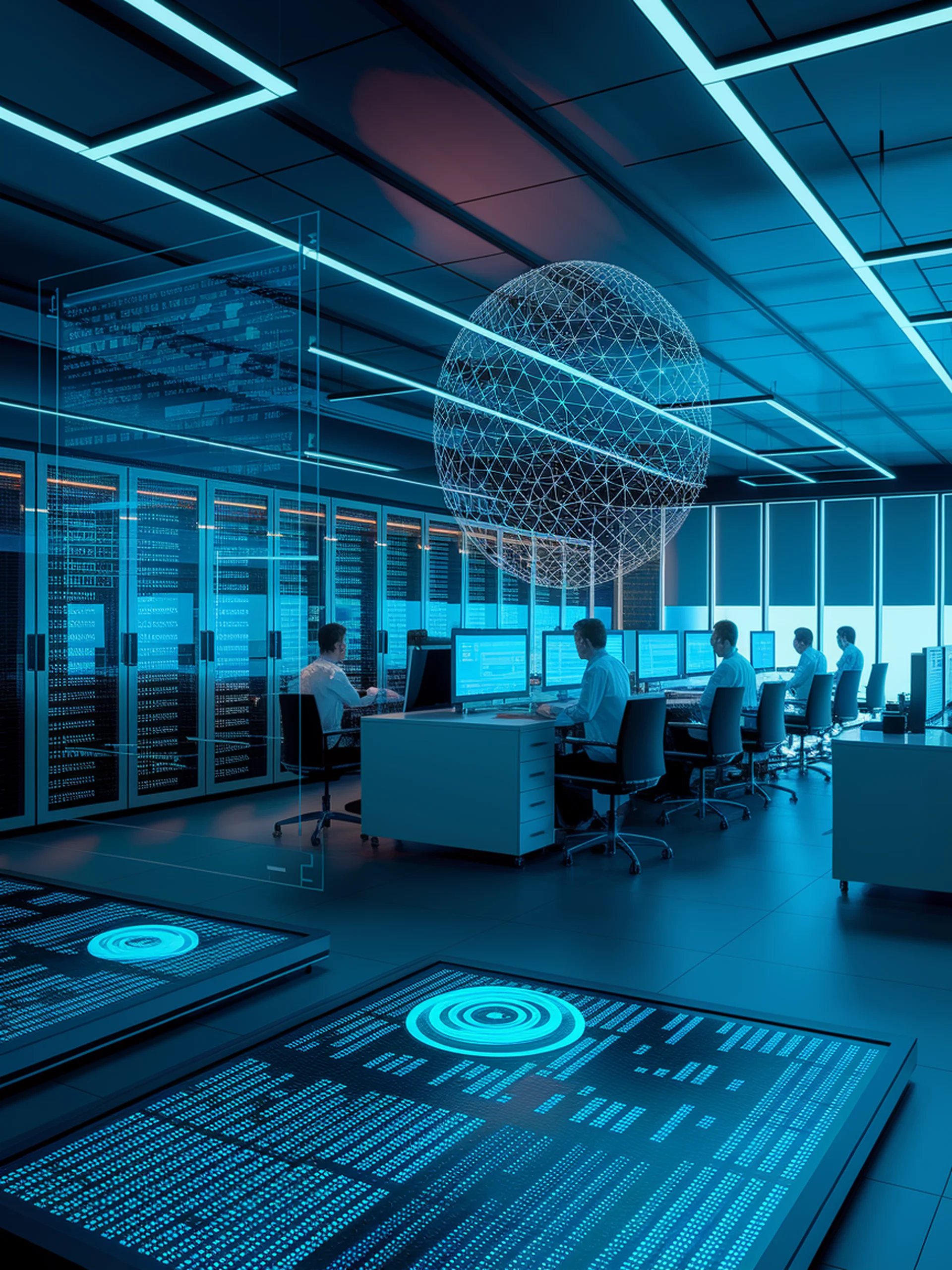
Protecting Multimodal Datasets from Unauthorized Use
A novel fingerprinting