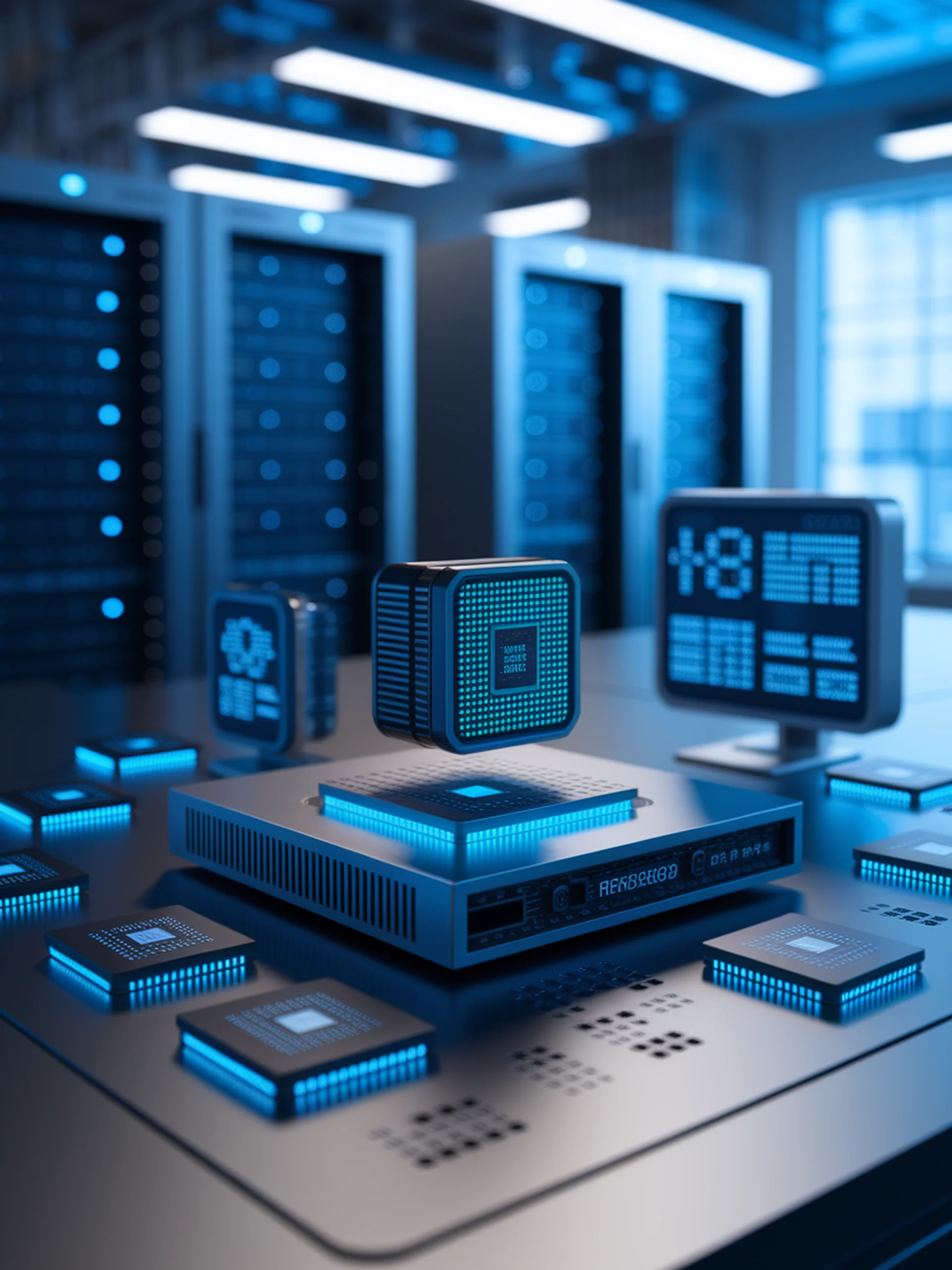
Smarter Pruning for Smaller LMs
Adaptive structural pruning outperforms traditional approaches
Adapt-Pruner offers a novel approach to train small language models that are both high-performing and computationally efficient.
- Dynamically prunes model structure during training rather than after pre-training
- Achieves better performance than traditional pre-training or post-training pruning
- Reduces computational costs by up to 50% compared to training from scratch
- Enables deployment of powerful language models on resource-constrained edge devices
This engineering breakthrough makes efficient AI more accessible for real-world applications where computational resources are limited, opening new possibilities for on-device AI.
Adapt-Pruner: Adaptive Structural Pruning for Efficient Small Language Model Training