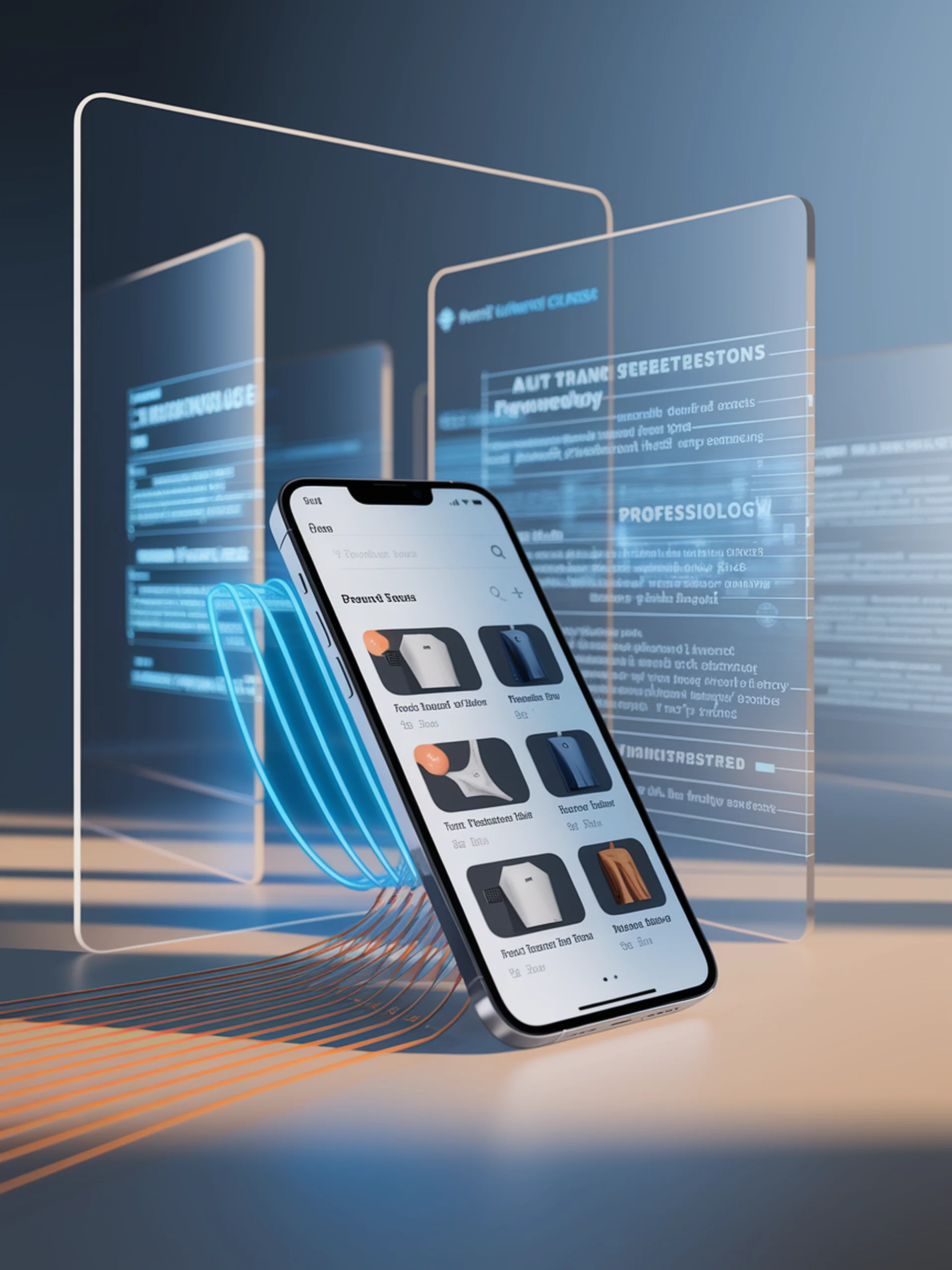
Enhancing E-Commerce Search with LLMs
A Multi-dimensional Distillation Approach for Better Relevance Learning
This research introduces a novel explainable LLM-driven framework for e-commerce search that improves query-item relevance while maintaining efficiency.
- Leverages LLMs' knowledge while addressing their practical limitations in e-commerce environments
- Implements multi-dimensional distillation to transfer LLM capabilities to smaller, specialized models
- Creates explainable relevance judgments through semantic dimension modeling
- Achieves superior performance particularly for long-tail queries
This engineering advancement matters because it bridges the gap between powerful but resource-intensive LLMs and the practical needs of e-commerce platforms, enabling better search experiences without compromising system efficiency.
Explainable LLM-driven Multi-dimensional Distillation for E-Commerce Relevance Learning