approach for vision-language models
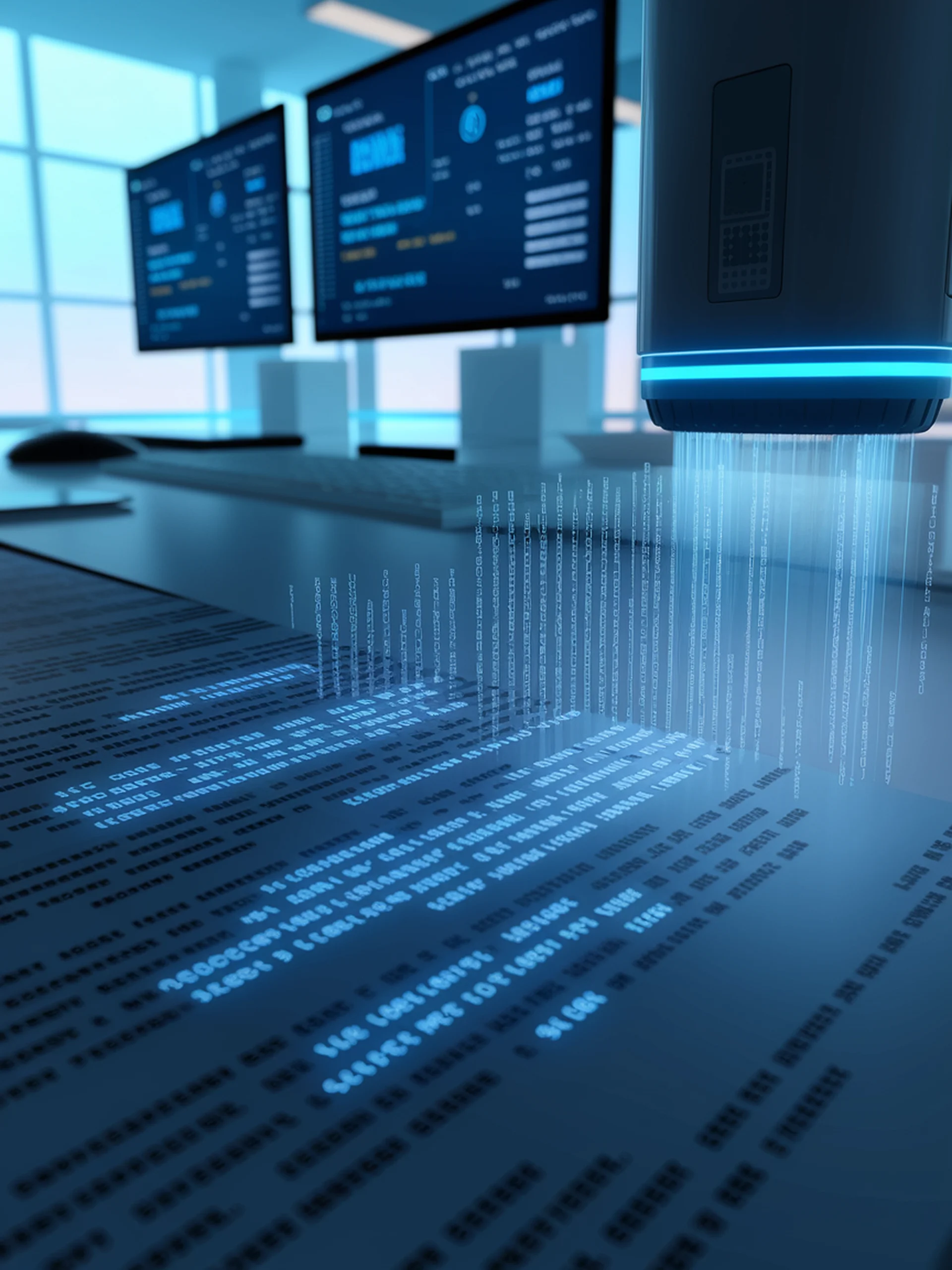
Securing LLM Content with Smart Watermarking
