Edge Computing and TinyML
Deployment of LLMs in resource-constrained edge devices and IoT applications with optimization for efficiency
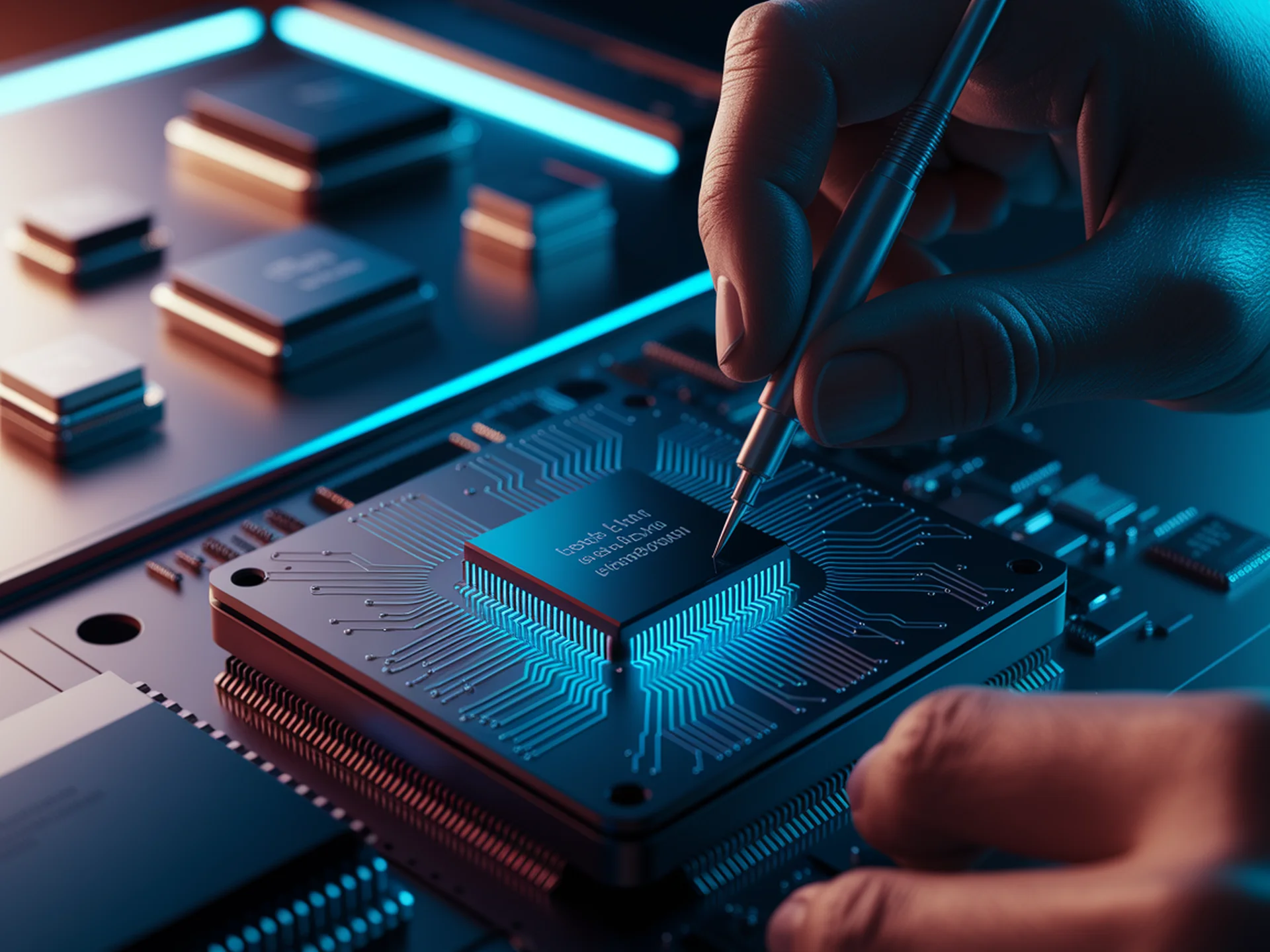
Edge Computing and TinyML
Research on Large Language Models in Edge Computing and TinyML
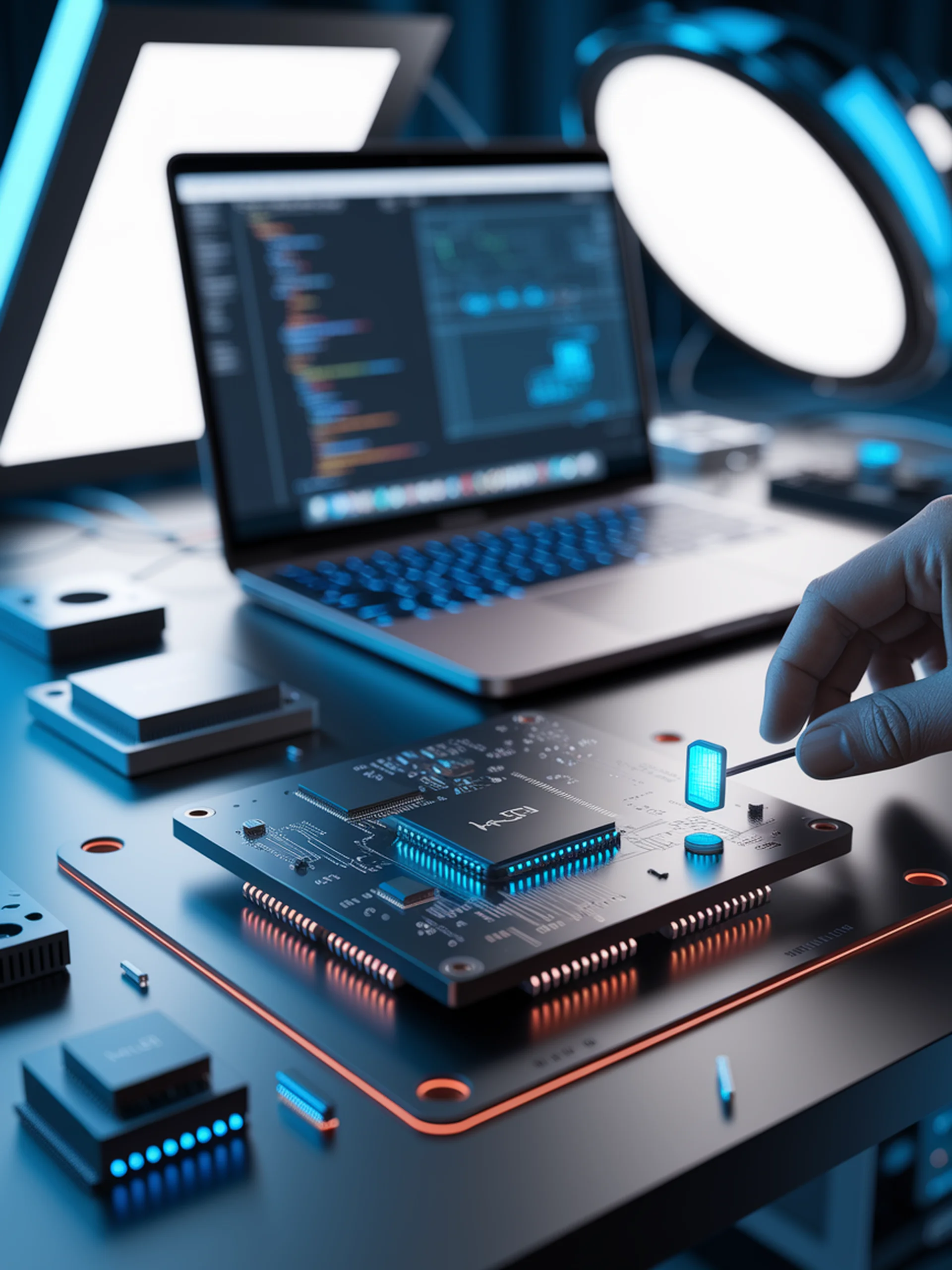
LLMs as TinyML Lifecycle Enablers
Leveraging Large Language Models to streamline embedded AI development
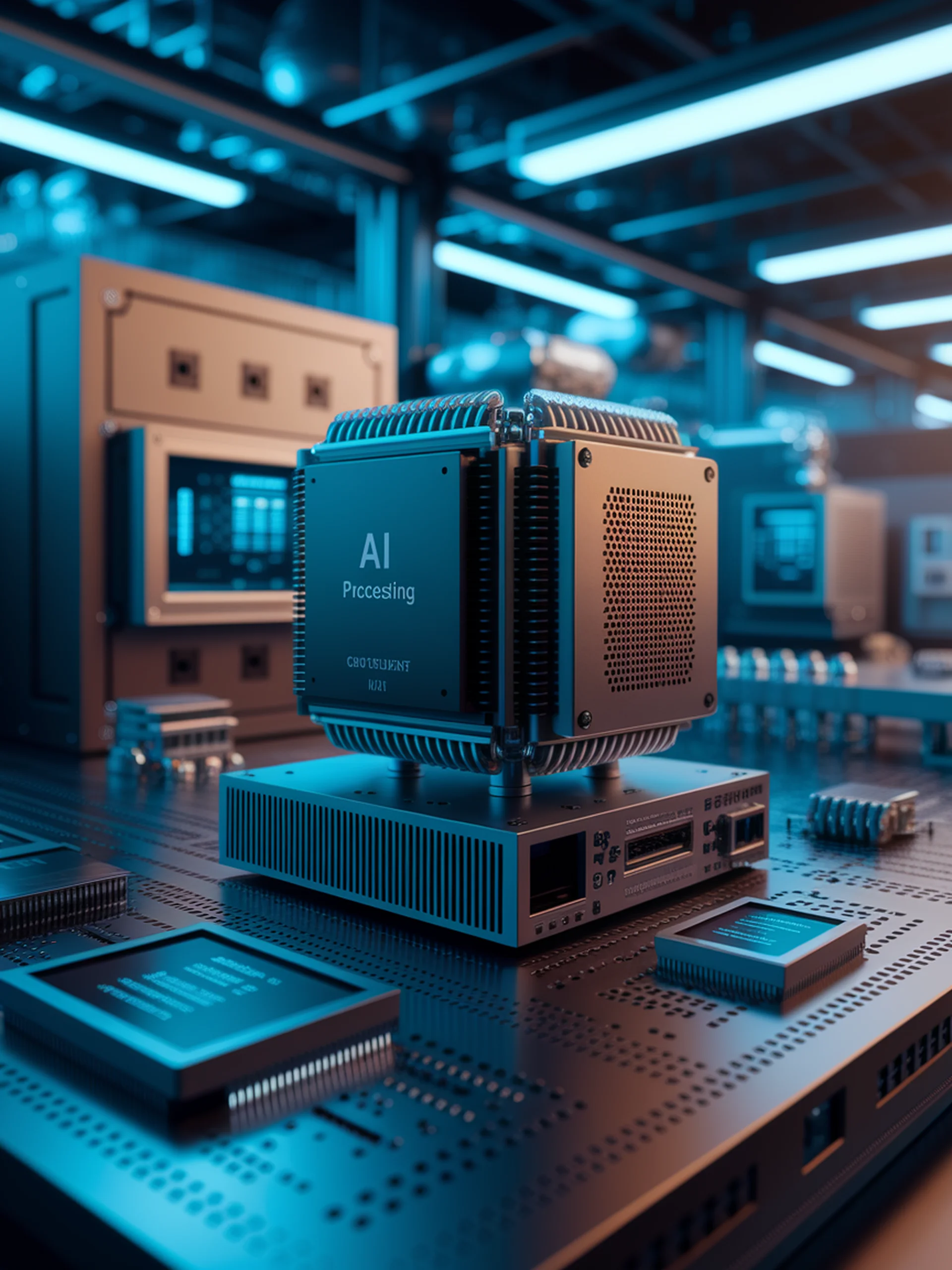
Accelerating AI at the Edge
Optimizing Deep Learning for Resource-Constrained Environments
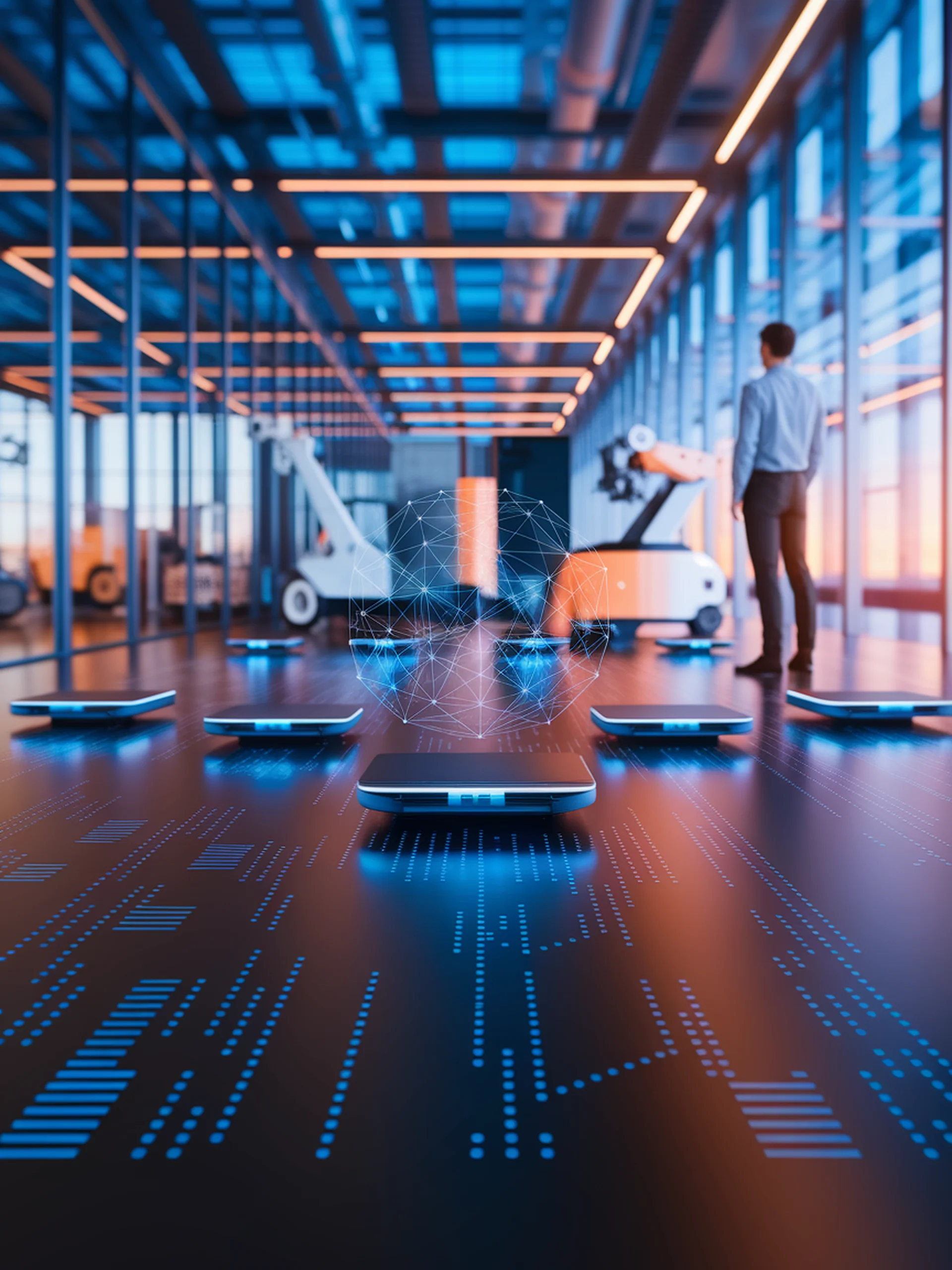
Next-Gen AI for Connected Systems
Reimagining CPS-IoT with Foundation Models
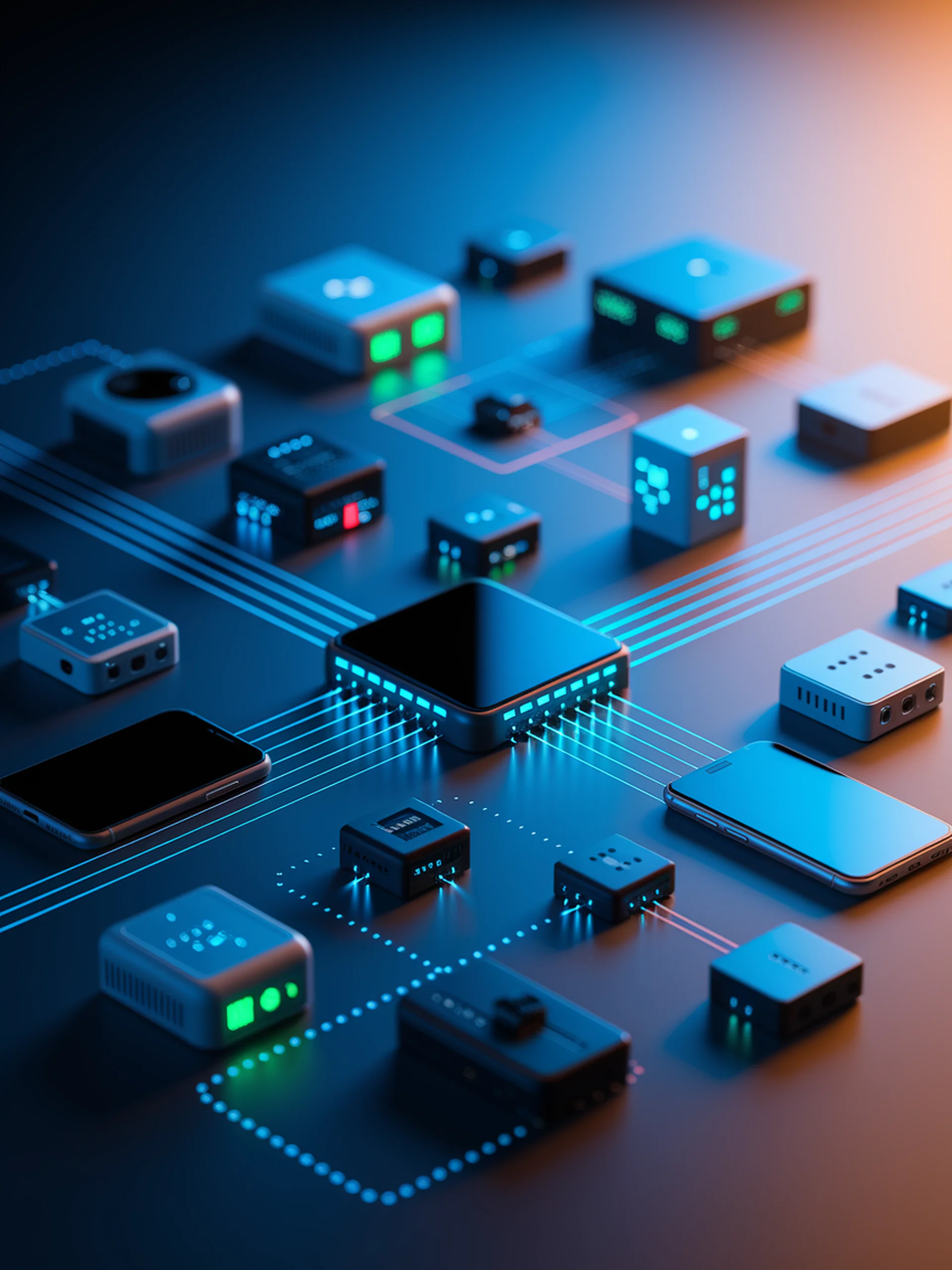
Efficient LLM Fine-Tuning on Edge Devices
A novel federated sketching approach for resource-constrained environments
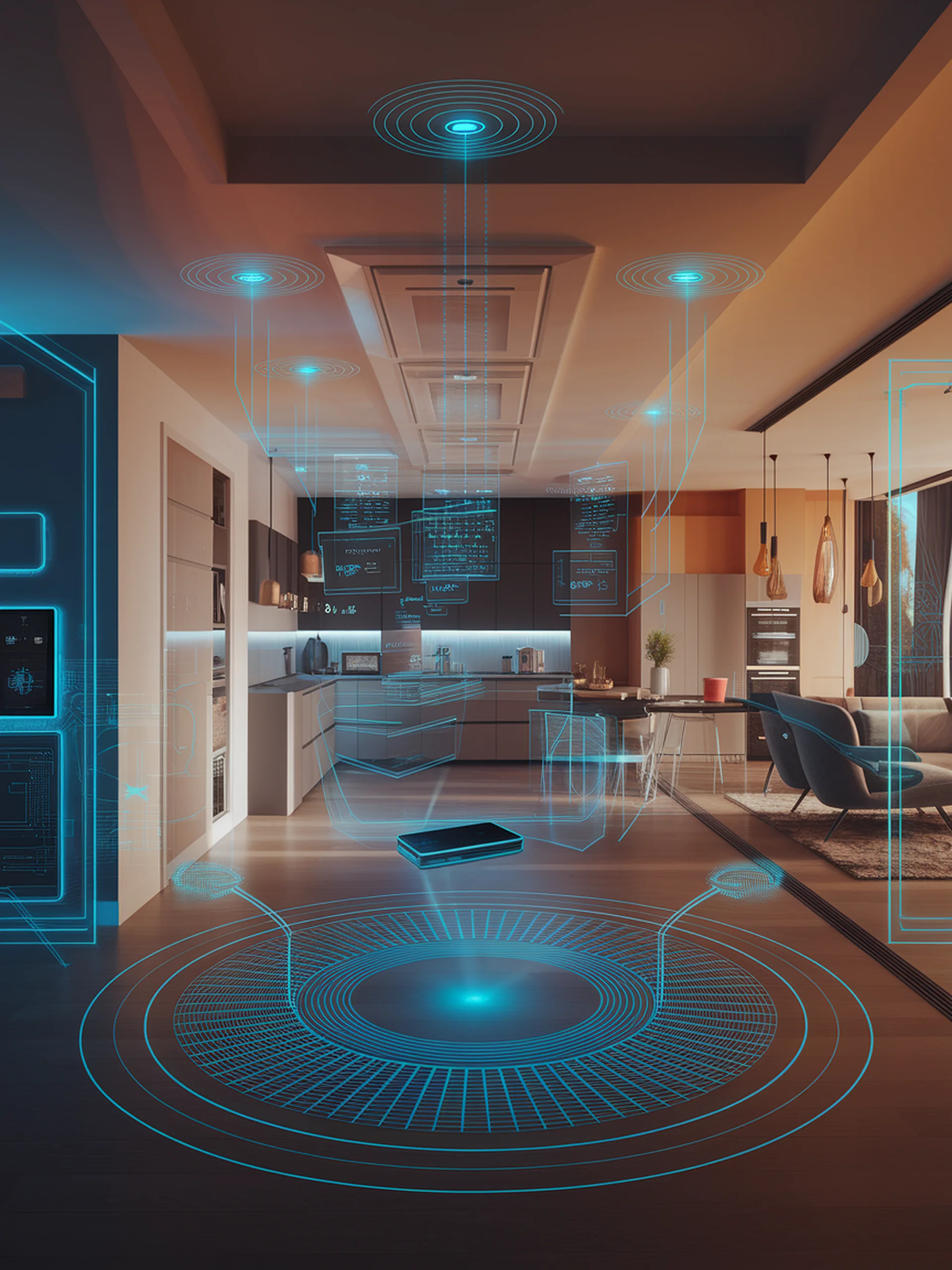
Intelligent IoT Systems Through LLMs
