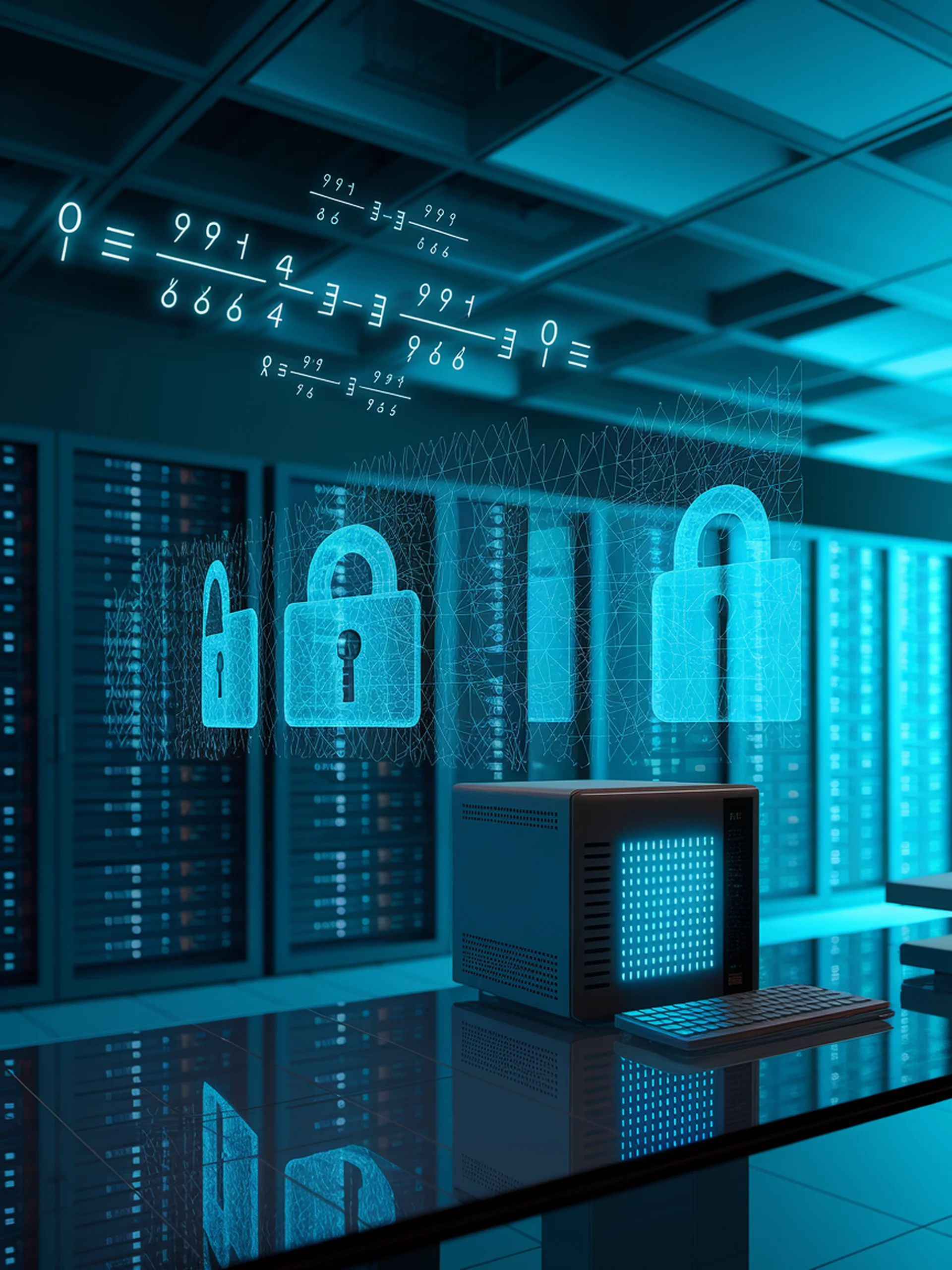
Securing LLMs with Taylor Expansion
A novel approach to protect ownership while enabling model sharing
TaylorMLP transforms LLM weights into Taylor series parameters, enabling secure model release while preserving ownership rights and preventing misuse.
- Ownership preservation through mathematical transformation rather than conventional encryption
- Prevents unauthorized use while maintaining functional capabilities of the original model
- Balances security and utility by enabling deployment without compromising ownership
- Novel security framework specifically designed for the LLM ecosystem
This research addresses a critical gap in AI security by providing model developers a way to release their work while maintaining control over their intellectual property, preventing unauthorized fine-tuning, and establishing a stronger security foundation for LLM deployment.
Taylor Unswift: Secured Weight Release for Large Language Models via Taylor Expansion