Entropy-guided approach balances detection and quality
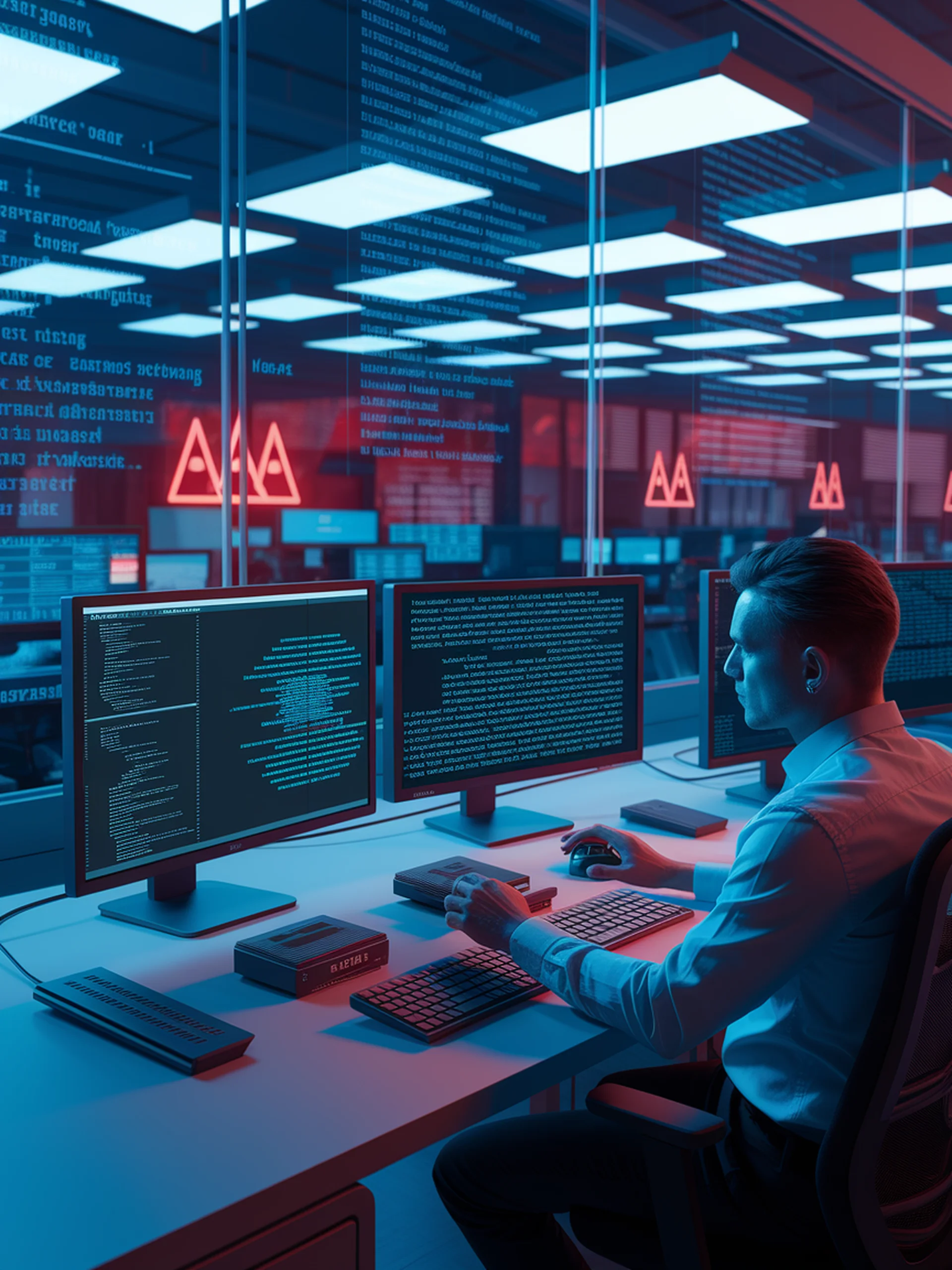