Enabling Dynamic IoT Environments via Mixed-Initiative Interaction
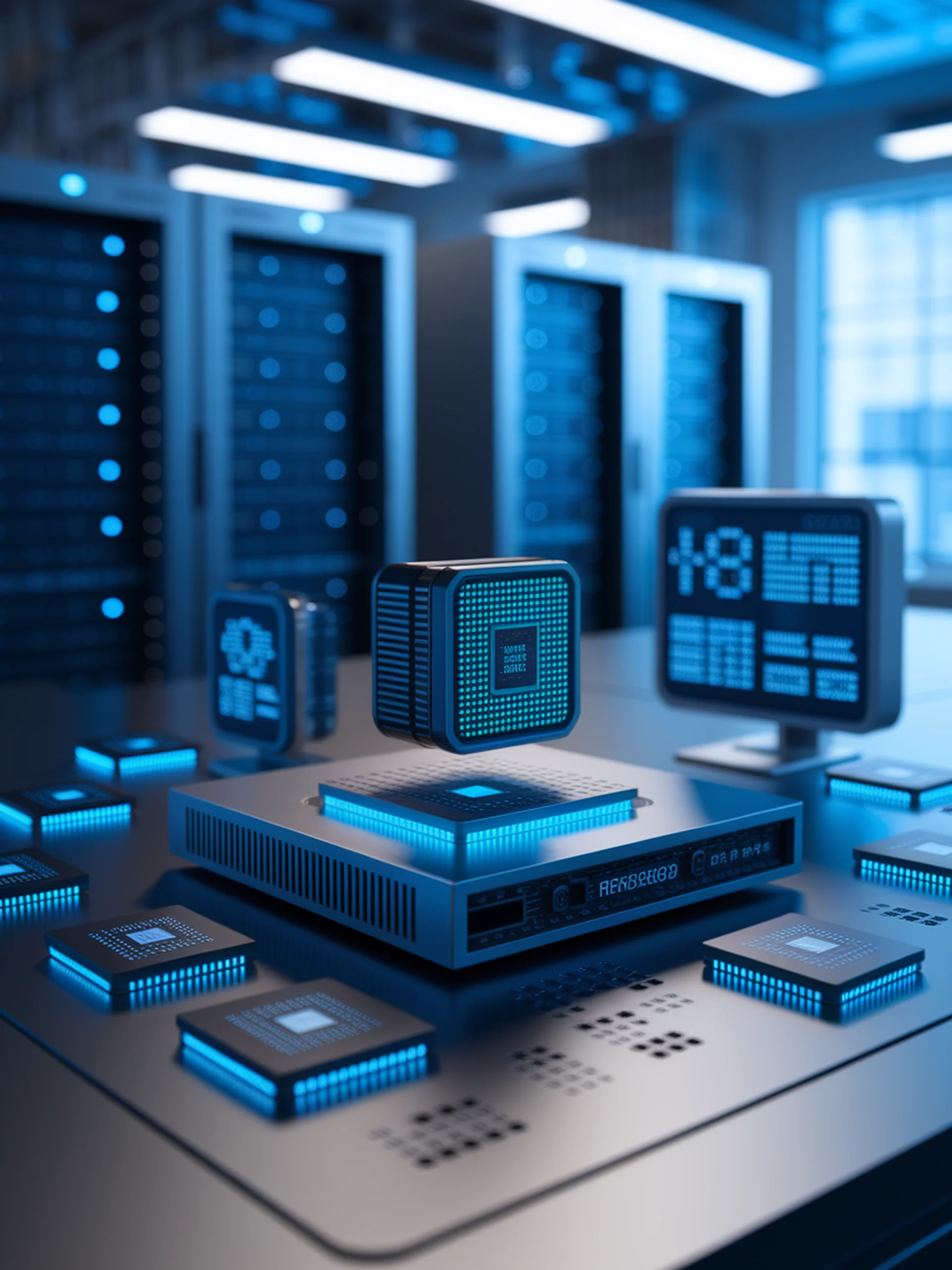
Smarter Pruning for Smaller LMs
Adaptive structural pruning outperforms traditional approaches
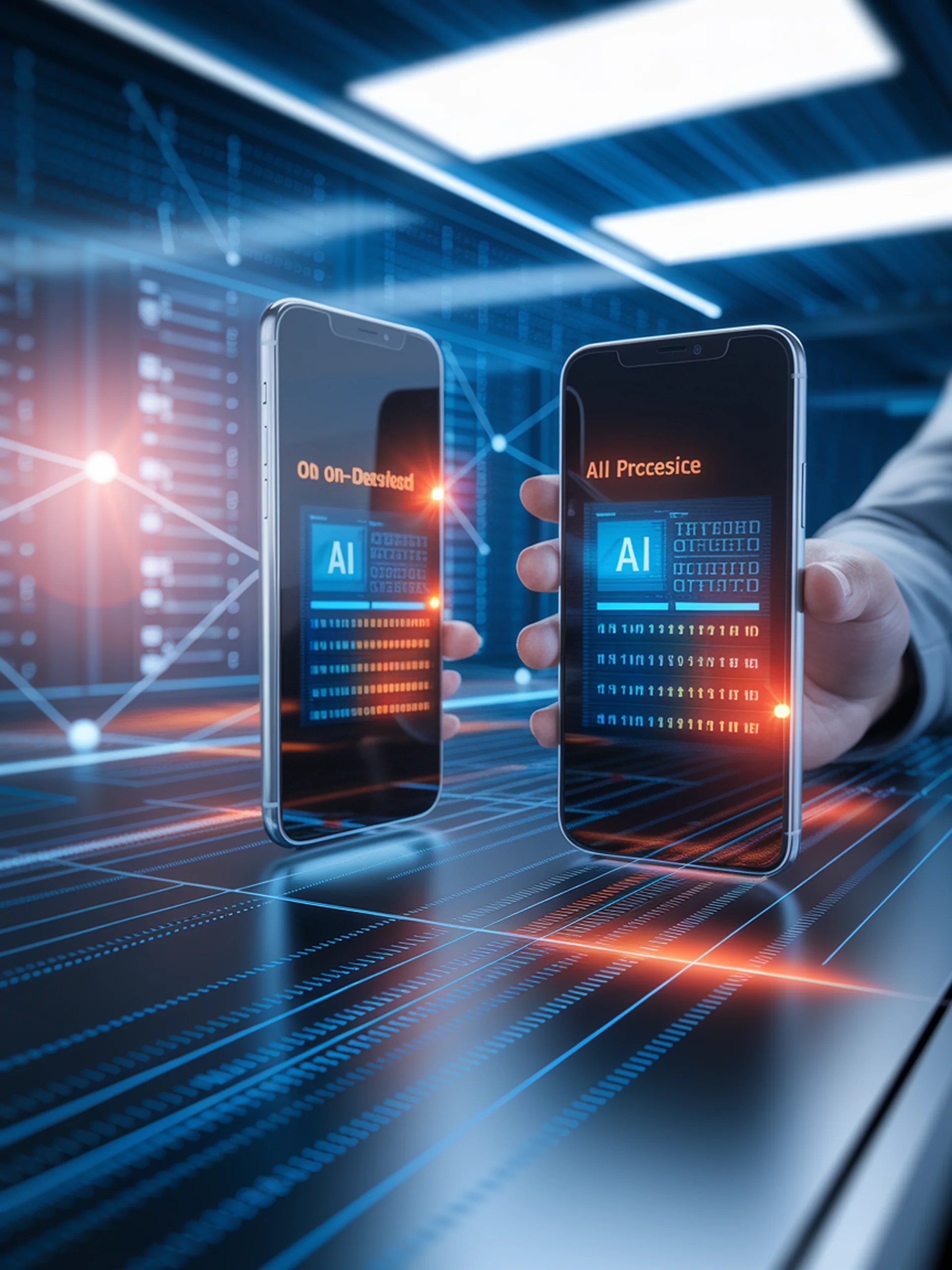
Smart Routing for On-Device AI
Optimizing LLM Performance Through Uncertainty-Based Decision Making
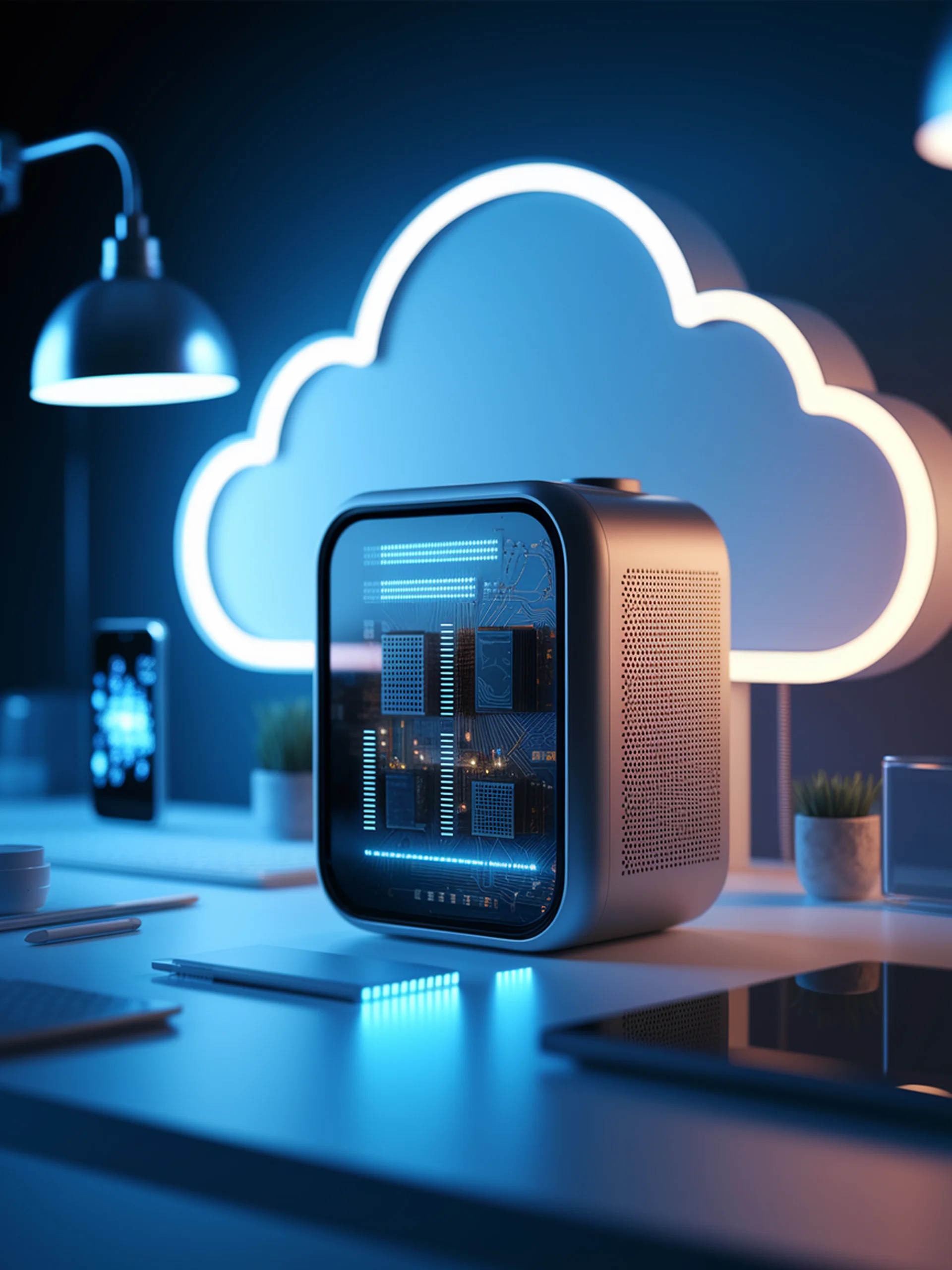
Bringing LLMs to the Edge
Innovative pruning-aware pretraining for efficient language models
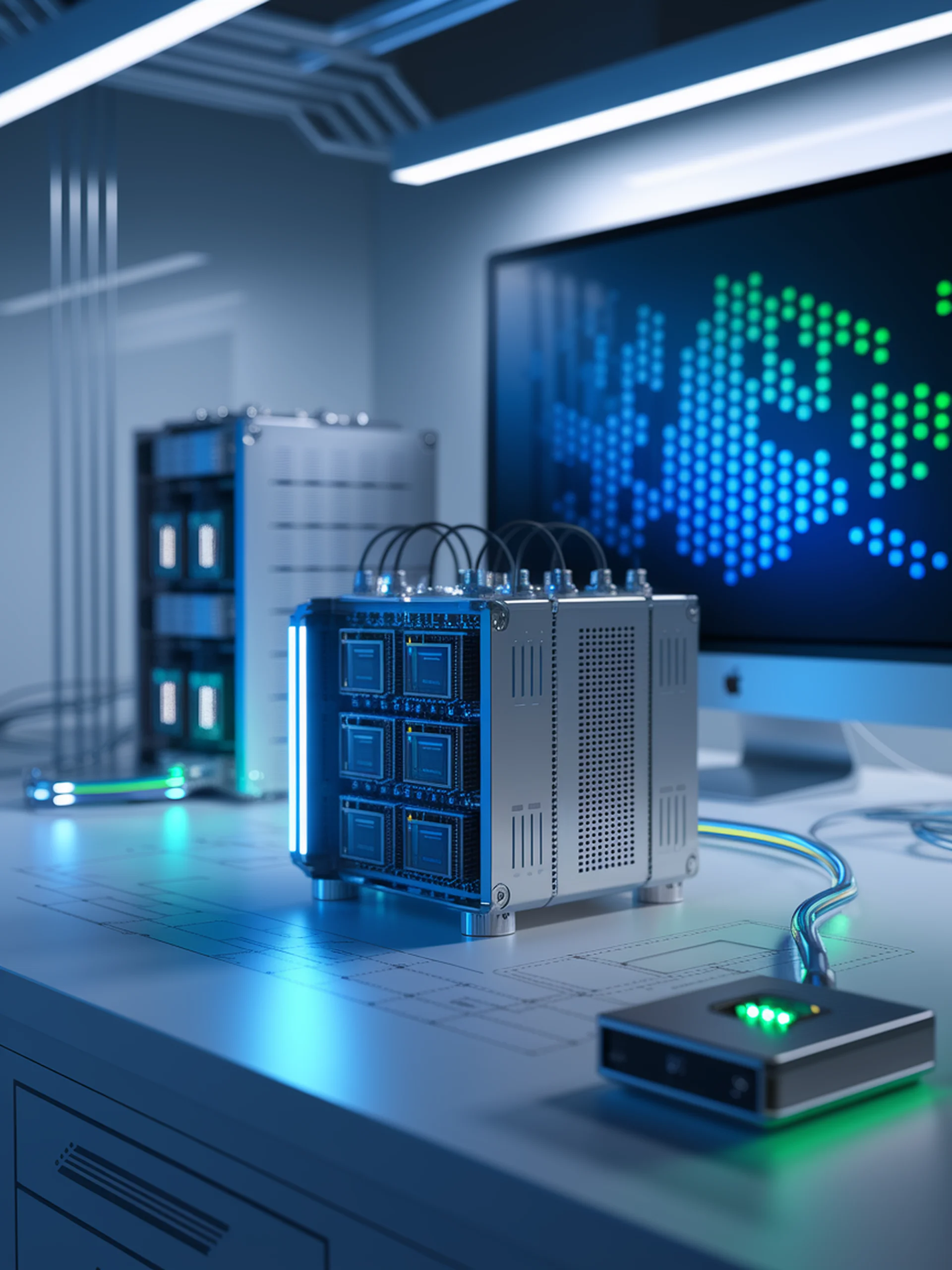
Accelerating Graph Neural Networks at the Edge
Hardware-optimized GNN execution for resource-constrained environments
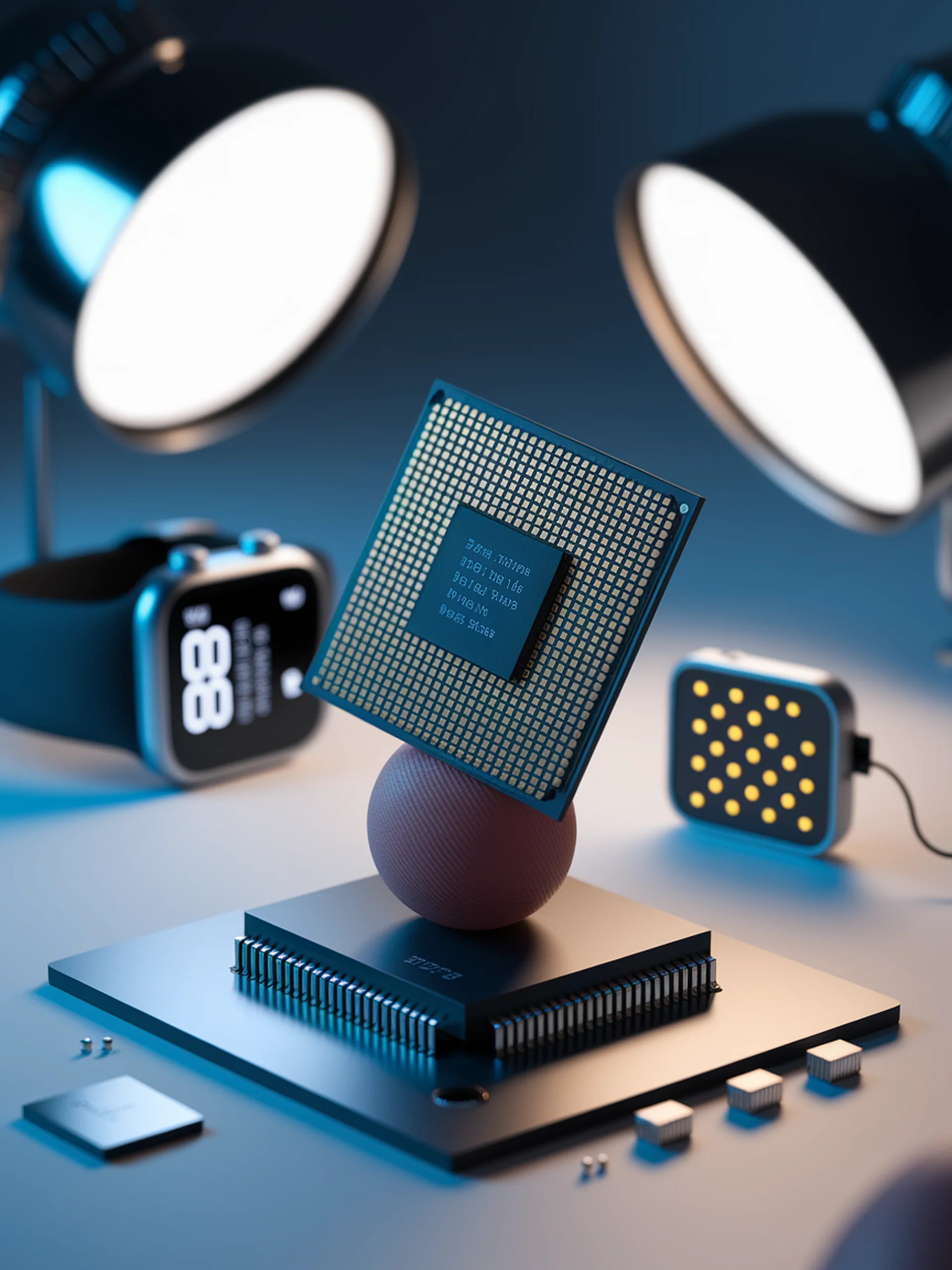
Breaking Memory Barriers in NLP
Making Large Language Models Work on Tiny Devices
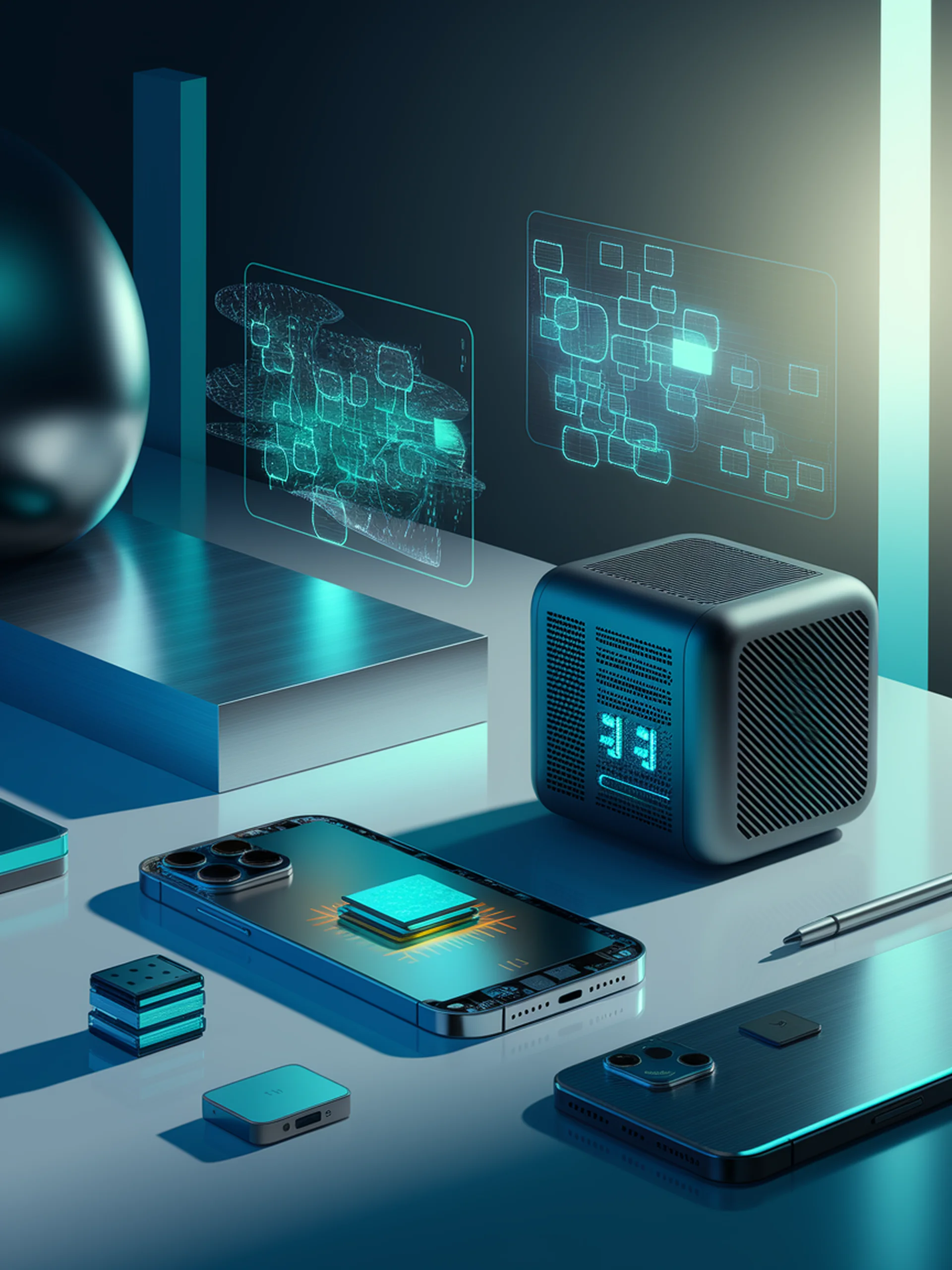
Efficient LLM Fine-Tuning at the Edge
Optimizing language models for resource-constrained devices
