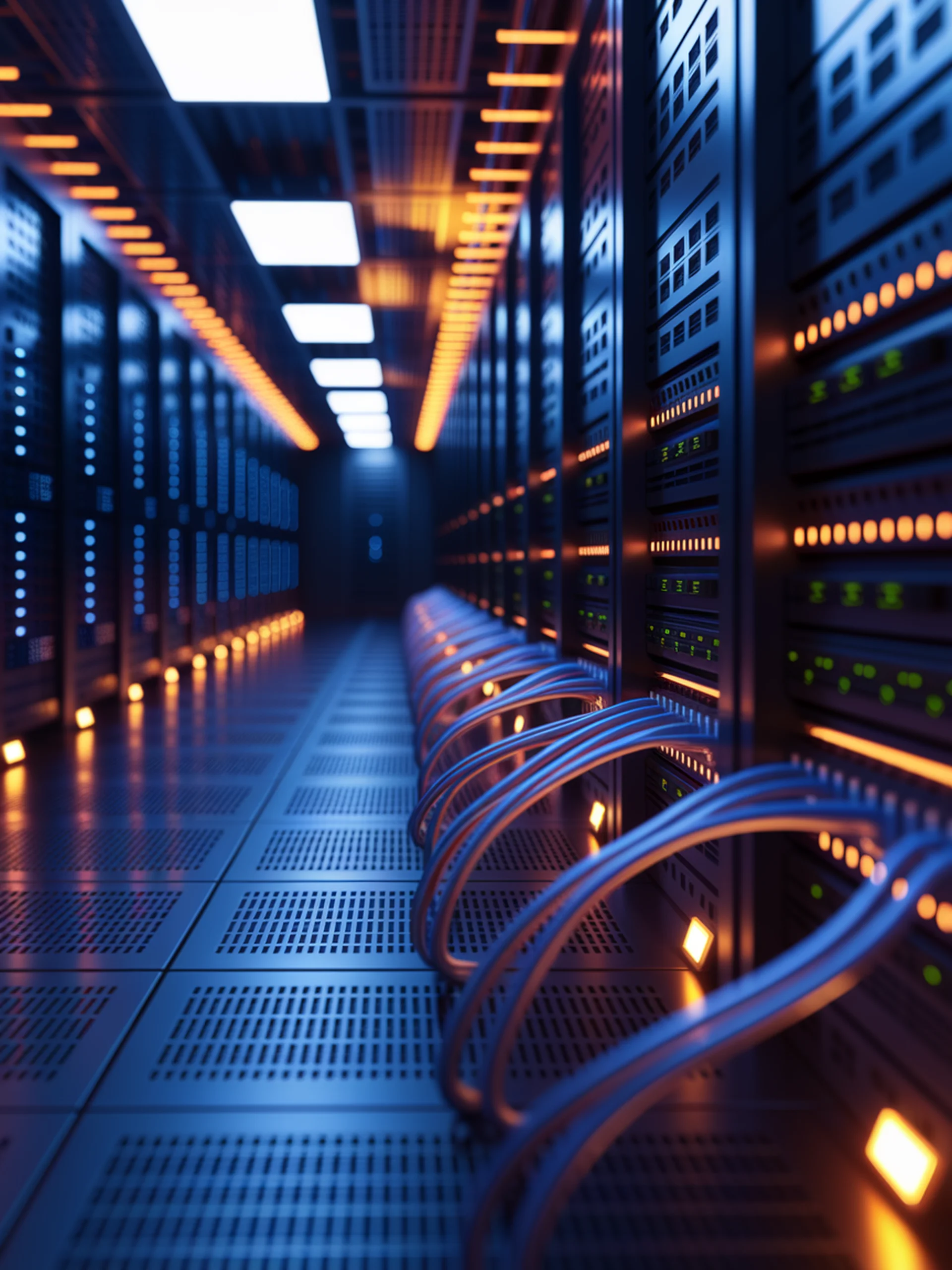
The Scaling Plateau in LLM Training
Diminishing returns challenge hardware efficiency in distributed AI systems
This research reveals critical efficiency challenges in scaling distributed training systems for large language models as hardware deployments grow.
- Training efficiency decreases significantly beyond 400-1000 GPUs due to communication bottlenecks
- Diminishing returns appear regardless of model size or training objective
- Hardware configurations with higher-bandwidth interconnects show better scaling properties
- Future LLM advancement requires rethinking distributed training approaches beyond simple hardware scaling
For engineering teams, this highlights the need to prioritize communication efficiency and alternative scaling strategies rather than just adding more hardware, potentially saving millions in infrastructure costs.
Hardware Scaling Trends and Diminishing Returns in Large-Scale Distributed Training