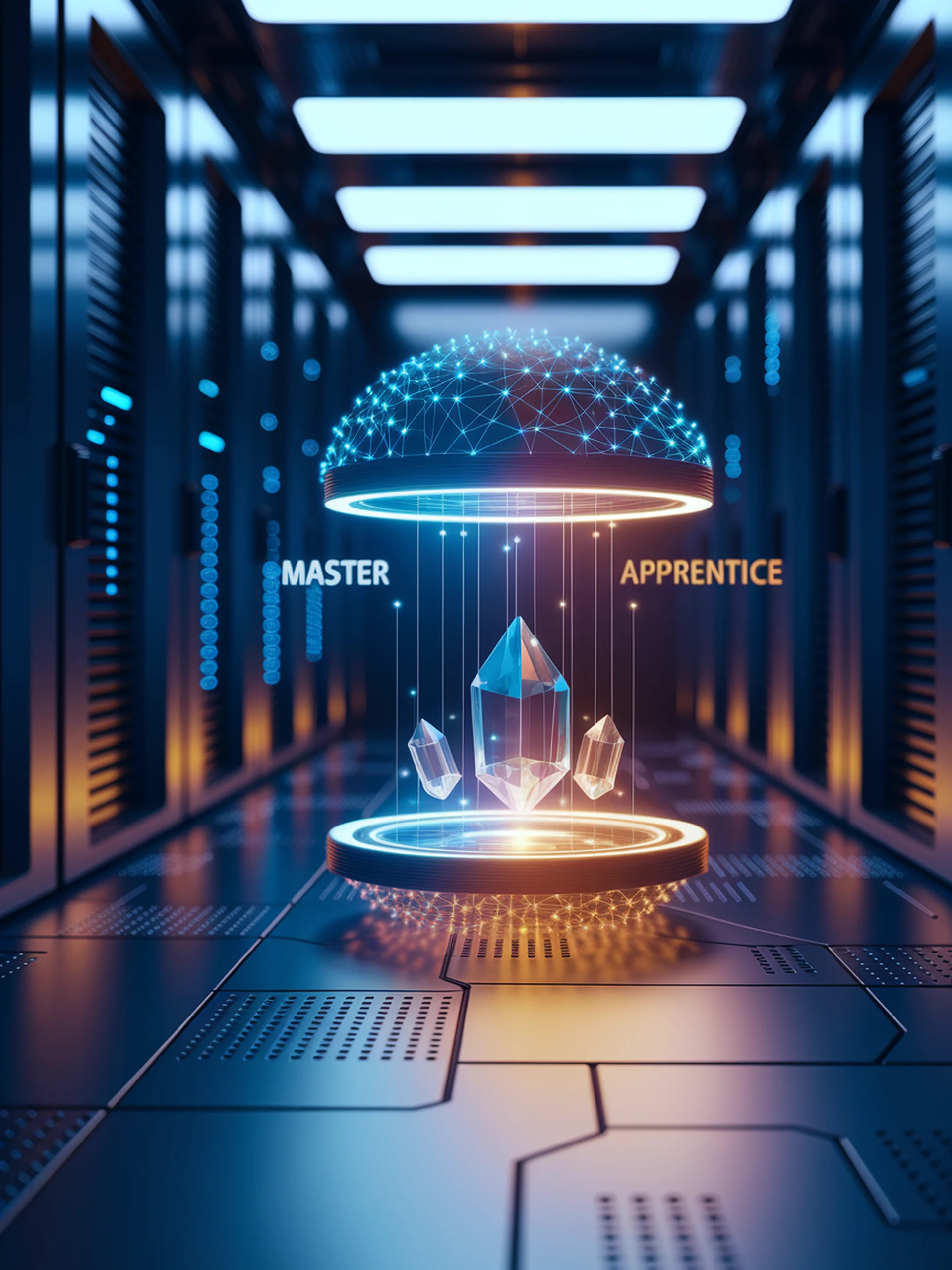
Master-Apprentice VLM Inference
Reducing costs while maintaining high-quality vision-language responses
Cache of Thought (CoT) introduces a collaborative framework between large and small Vision Language Models to optimize cost-effectiveness without sacrificing quality.
- Creates a knowledge cache from the large 'master' model that small 'apprentice' models can leverage
- Achieves similar quality to large models at significantly lower costs
- Enables dynamic decision-making on when to use smaller vs. larger models
- Provides practical solution for deploying VLMs in resource-constrained environments
This engineering innovation matters because it addresses the critical cost-quality tradeoff in deploying vision-language models at scale, making advanced AI capabilities more accessible and economically viable for real-world applications.
Cache-of-Thought: Master-Apprentice Framework for Cost-Effective Vision Language Model Inference