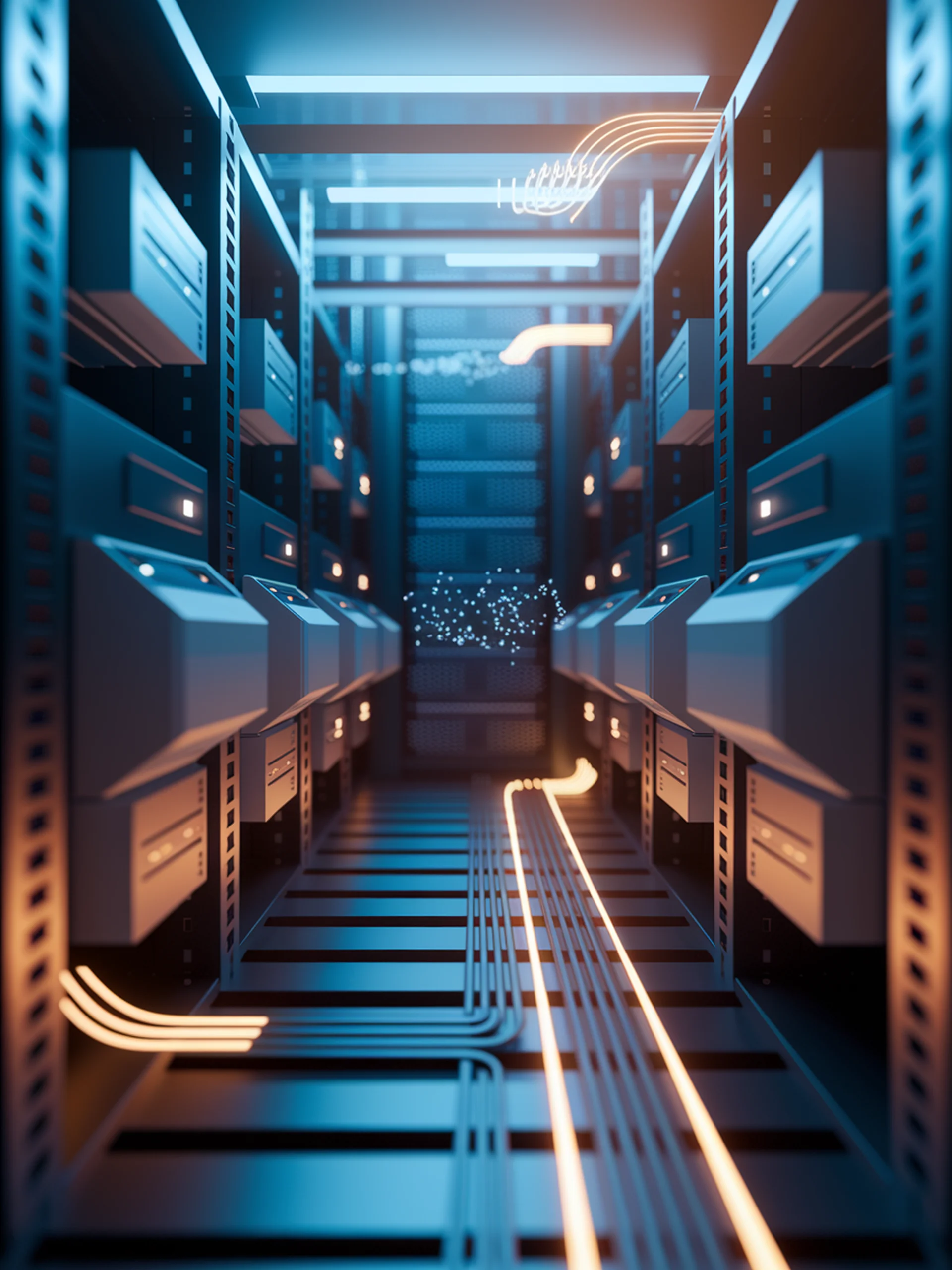
Optimizing MLLMs for Edge-Cloud Federated Learning
A Swarm Intelligence Approach to Deploy Advanced AI Models at the Edge
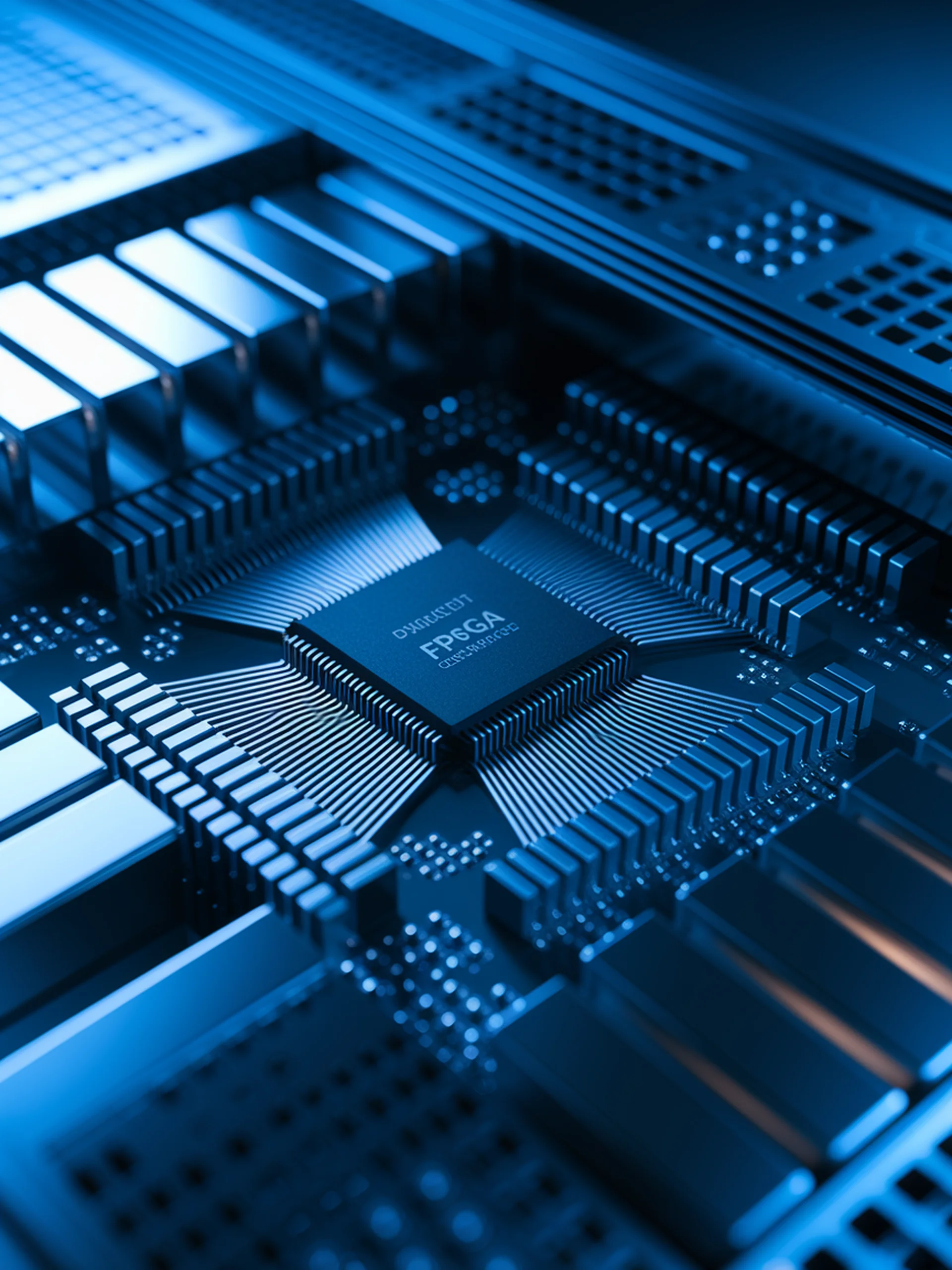
Breaking Memory Limits for Edge-Based LLMs
Maximizing FPGA potential for efficient LLM deployment at the edge
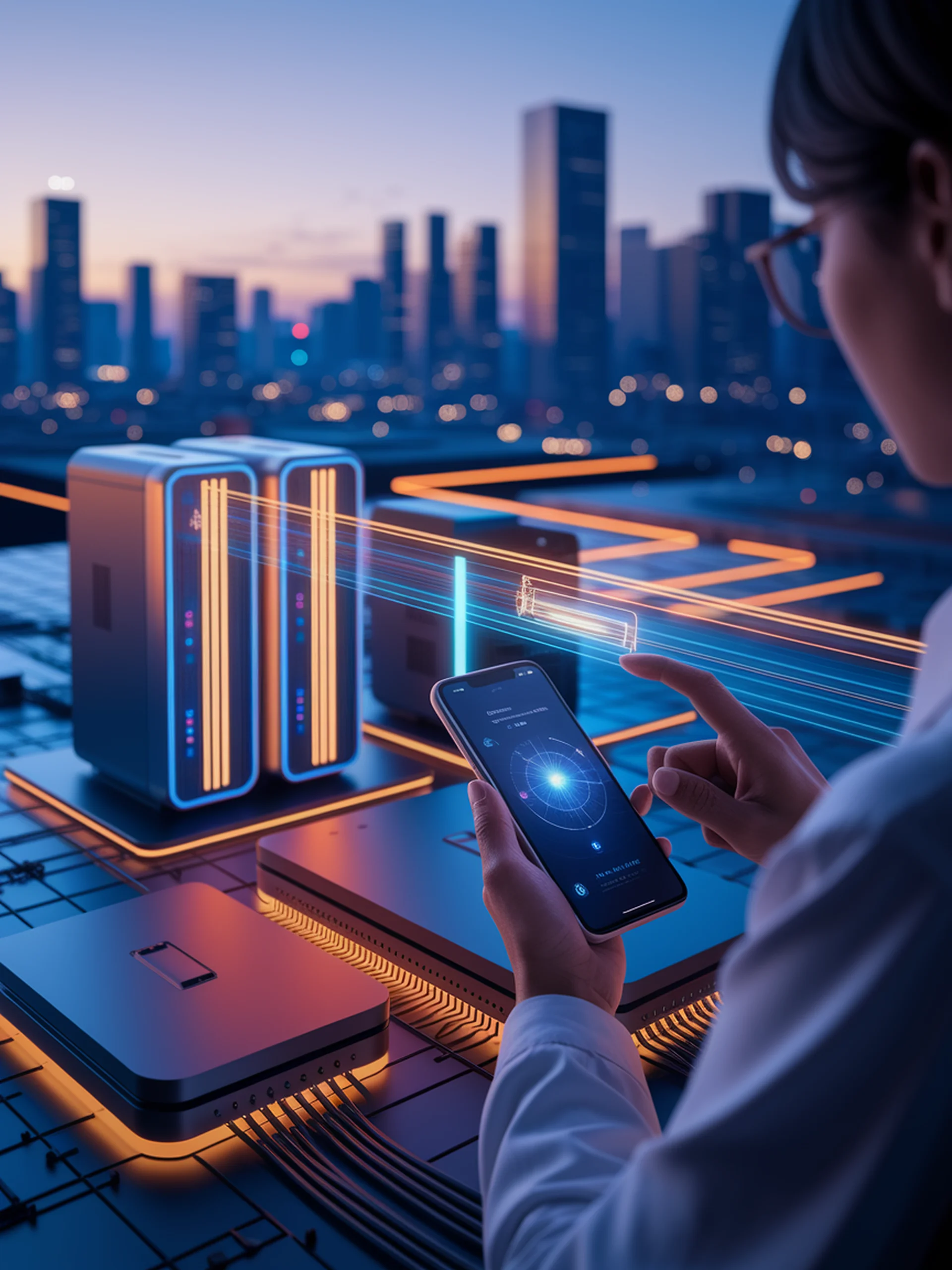
Edge-Powered Mobile AIGC Services
Enhancing AI Content Generation through Interactive Prompts and Dynamic Service Allocation
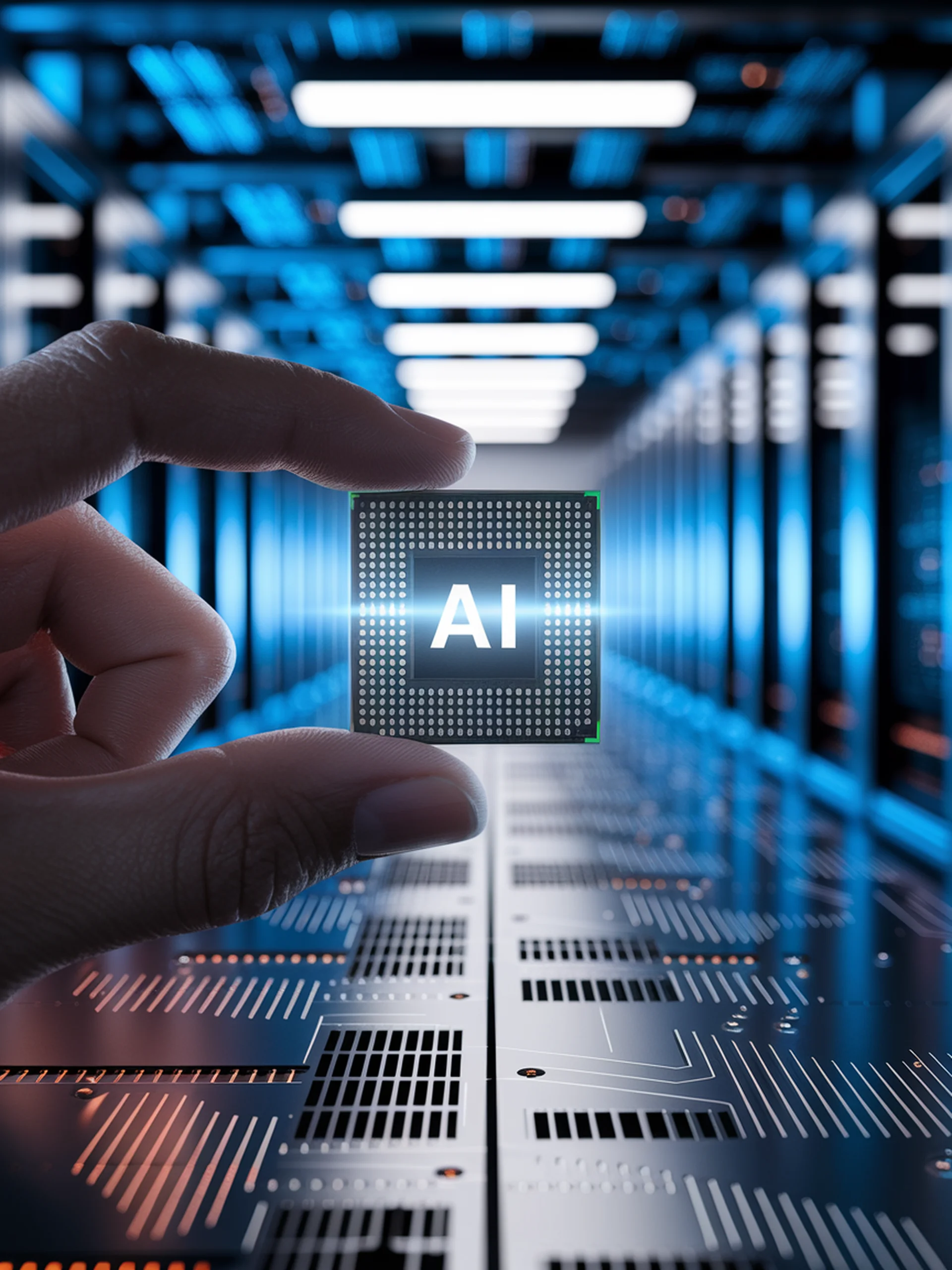
Smaller, Smarter AI Models
Building Efficient Models with Strong Reasoning Capabilities
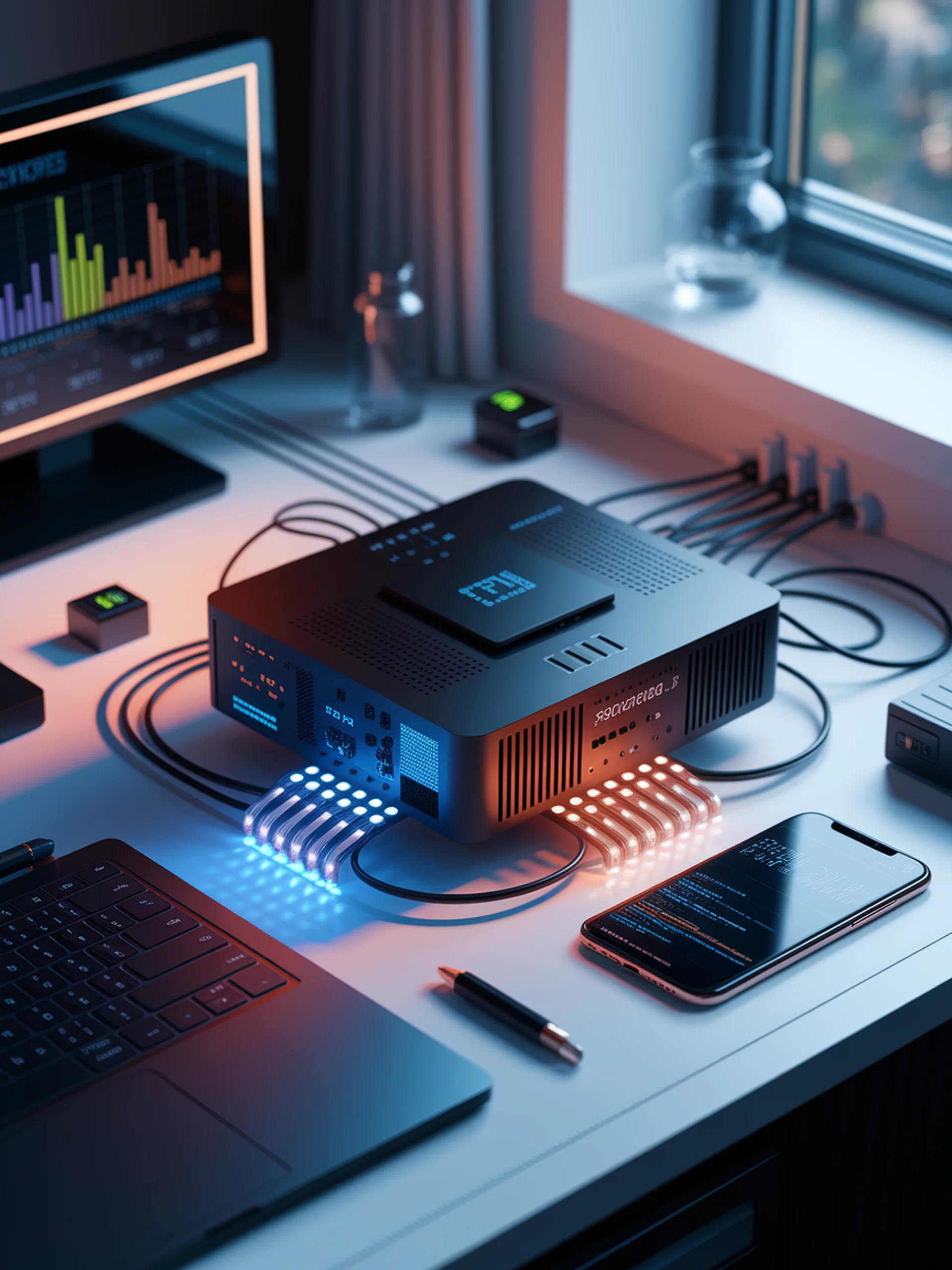
Efficient Edge Inference for Ternary LLMs
Accelerating Large Language Models on Resource-Constrained Devices
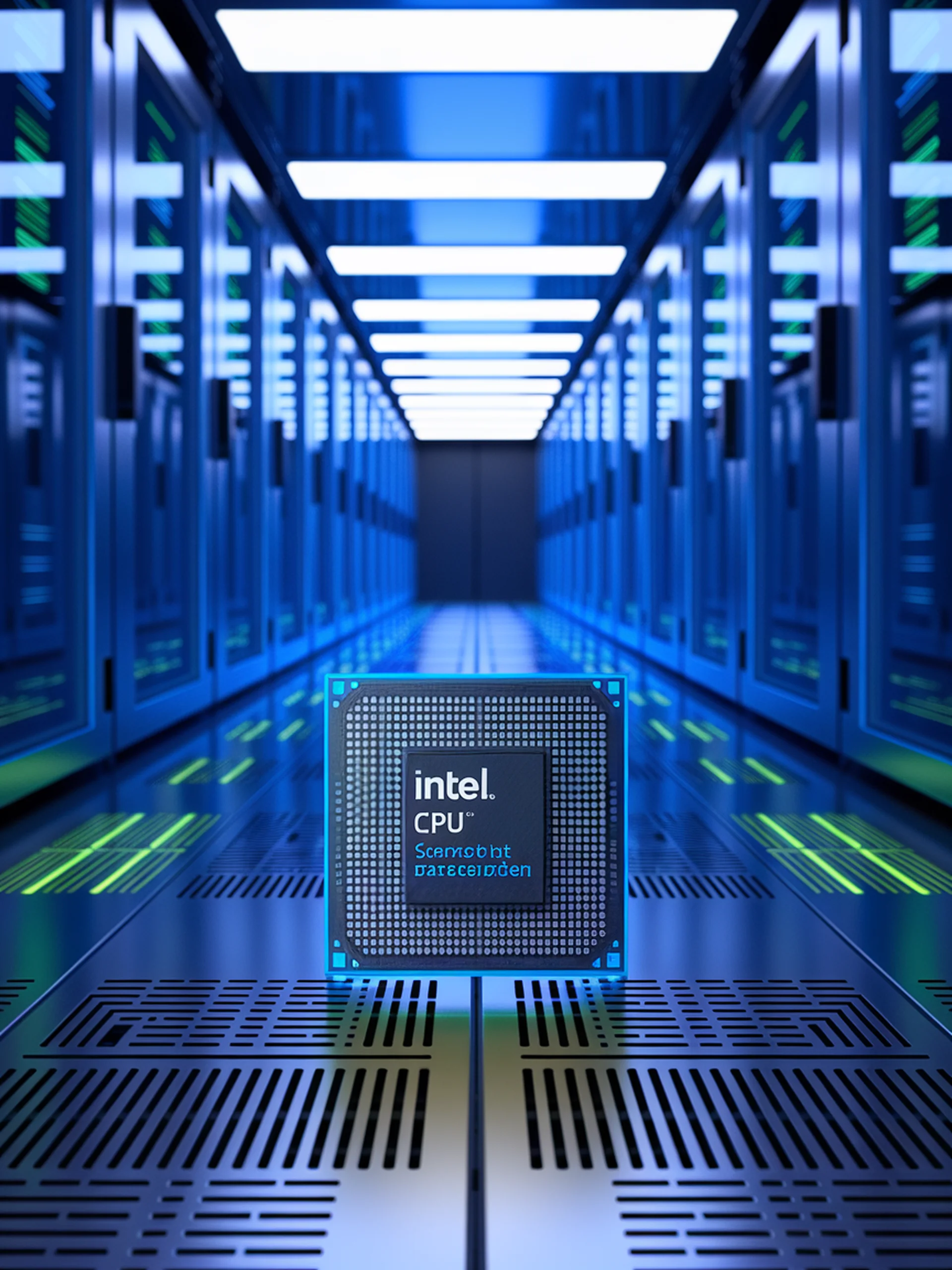
Supercharging LLMs on Standard CPUs
How SparAMX makes AI more accessible through CPU optimization
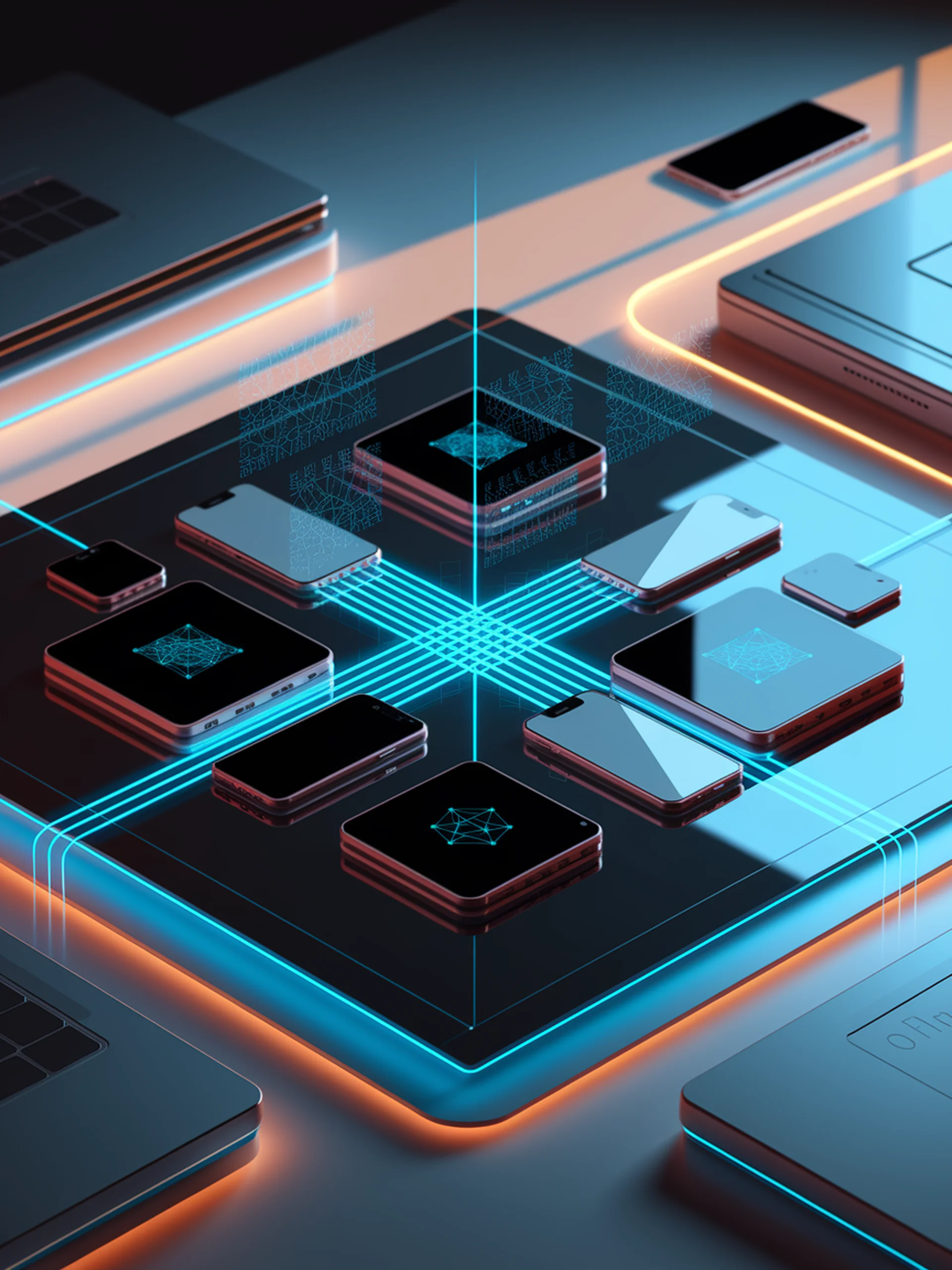
