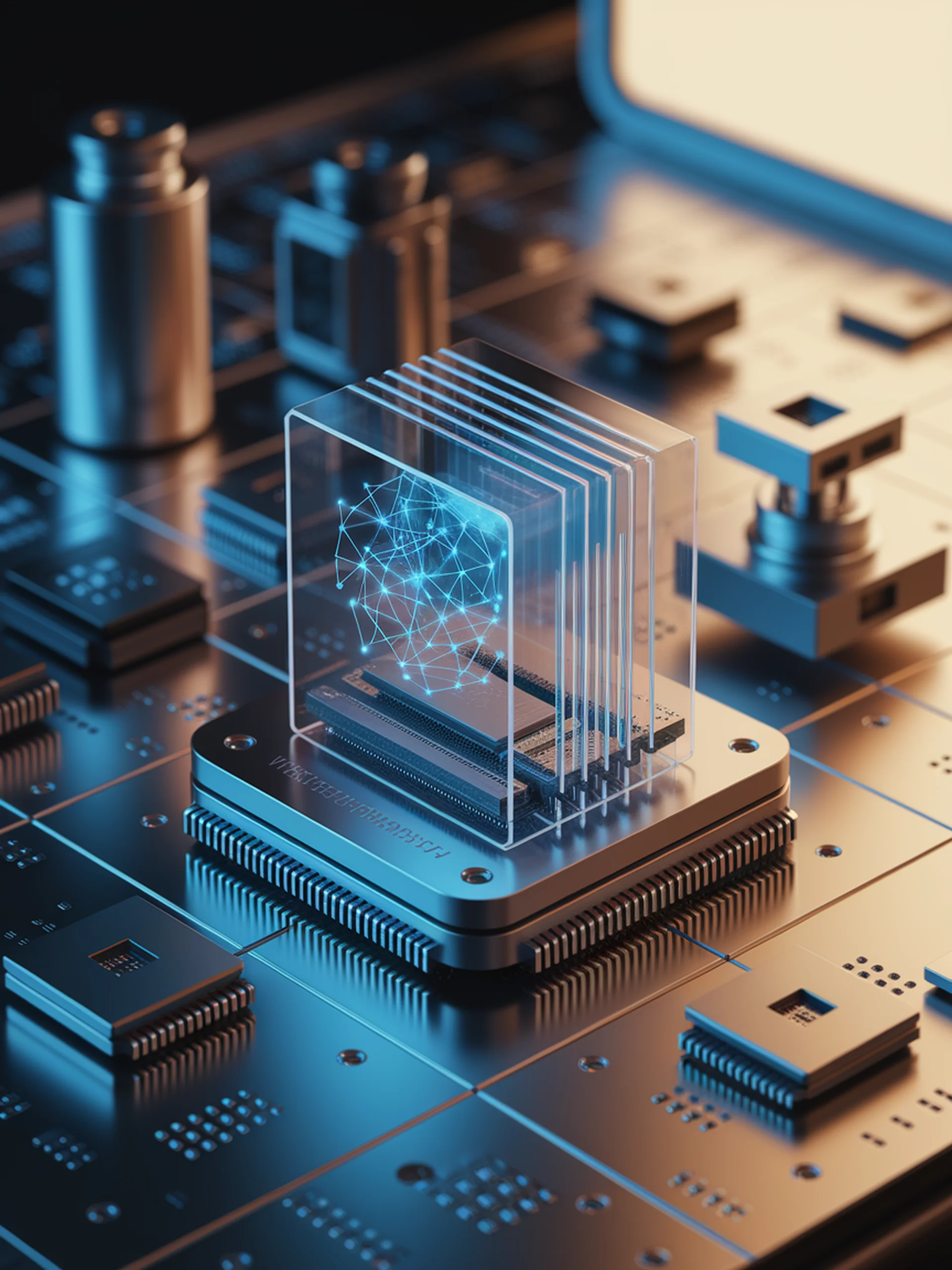
Revolutionizing TinyML with LLMs
A novel framework for efficient, explainable edge AI
This research introduces a groundbreaking approach that uses Large Language Models (LLMs) to design optimized neural networks for resource-constrained devices.
- Combines LLM-guided neural architecture search with Vision Transformer knowledge distillation
- Achieves superior balance between accuracy, computational efficiency, and memory usage
- Incorporates an explainability module to enhance model transparency
- Demonstrates practical applications for Engineering and Security in edge computing environments
This framework represents a significant advancement for Engineering teams deploying AI on tiny devices, enabling more powerful models to run efficiently at the edge while maintaining explainability.
Can LLMs Revolutionize the Design of Explainable and Efficient TinyML Models?