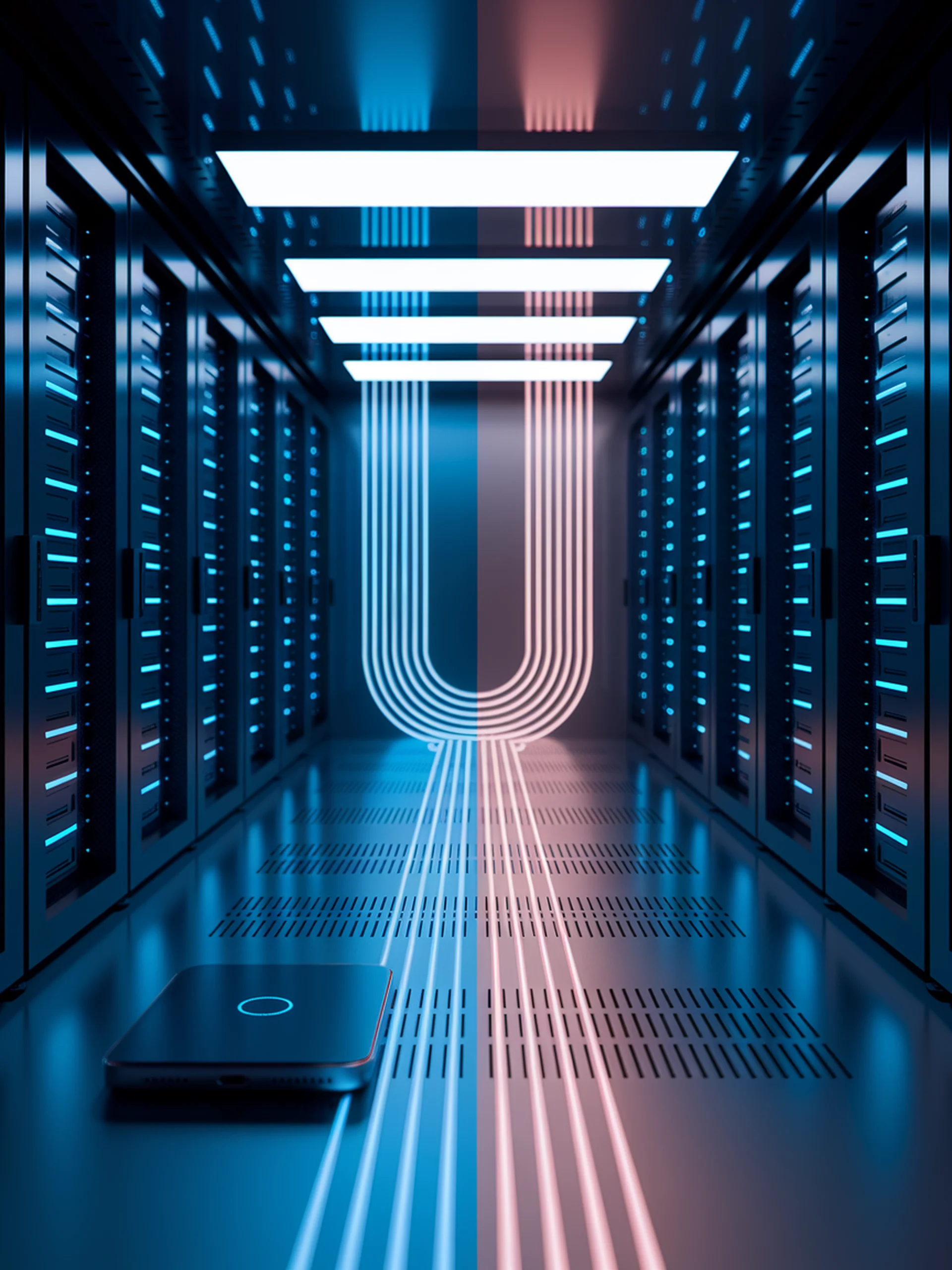
HAT: Rethinking LLM Deployment
A Device-Cloud Collaborative Framework for Faster, More Private LLMs
HAT introduces a novel hybrid inference approach that distributes LLM processing between devices and cloud to overcome limitations of traditional cloud-only implementations.
- Combines U-shaped inference with speculative decoding to optimize performance
- Addresses critical needs for lower latency and enhanced privacy
- Strategically partitions LLM processing across device and cloud resources
- Demonstrates the viability of hybrid architectures for next-generation AI deployment
This engineering innovation matters because it could transform how LLMs are deployed in privacy-sensitive or latency-critical applications, potentially enabling more widespread adoption of large language models in resource-constrained environments.
A Novel Hat-Shaped Device-Cloud Collaborative Inference Framework for Large Language Models