Enabling LLMs on Edge Devices
A breakthrough approach for distributed LLM inference across multiple devices
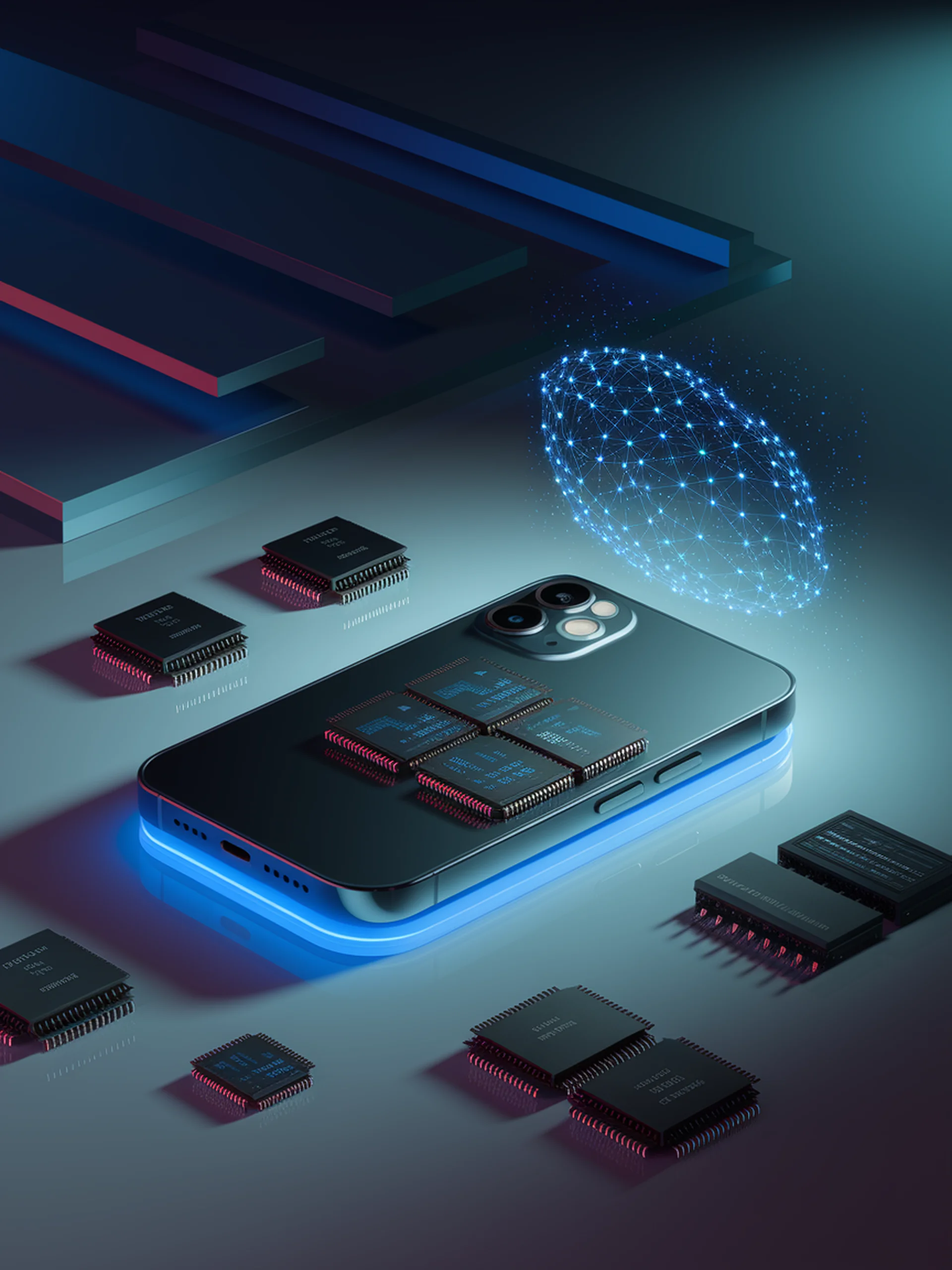
Revolutionizing On-Device LLM Fine-Tuning
Fully Quantized Training with Integer-Only Operations
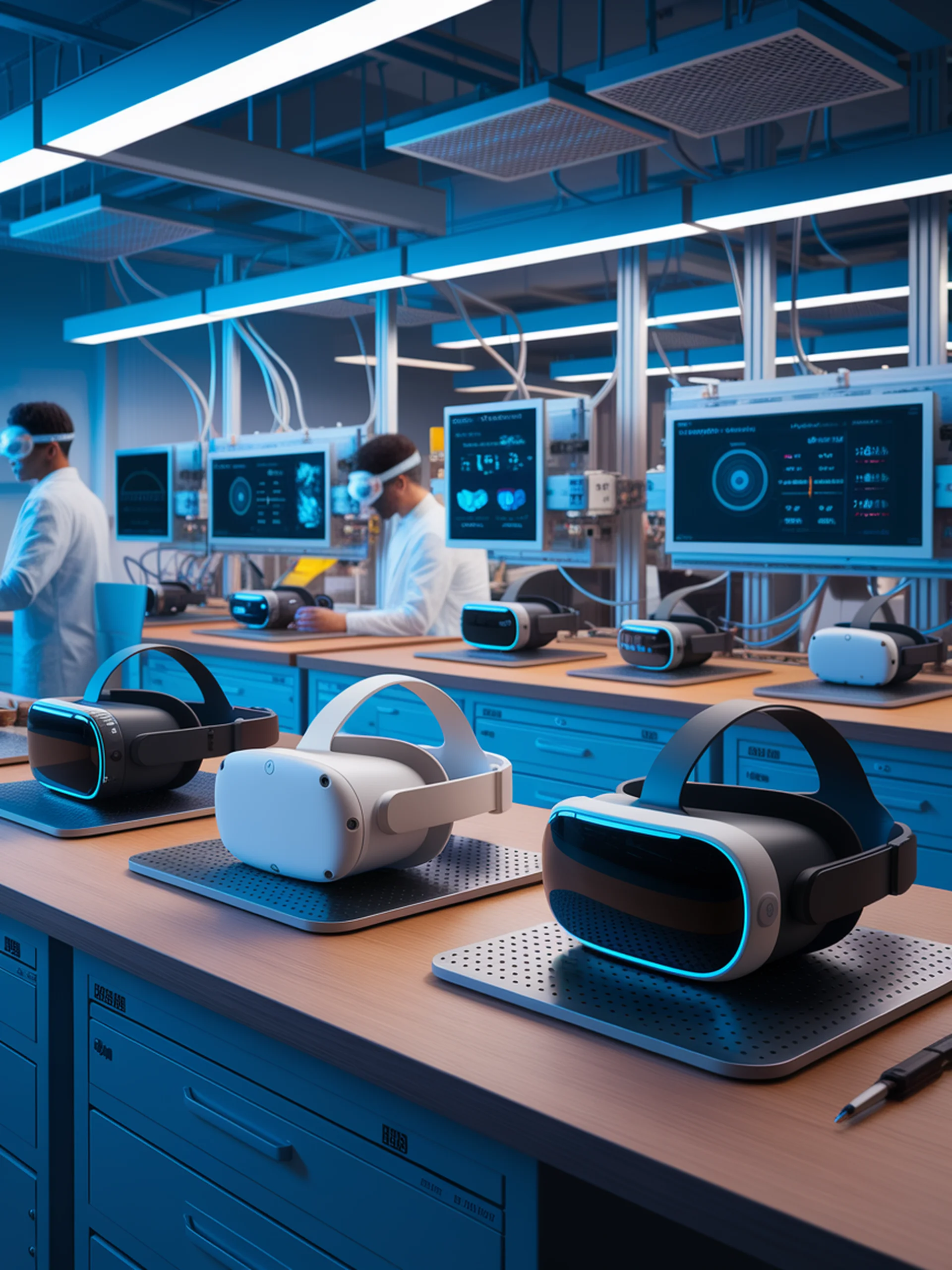
LLMs at the Edge: XR Device Performance Analysis
Benchmarking 17 language models across leading XR platforms
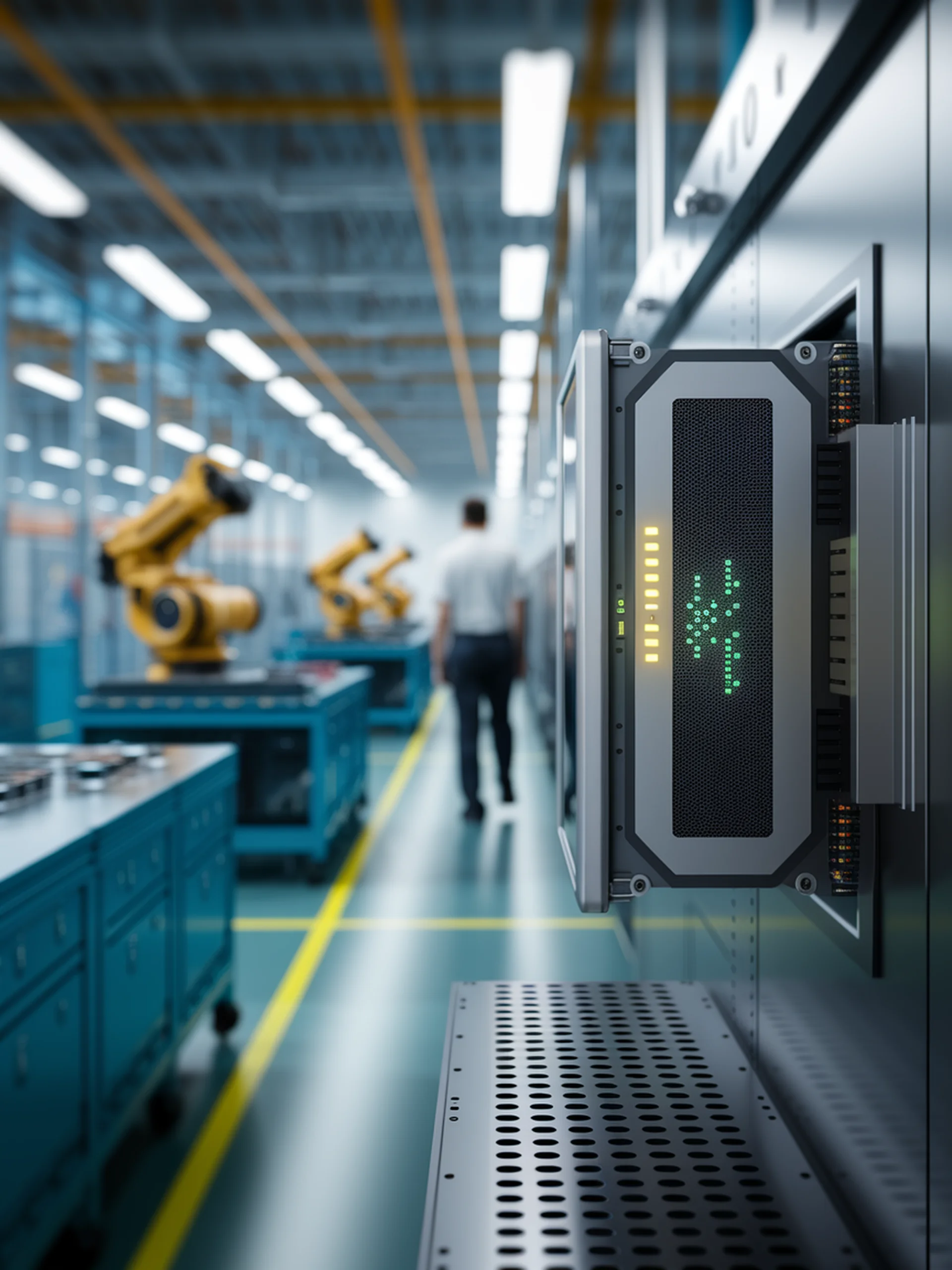
Bringing GenAI to the Edge
Optimizing AI models for secure, low-latency deployment on edge devices
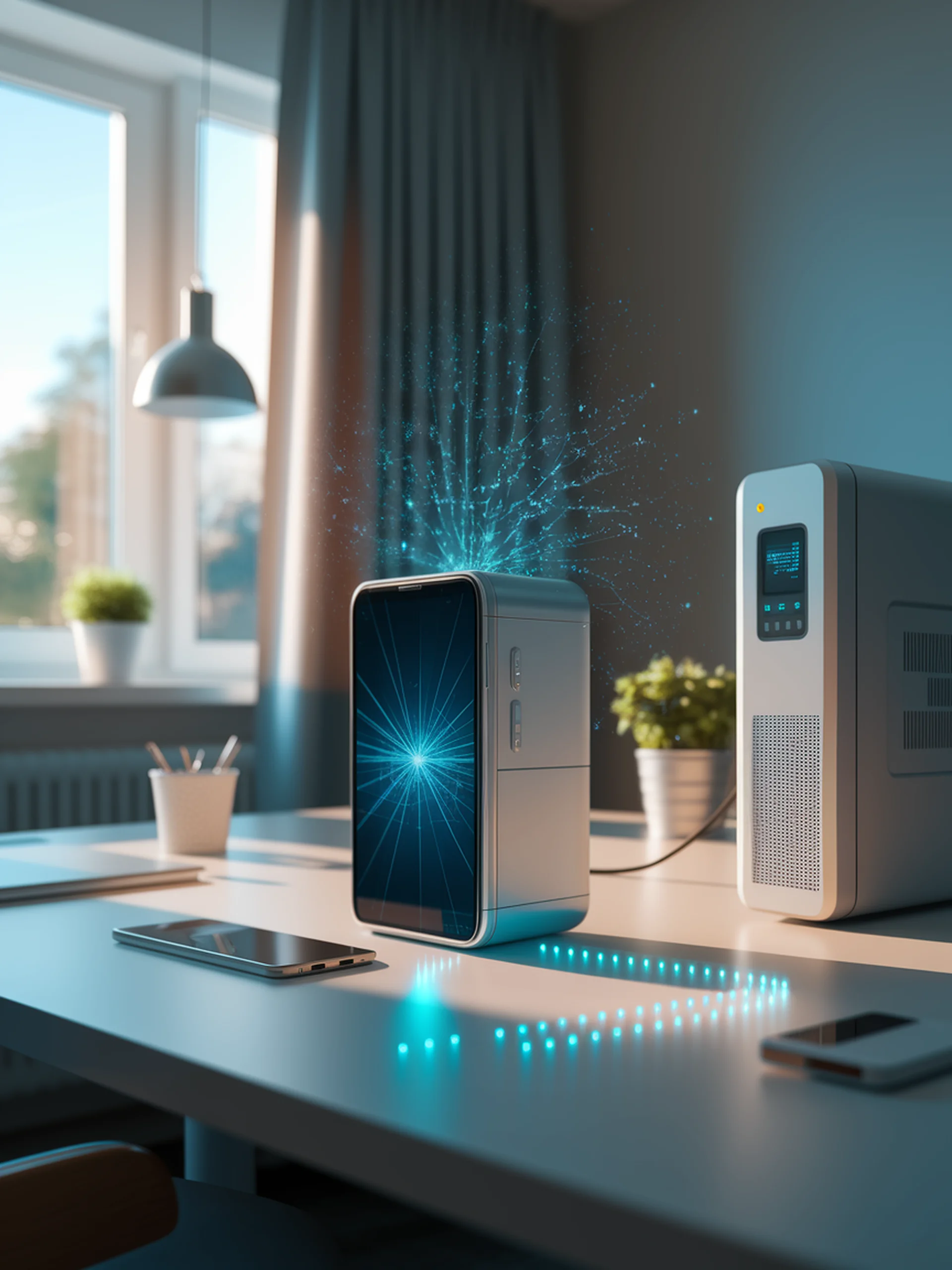
Mobile-Friendly LLM Fine-Tuning
Enabling personalized AI on resource-constrained devices
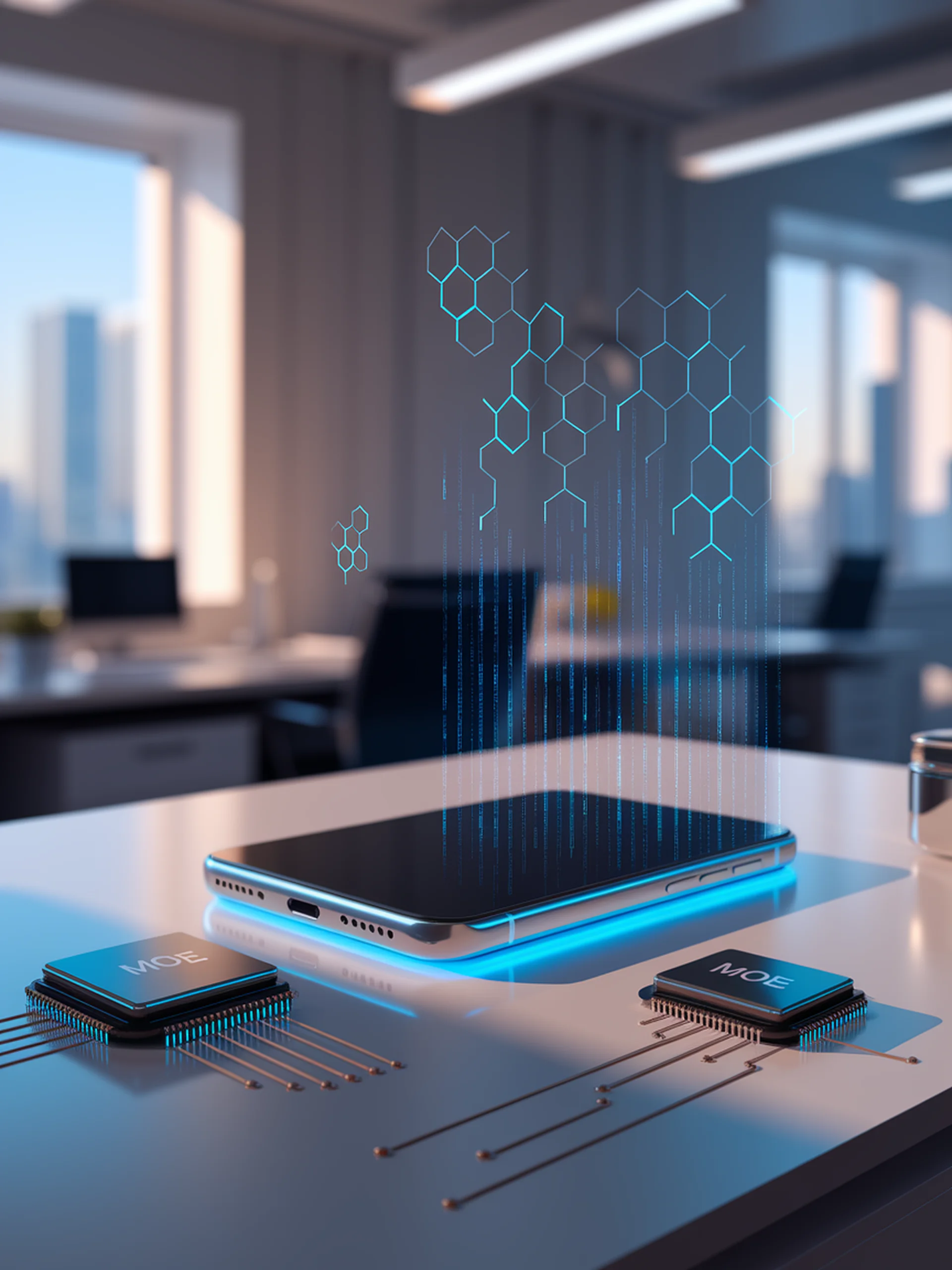
Bringing AI Power to Your Device
CoSMoEs: Making Advanced AI Models Run Efficiently on Mobile Devices
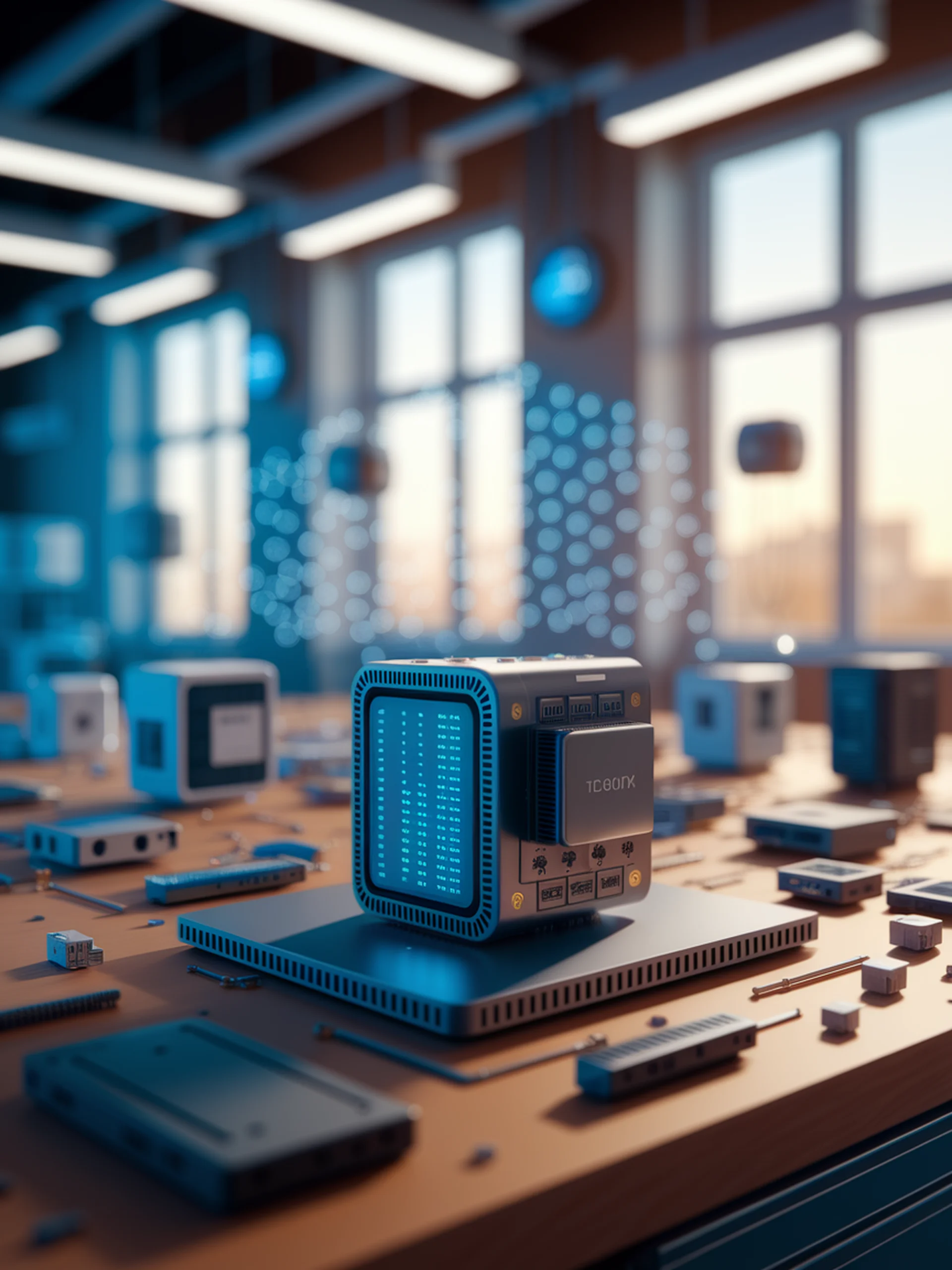
Bringing LLM Power to IoT Edge Devices
Fine-tuned small language models for specialized IoT development
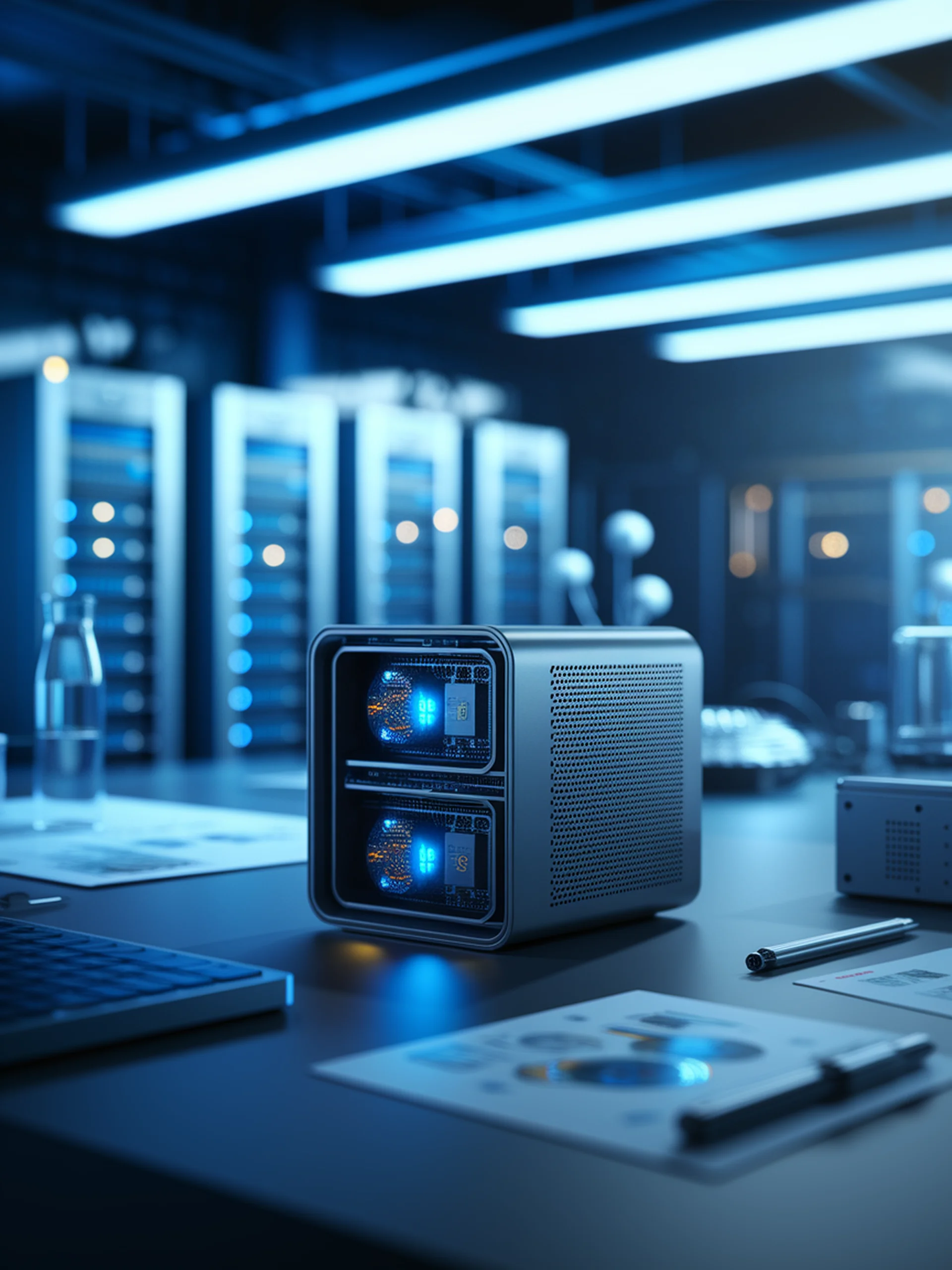
Optimizing LLMs for Edge Computing
Balancing performance and resource constraints at the network edge
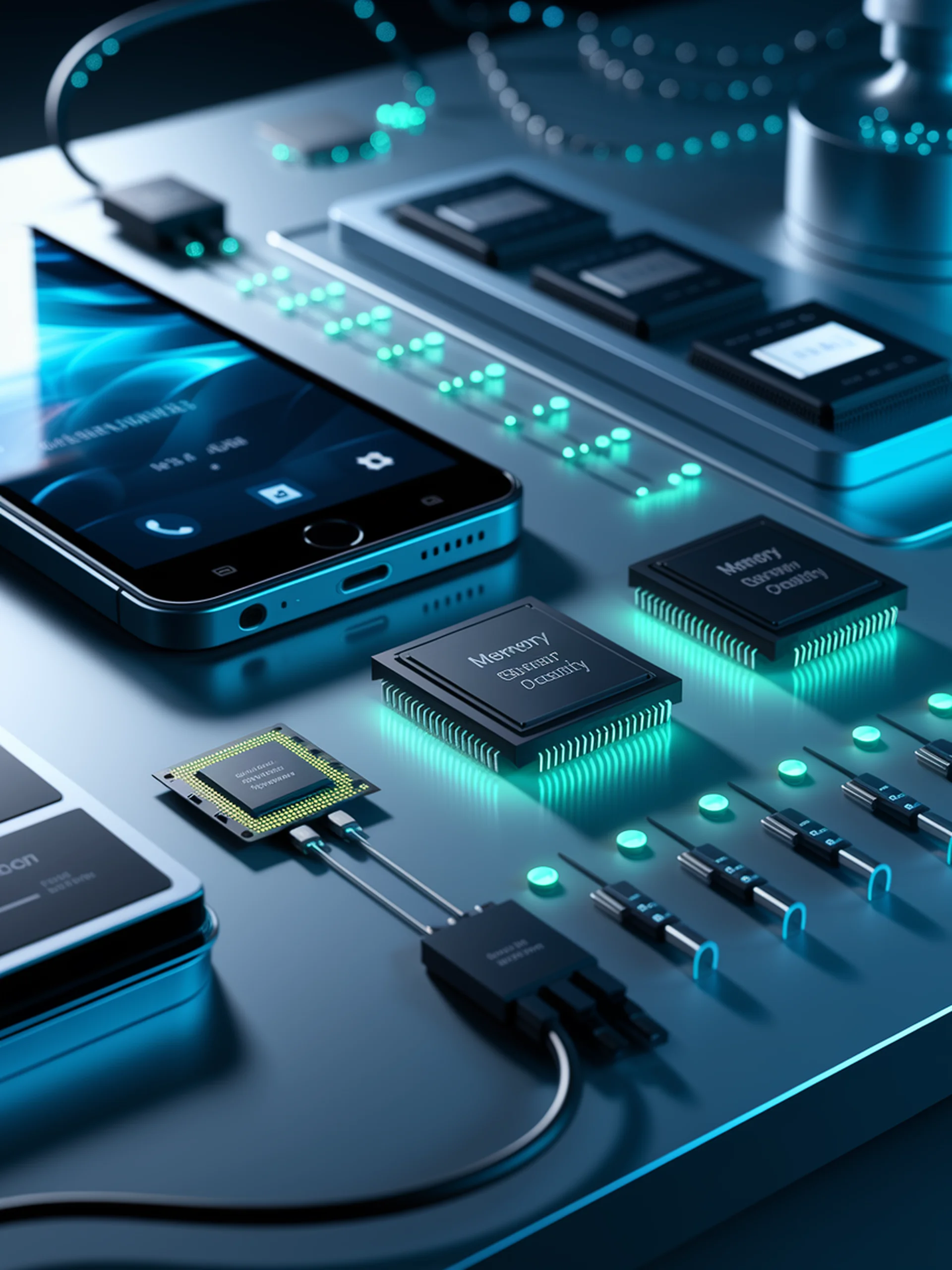
FlexInfer: Breaking Device Memory Barriers
Enabling efficient LLM inference on resource-constrained devices
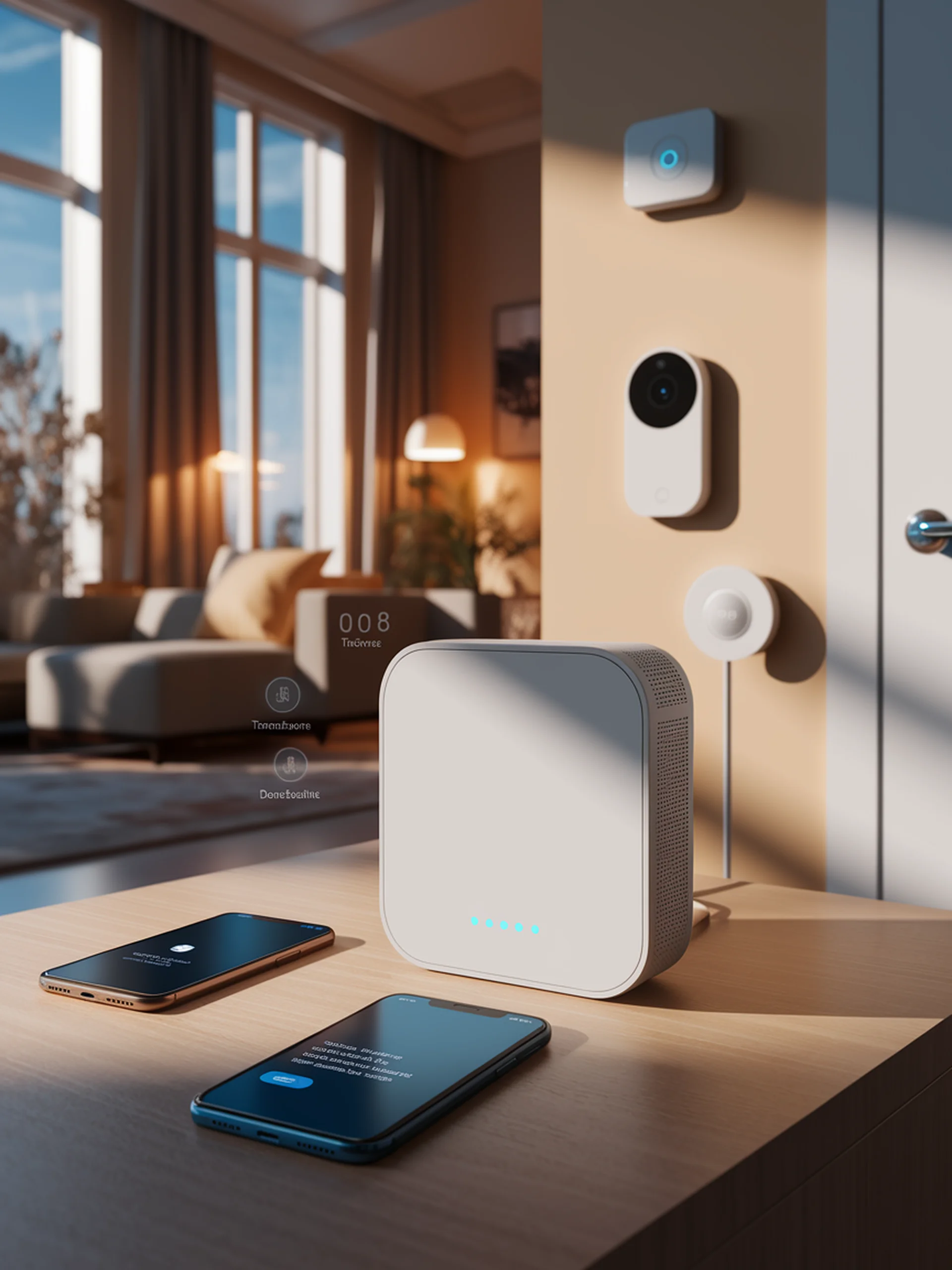
Automating IoT Development with LLMs
Natural language programming for secure AIoT applications
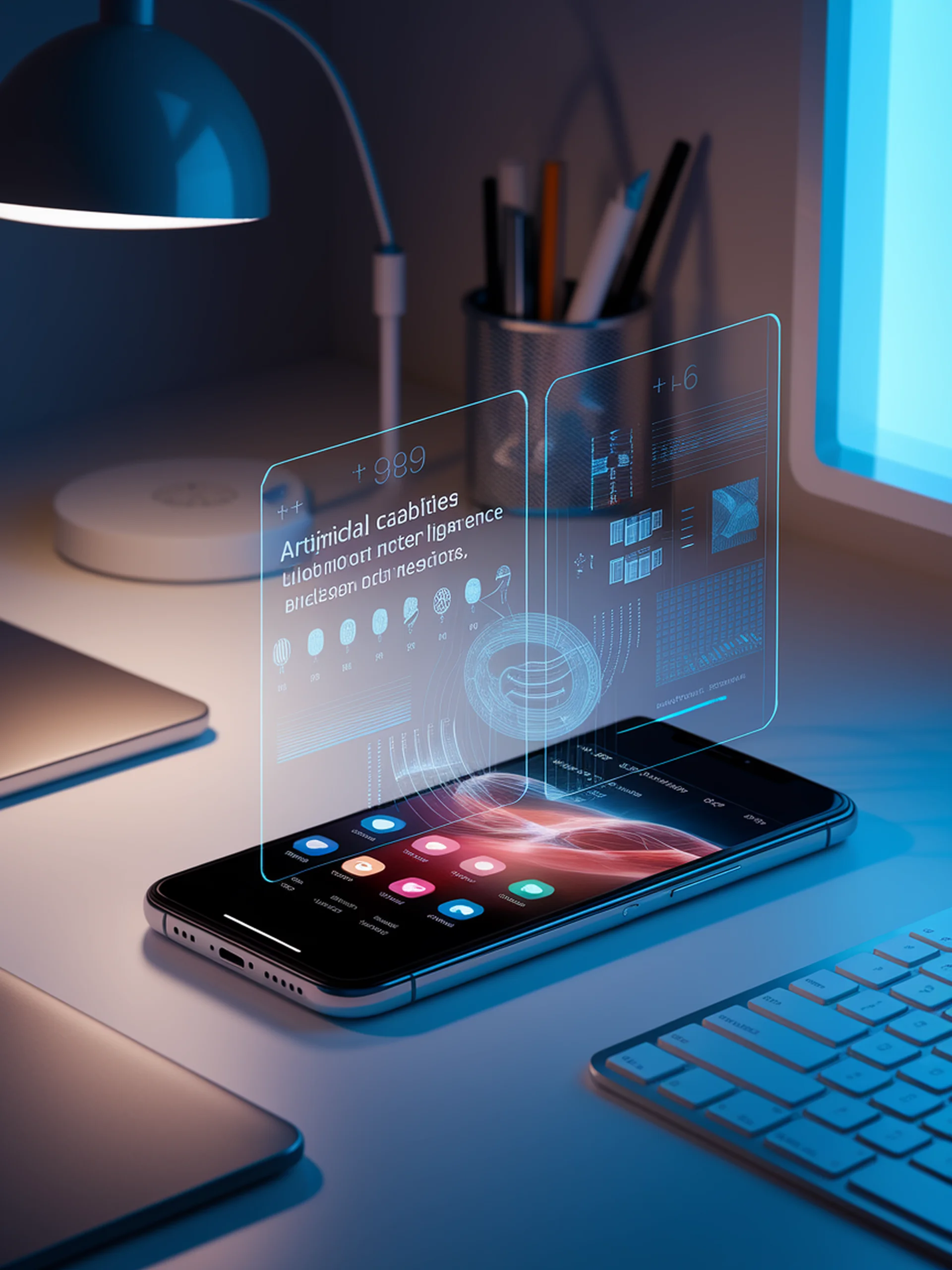
GenieBlue: Mobile-Optimized Multimodal AI
Enhancing On-Device Language Models Without Sacrificing Capabilities
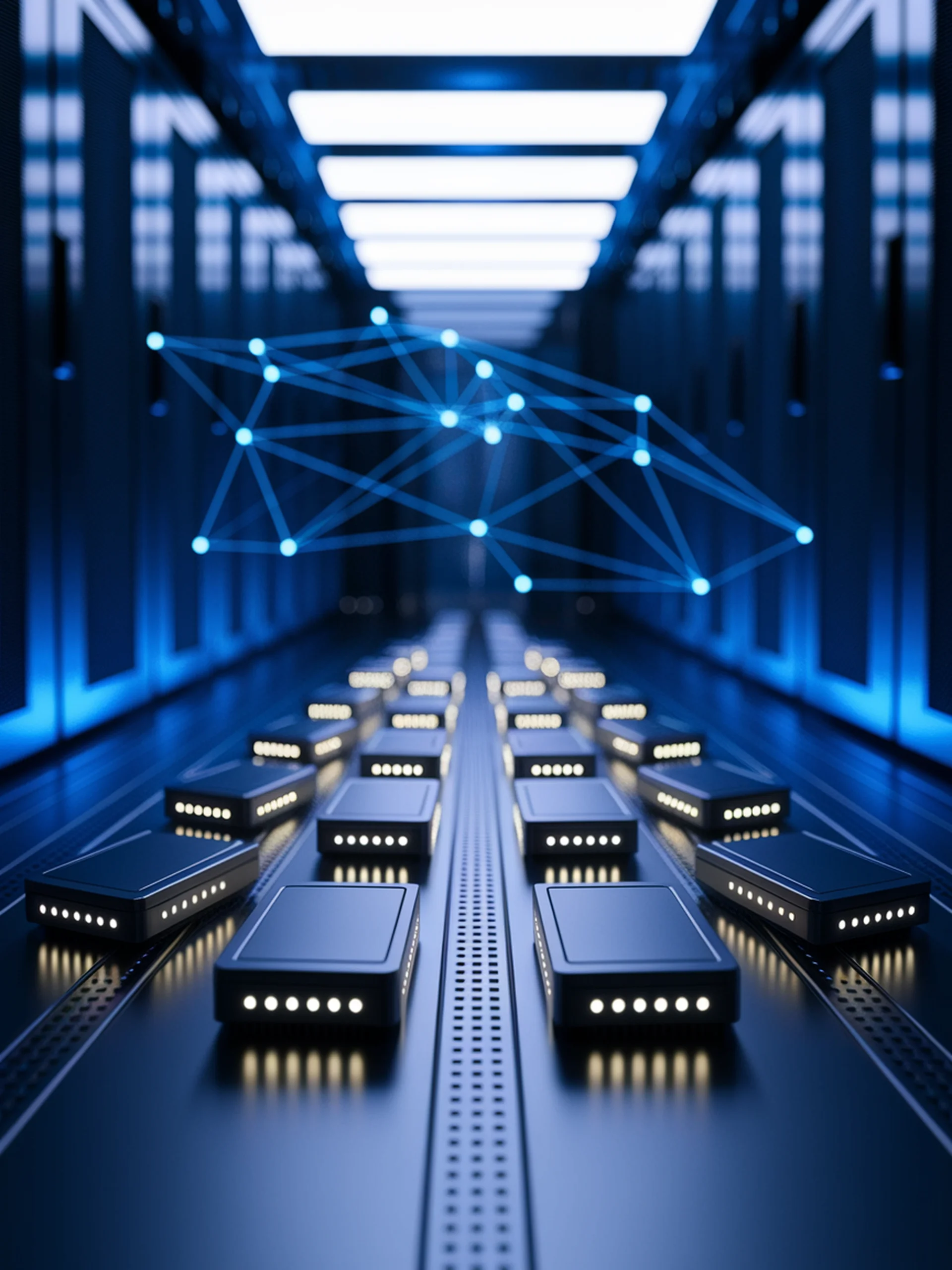
Making LLM Fine-Tuning Private & Efficient
Using Layer Dropout to Enhance Federated Learning for LLMs
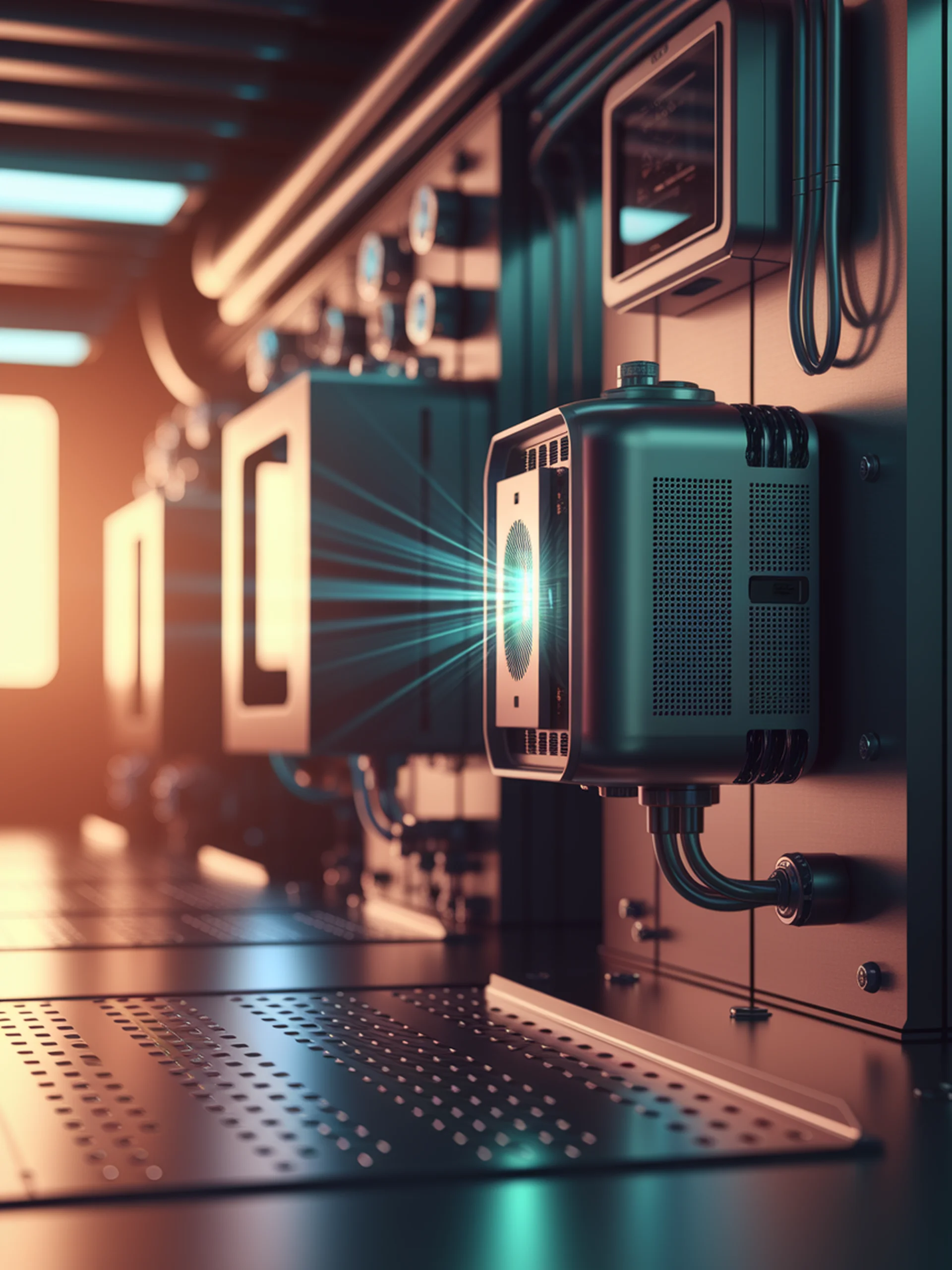
Optimizing LLMs for Edge Devices
Novel memory-efficient techniques for low-power environments
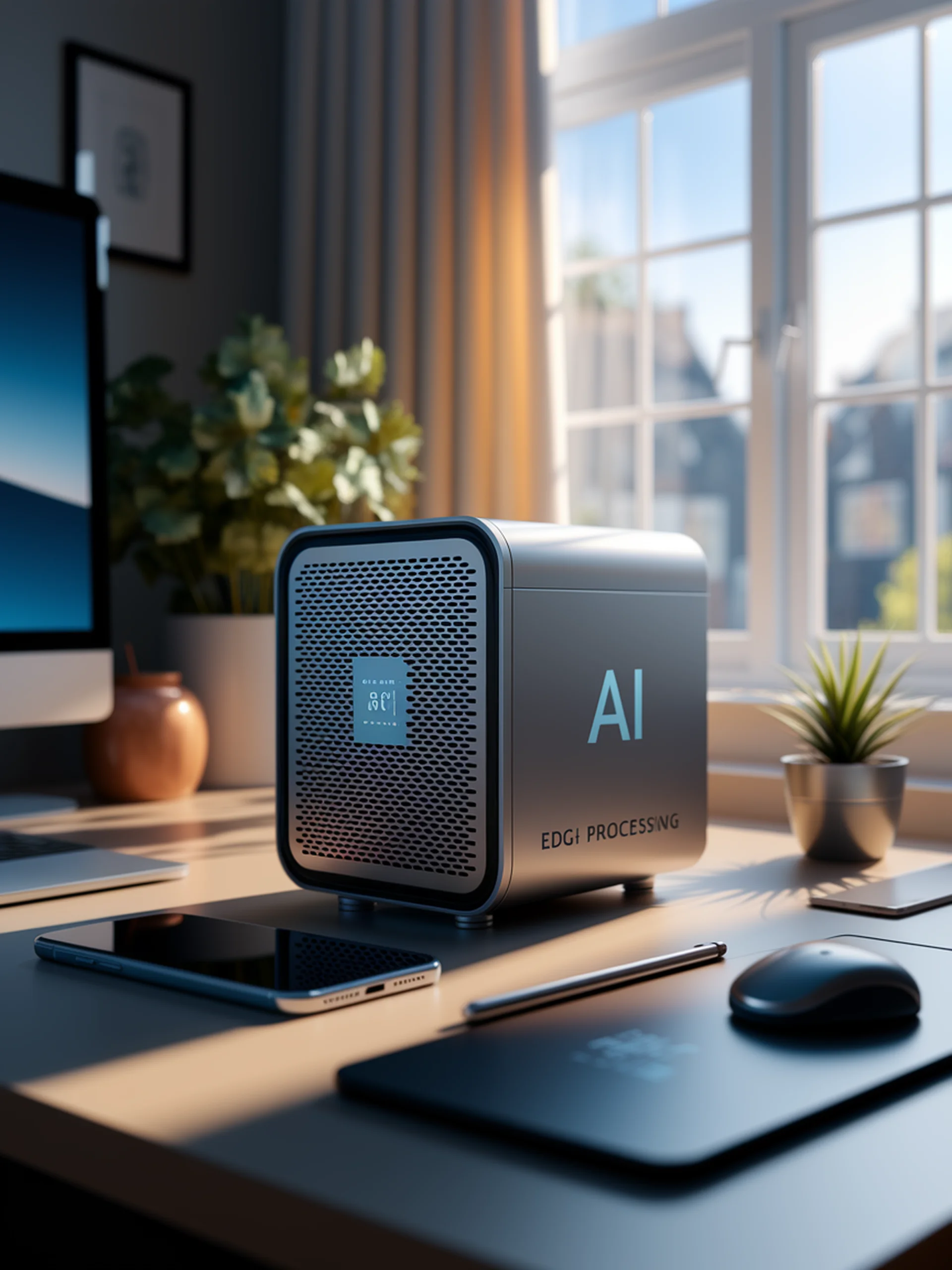
Edge-Optimized Language Models
Hardware-co-designed PLMs for resource-constrained devices
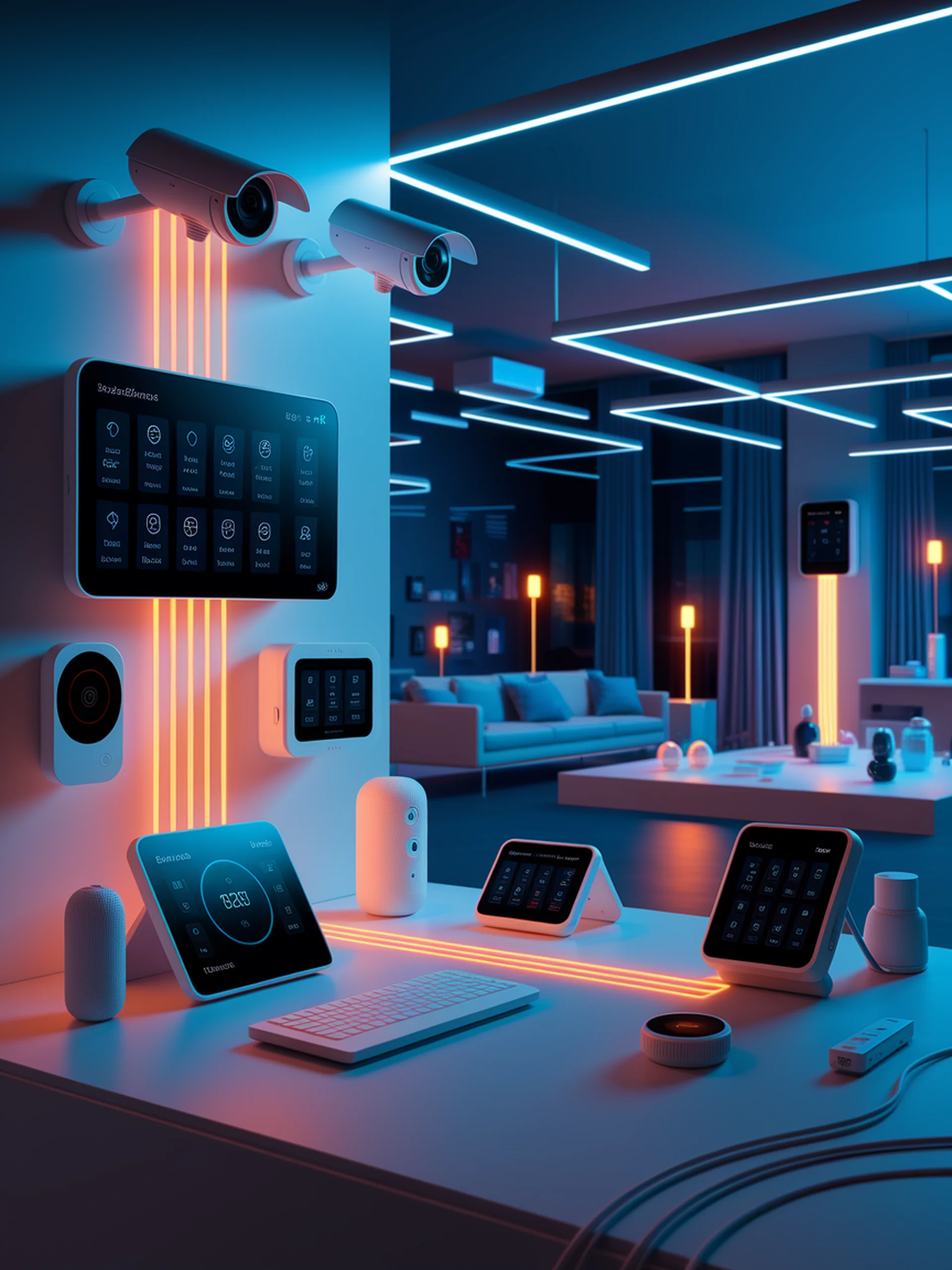
Intelligent Search for IoT Ecosystems
Unifying fragmented IoT data through agentic search capabilities
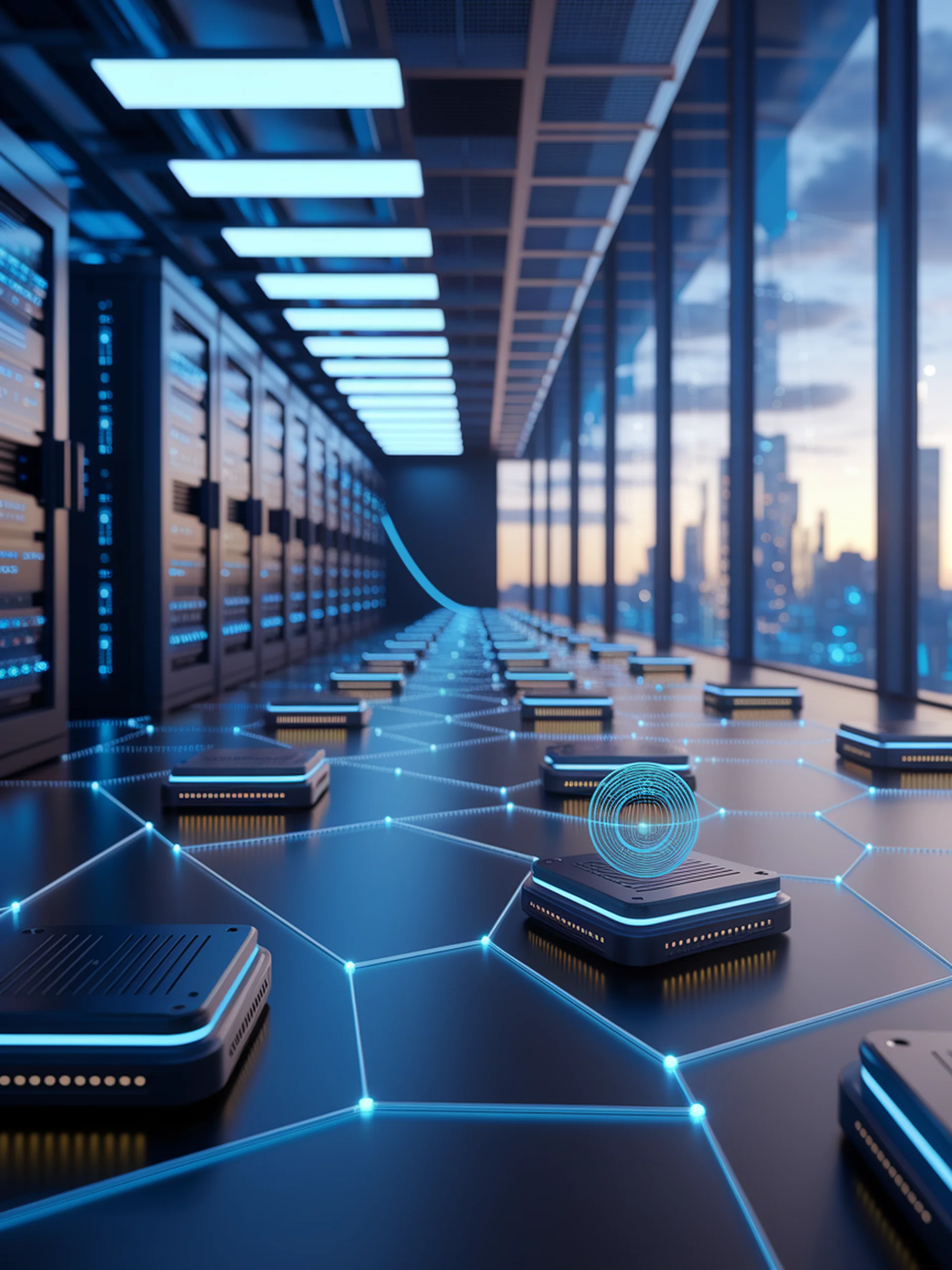
Intelligent IoT: The LLM Revolution for 6G Networks
A new architecture for autonomous, intelligent IoT systems
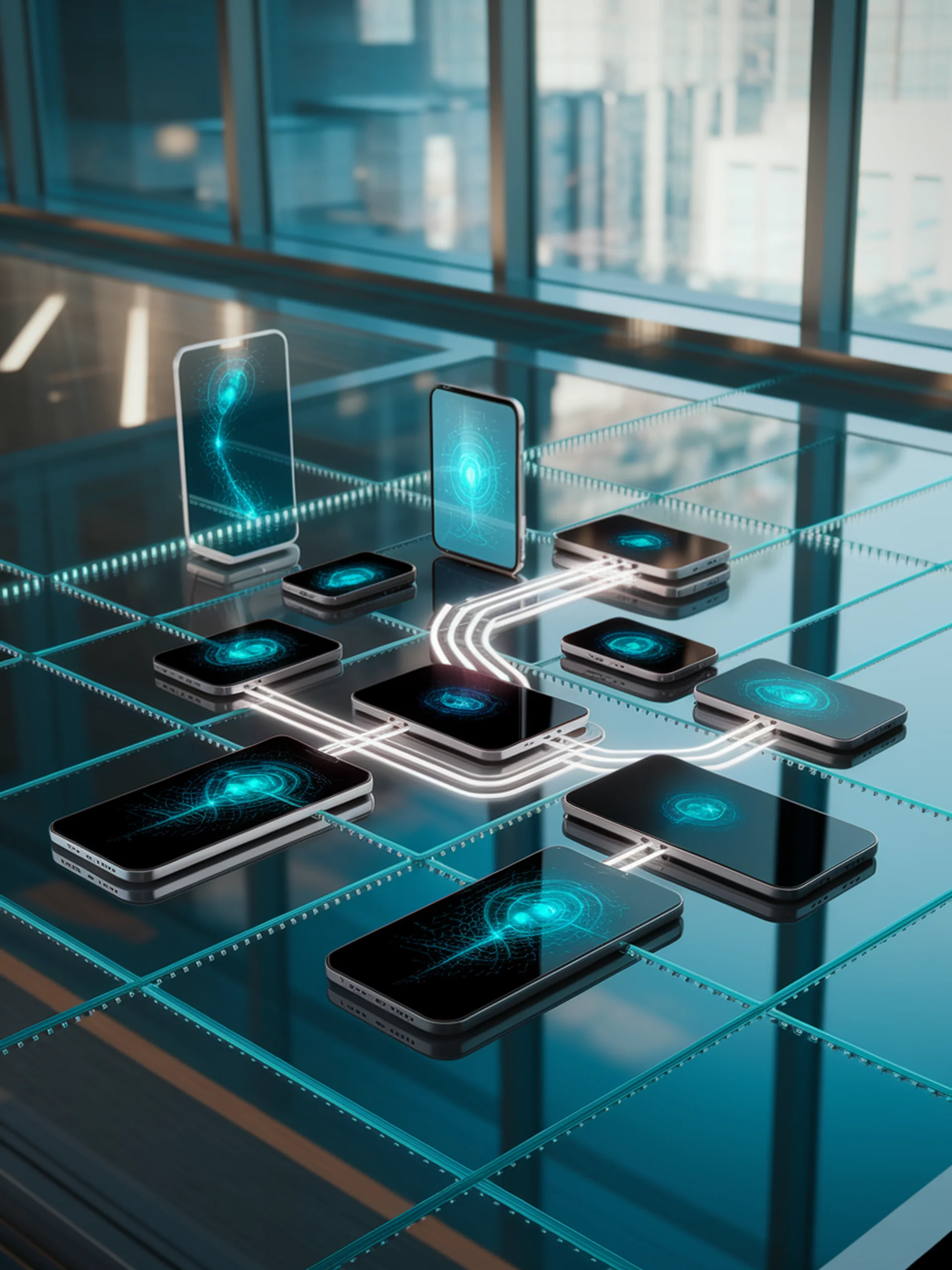
Collaborative LLM Inference at the Edge
Distributing LLM workloads across multiple devices for efficient processing
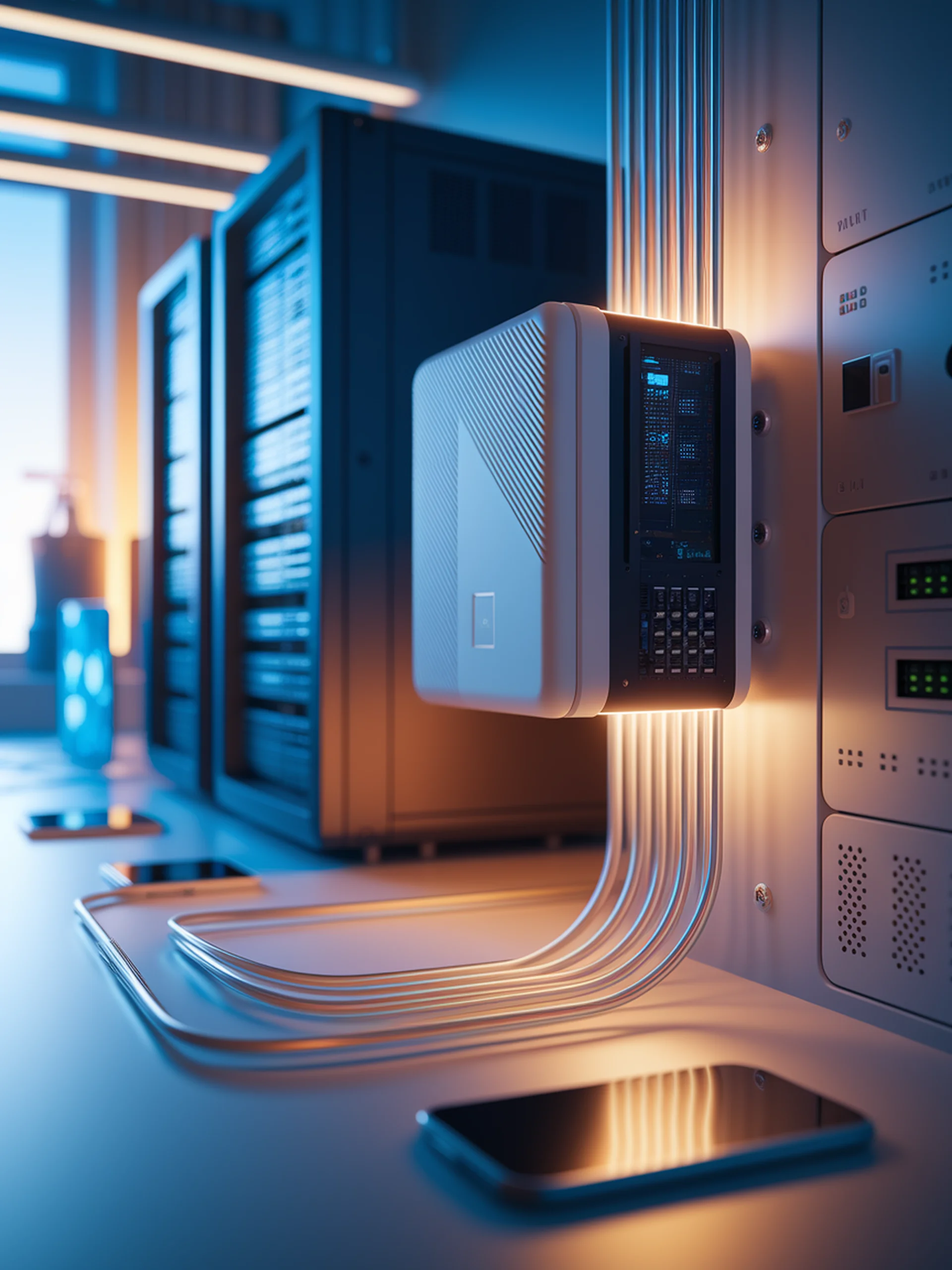
SplitFrozen: Making LLMs Work on Edge Devices
Efficient fine-tuning for resource-constrained environments
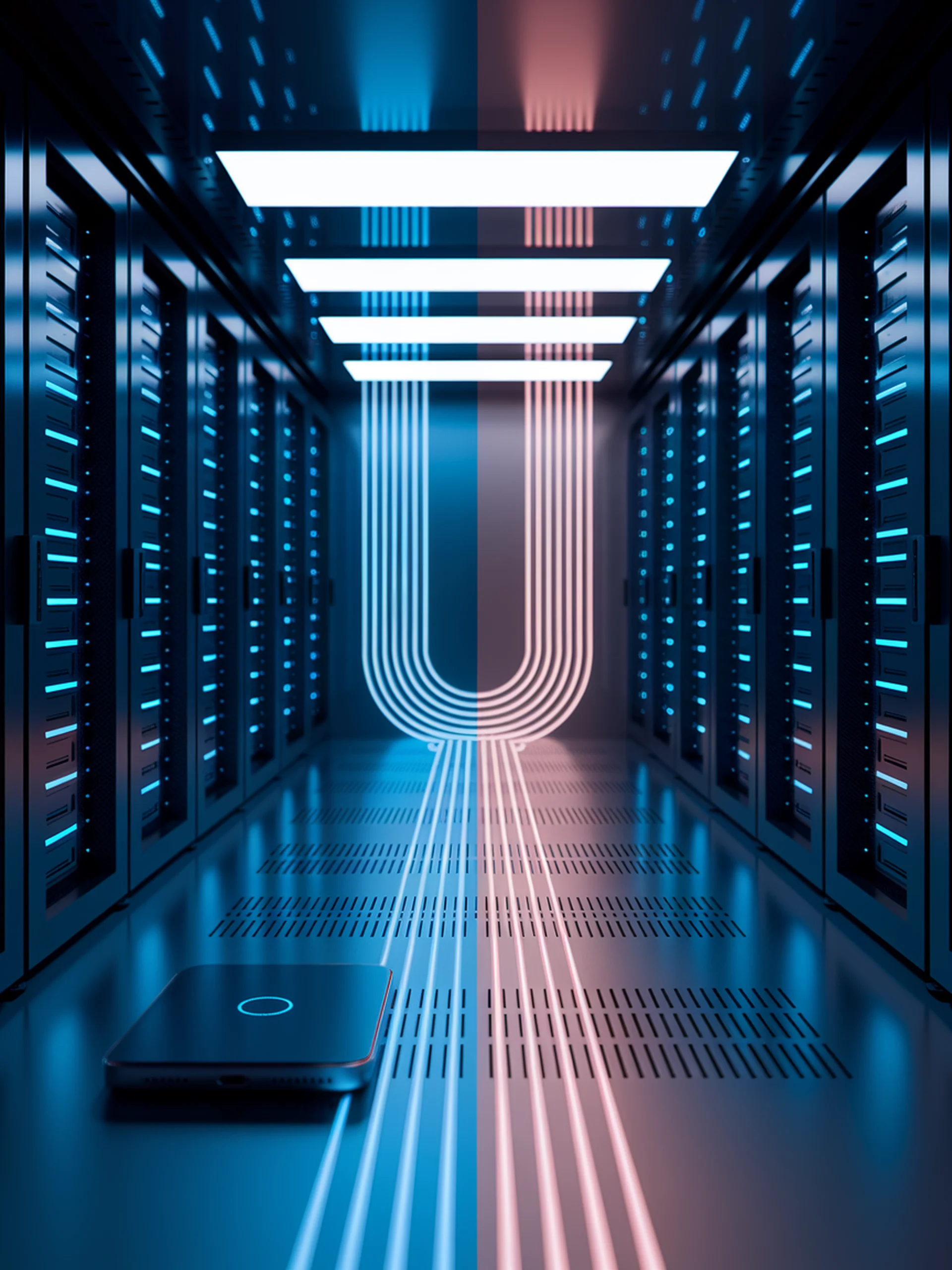
HAT: Rethinking LLM Deployment
A Device-Cloud Collaborative Framework for Faster, More Private LLMs

Breaking Memory Barriers for AI on Edge Devices
A solution for infinite context windows on resource-constrained hardware
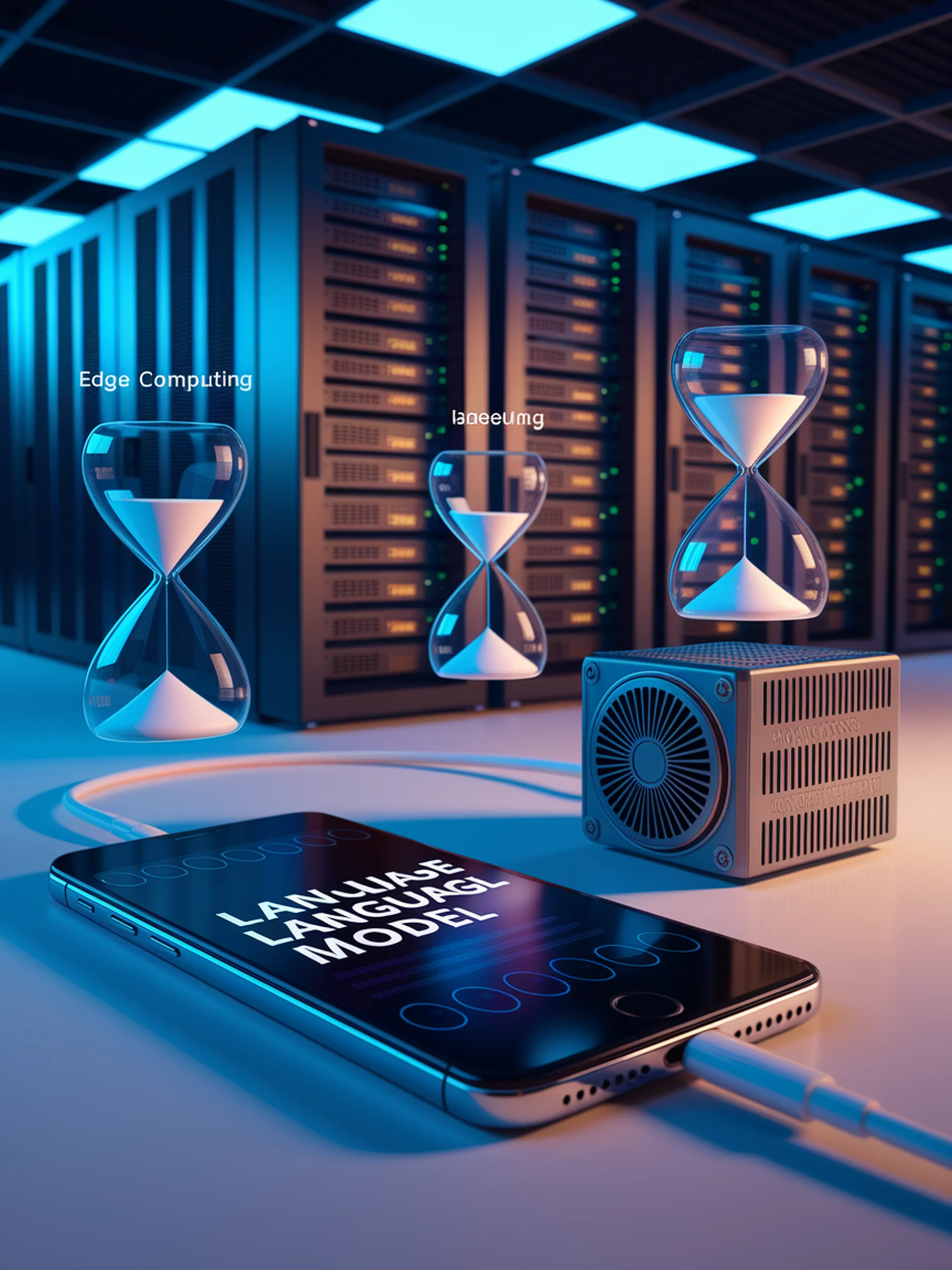
Deploying LLMs on Mobile: Efficiency Tradeoffs
Measuring performance across mobile, edge, and cloud deployments

Optimizing LLMs for Edge Devices
Enabling high-throughput language models on resource-constrained hardware
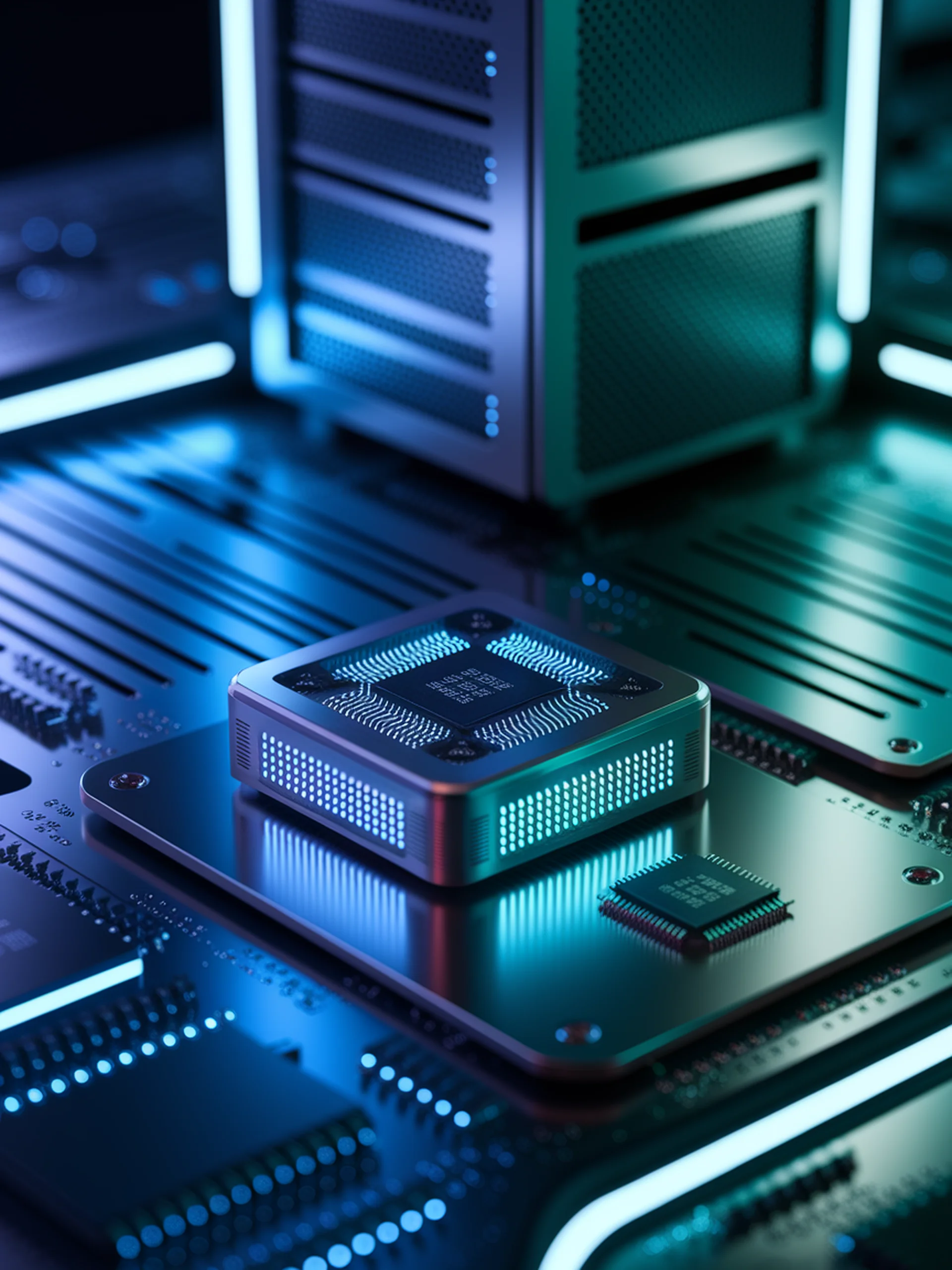
Optimizing LLMs for Edge Devices
Quantization strategies for efficient AI at the edge
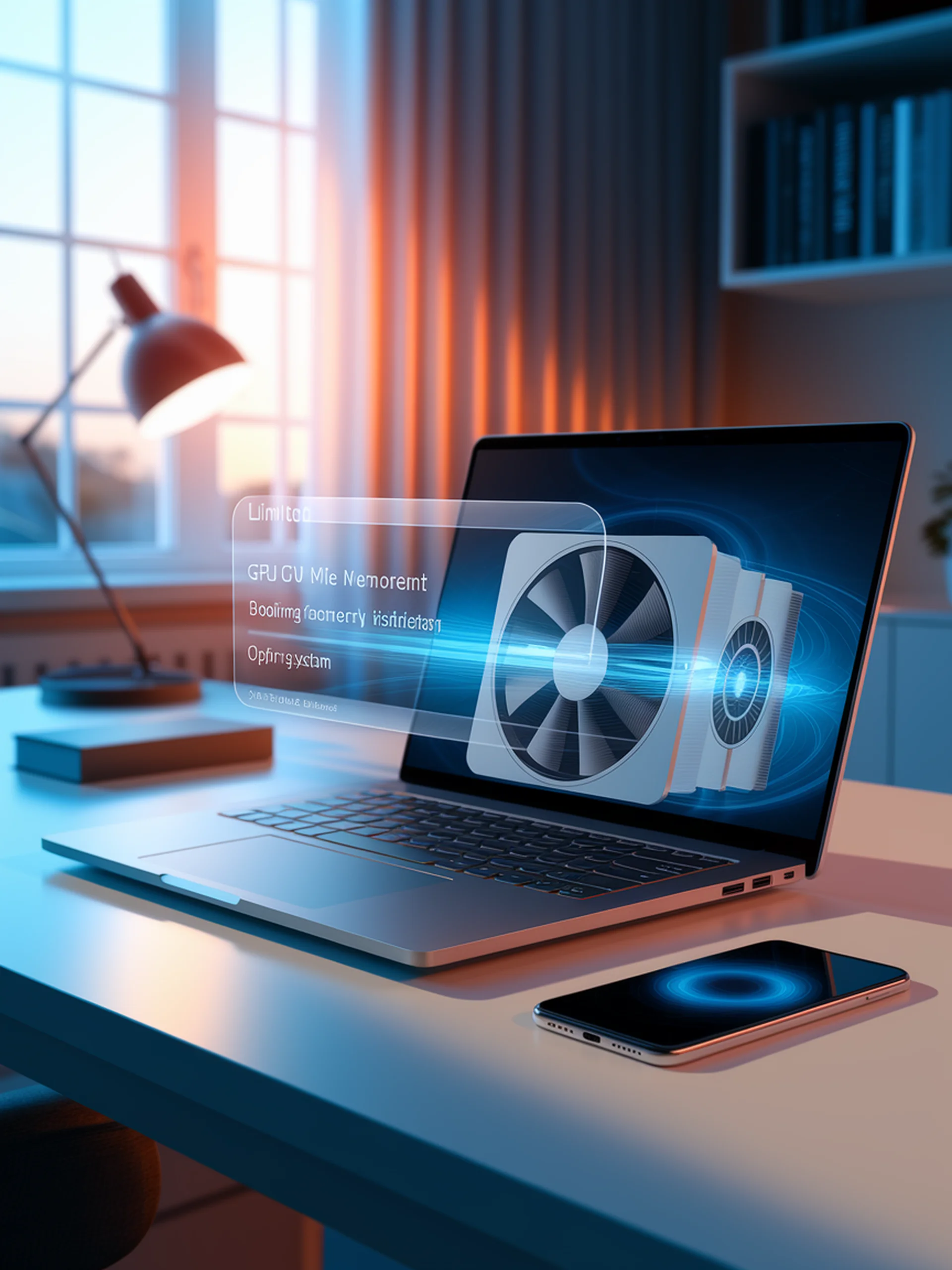
Accelerating LLMs on Consumer Devices
Pipelined Offloading for Efficient Inference with Limited GPU Memory
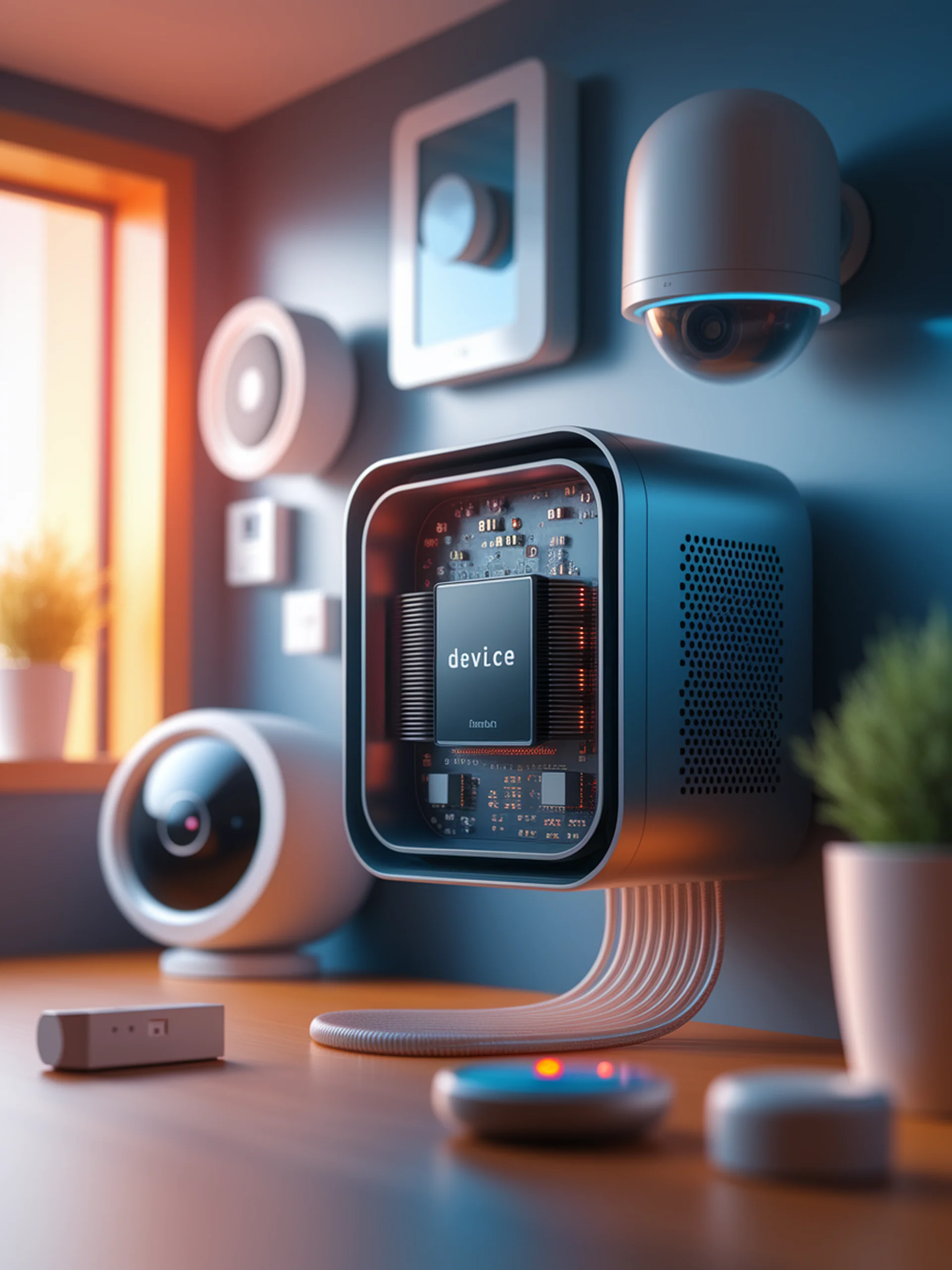
TaskEdge: Bringing LLMs to Resource-Constrained Devices
Smart Fine-Tuning for Edge Computing
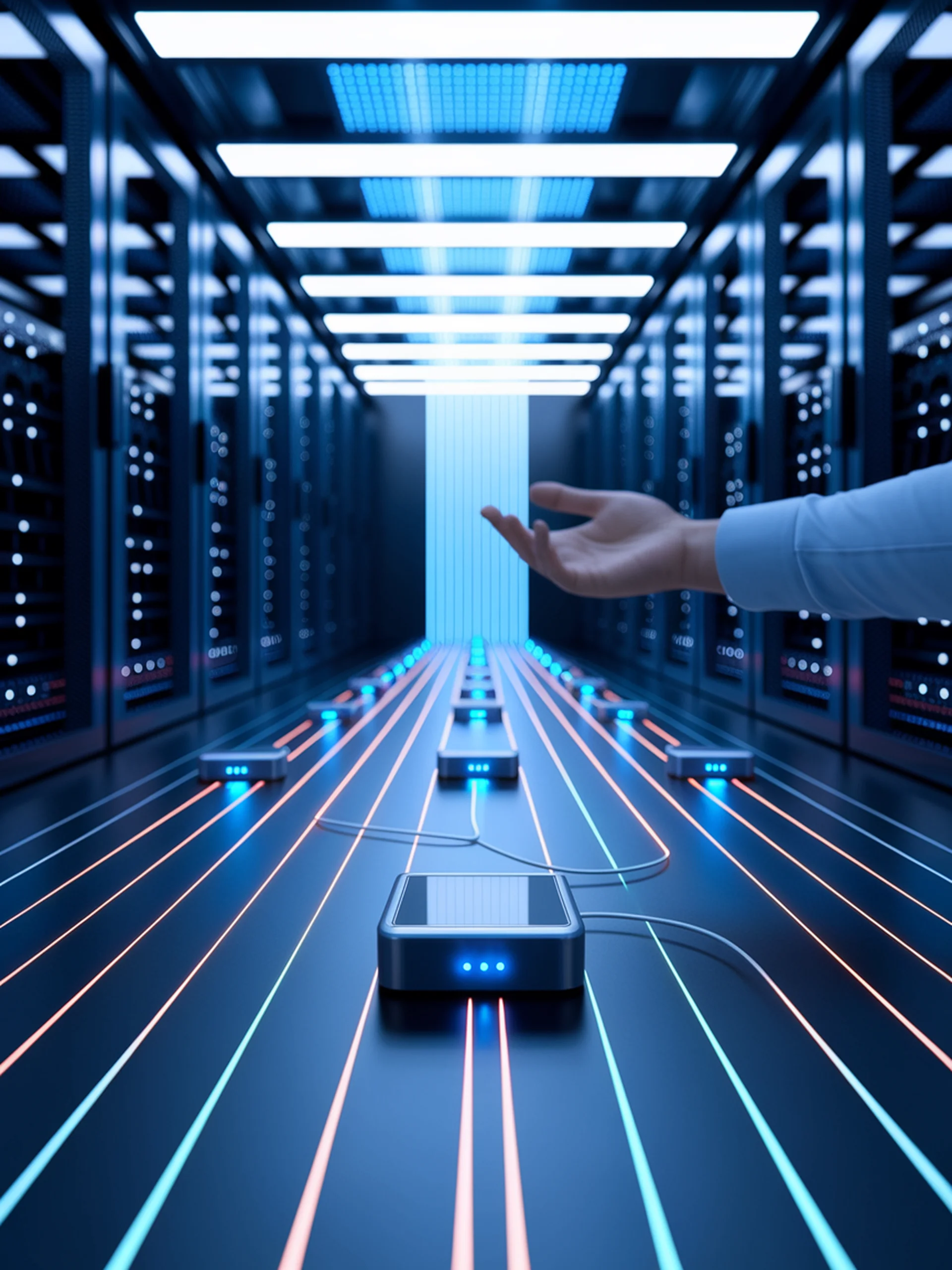
Intent-Driven Computing Across Devices
Leveraging LLMs for Intelligent Resource Management in Distributed Systems
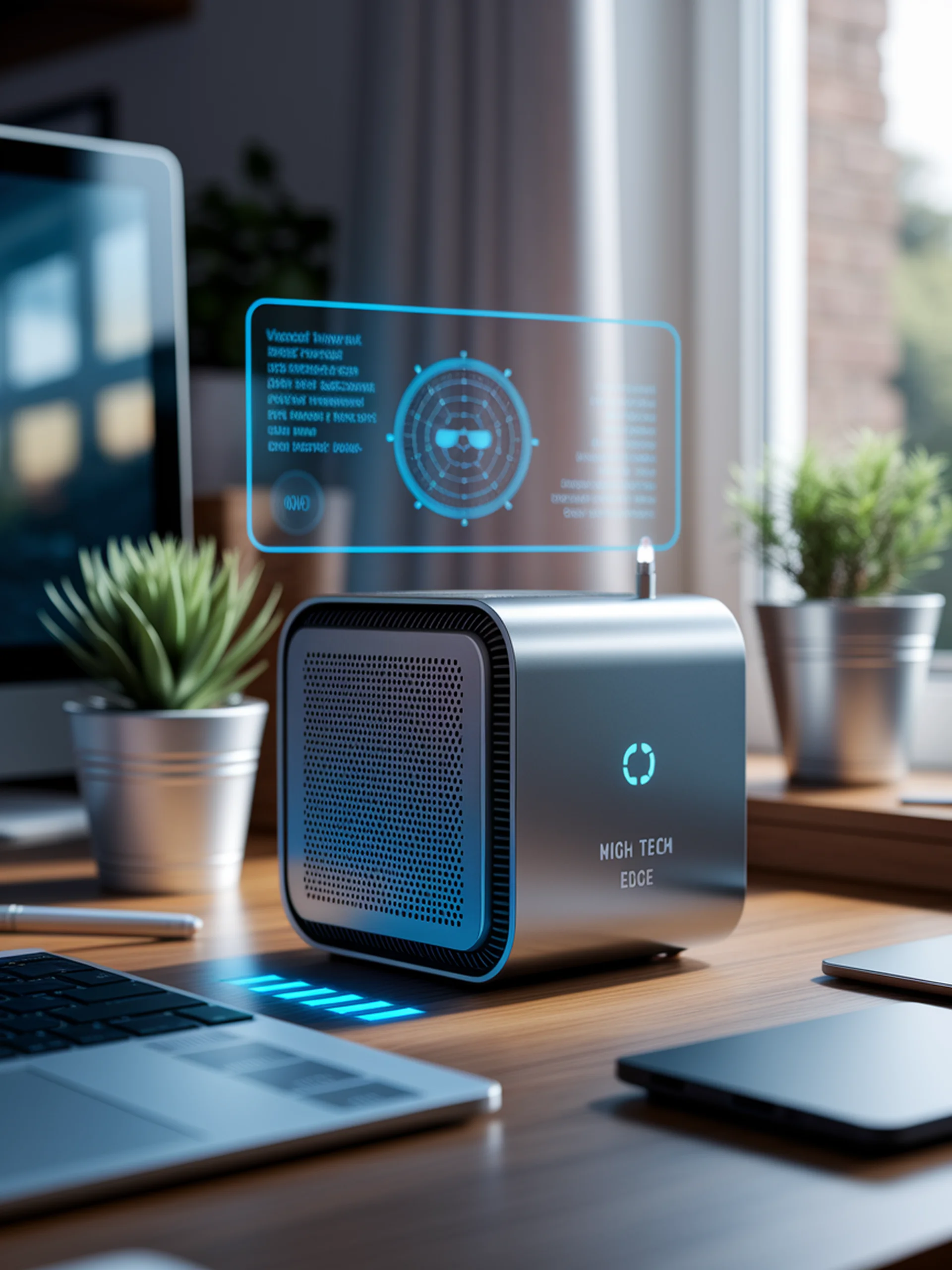
SmolVLM: Efficient Vision-Language Models
Optimizing multimodal AI for resource-constrained environments
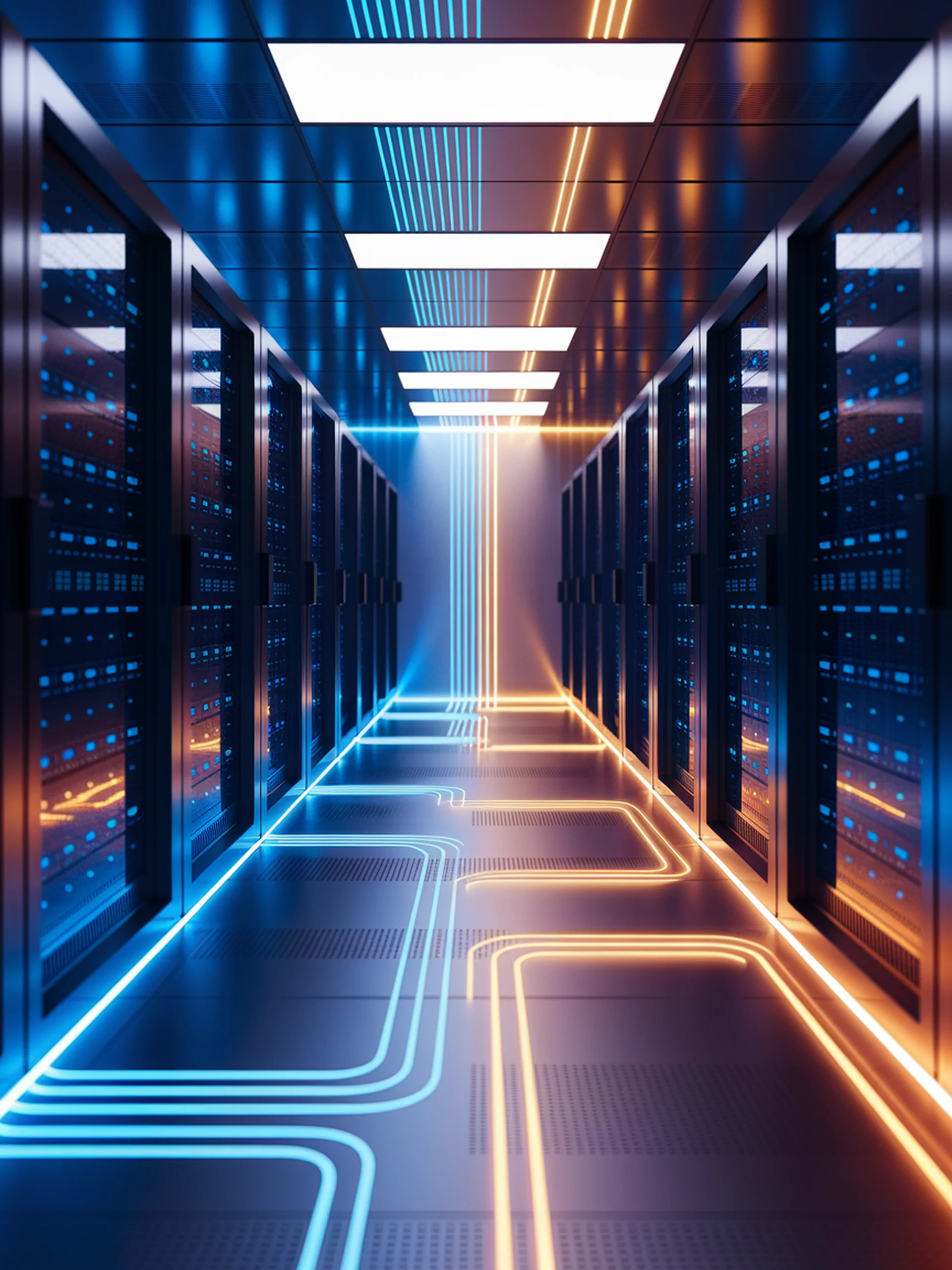
Hybrid LLM Systems for Faster, Smarter Inference
Optimizing AI Decision-Making with Threshold-Based Control
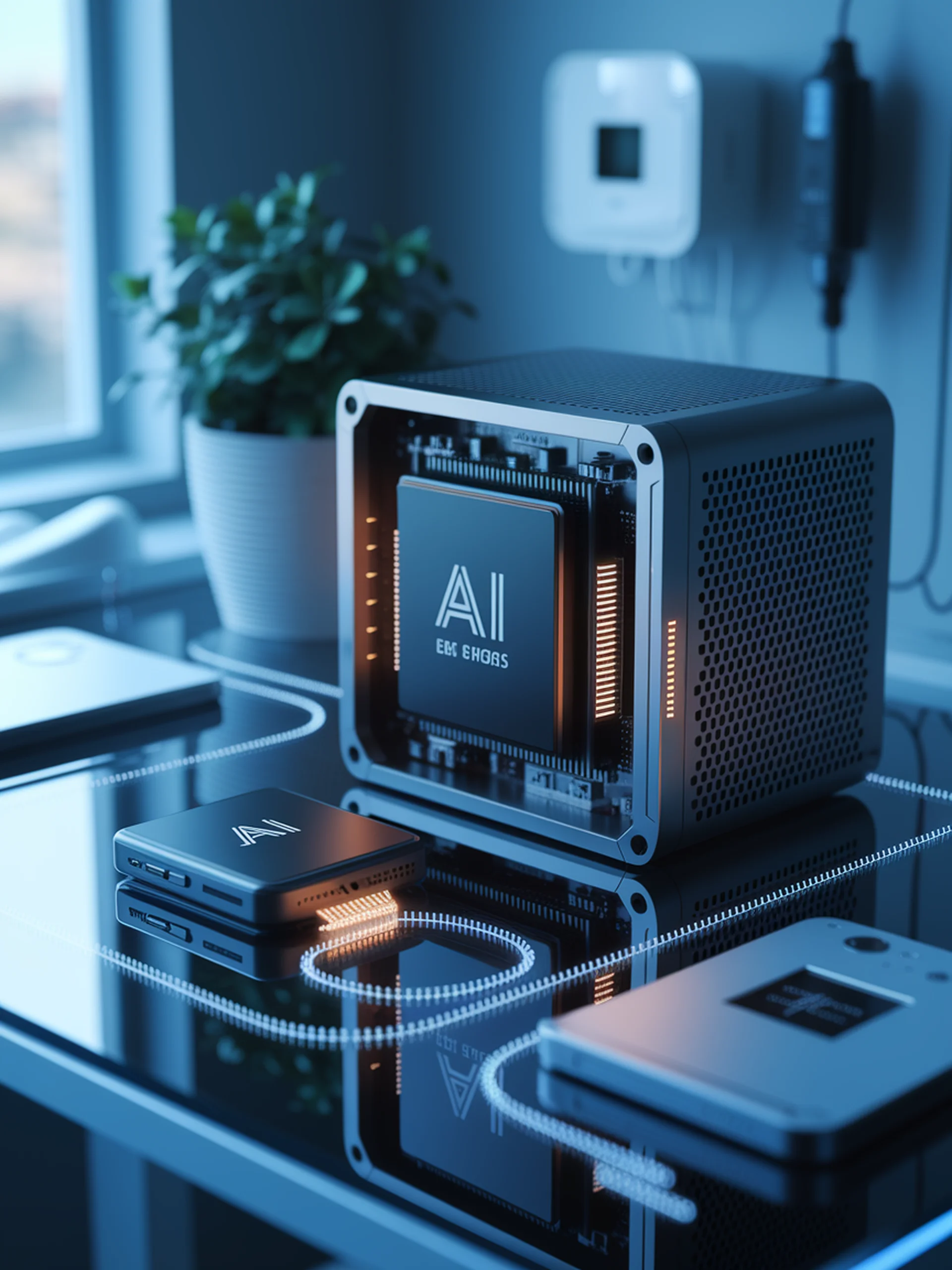
Smart Token Routing for Edge AI
Optimizing LLM inference on resource-constrained devices
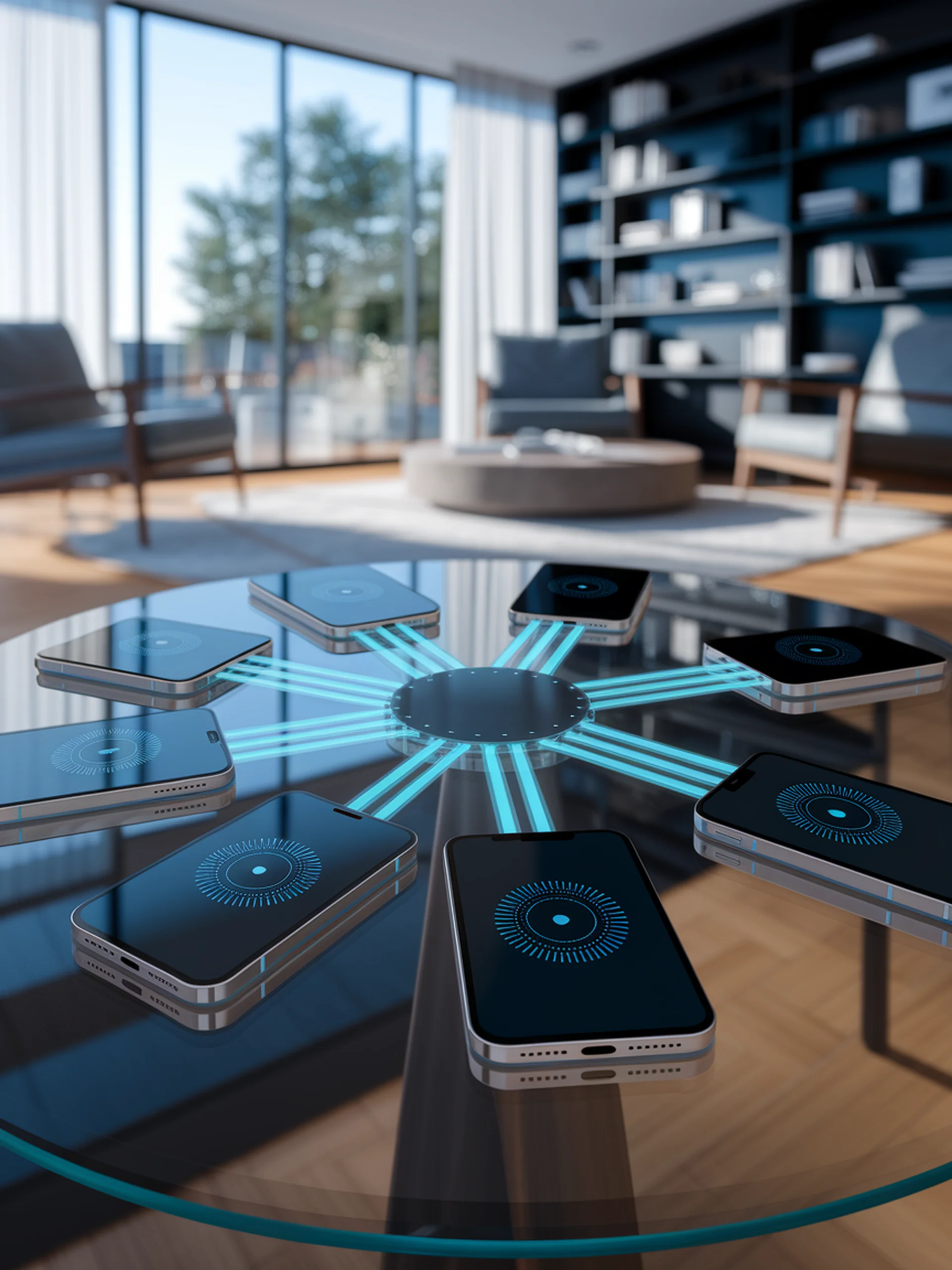
Jupiter: Fast LLM Collaboration at the Edge
Enabling efficient LLM inference across resource-constrained devices
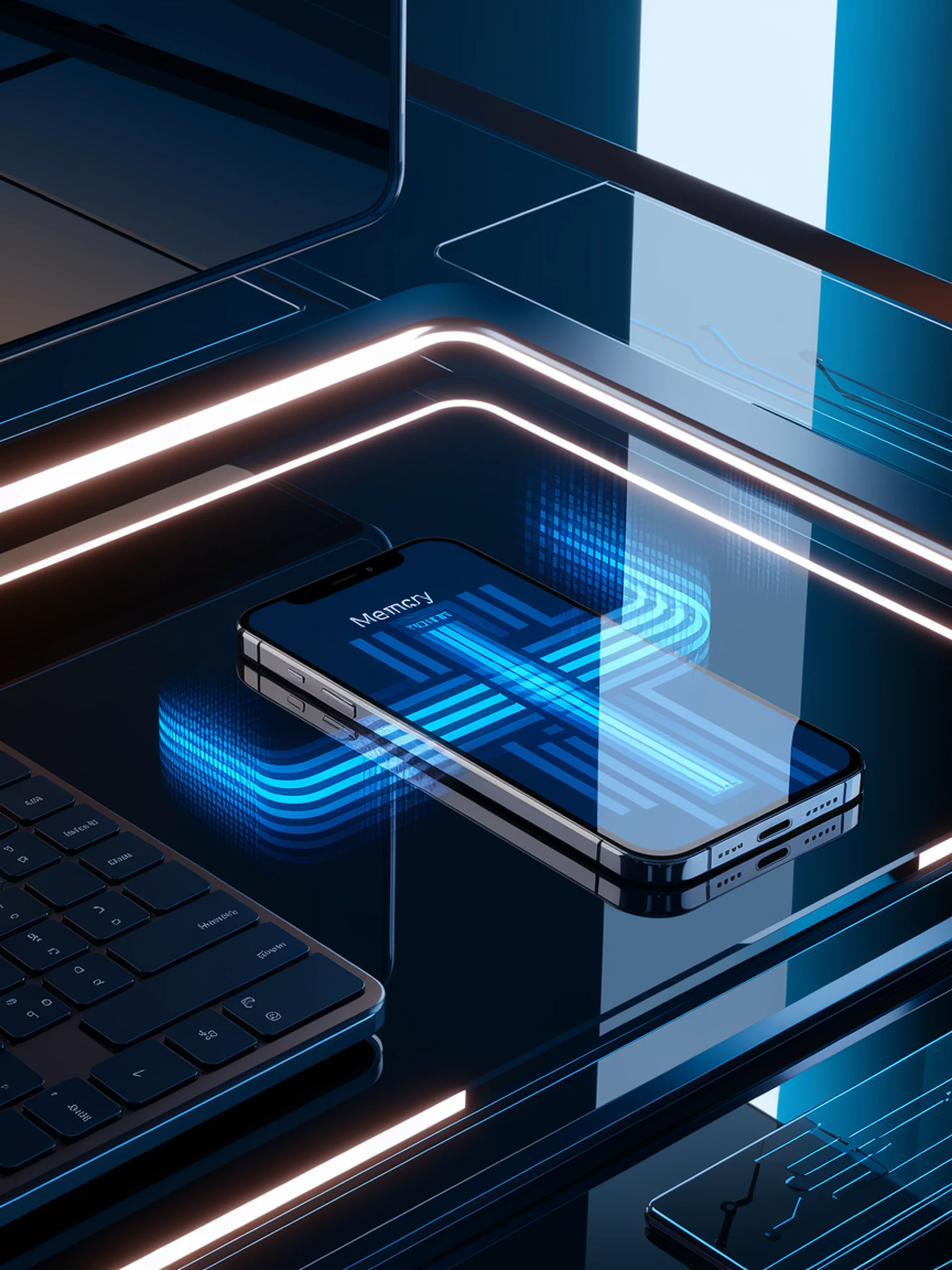
Breaking Memory Barriers for Mobile LLMs
Enabling larger language models on memory-constrained devices
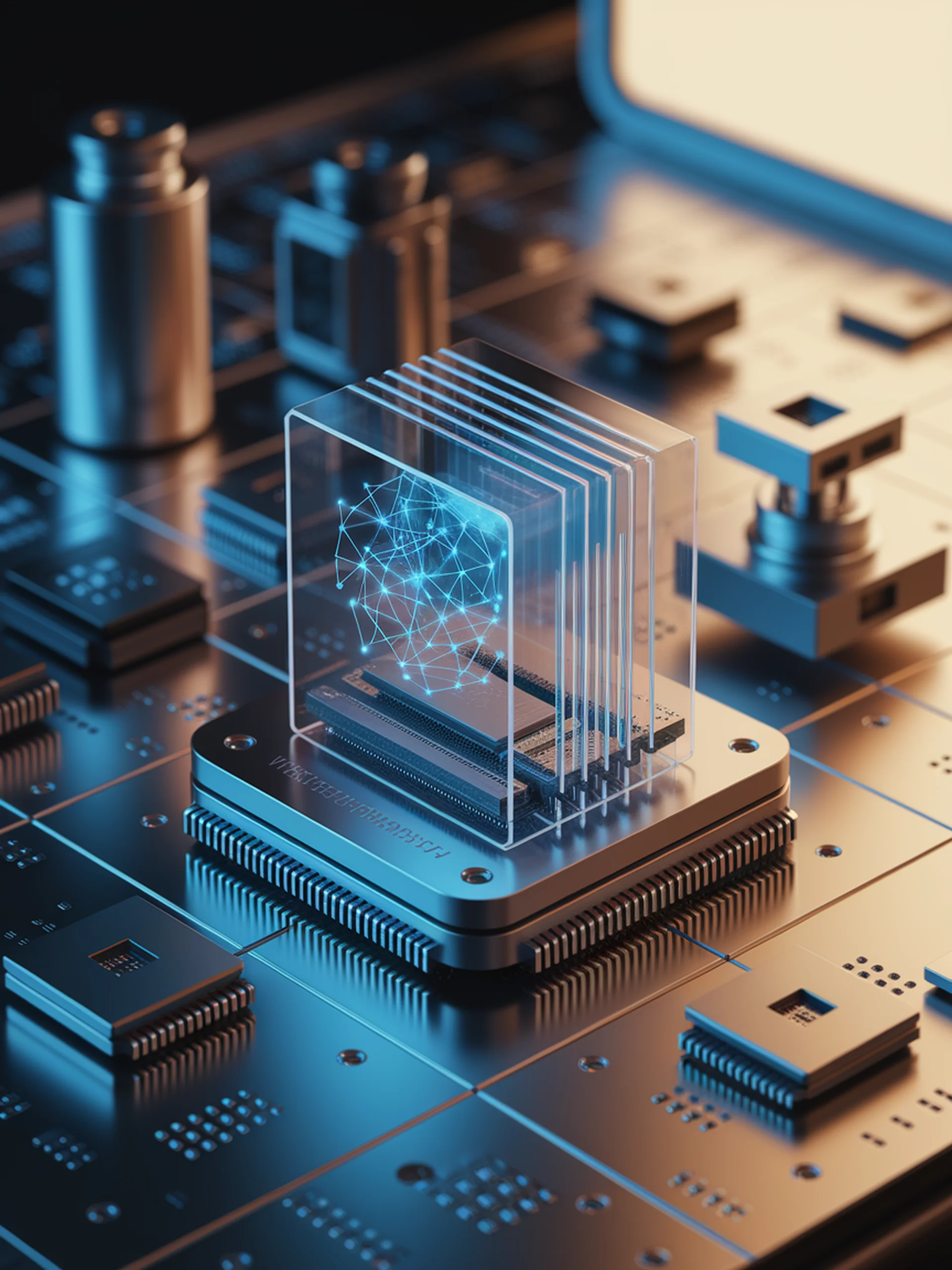
Revolutionizing TinyML with LLMs
A novel framework for efficient, explainable edge AI
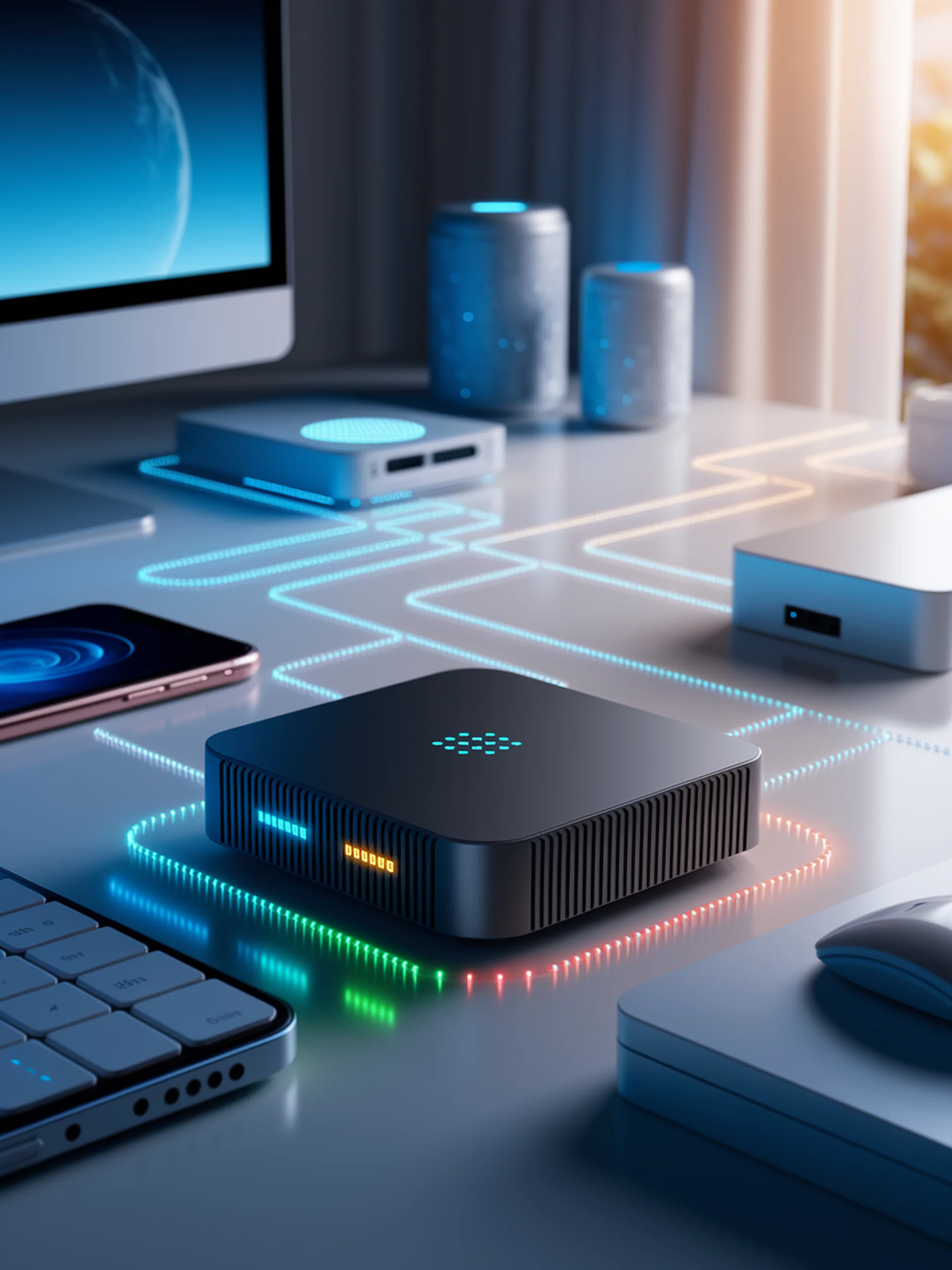