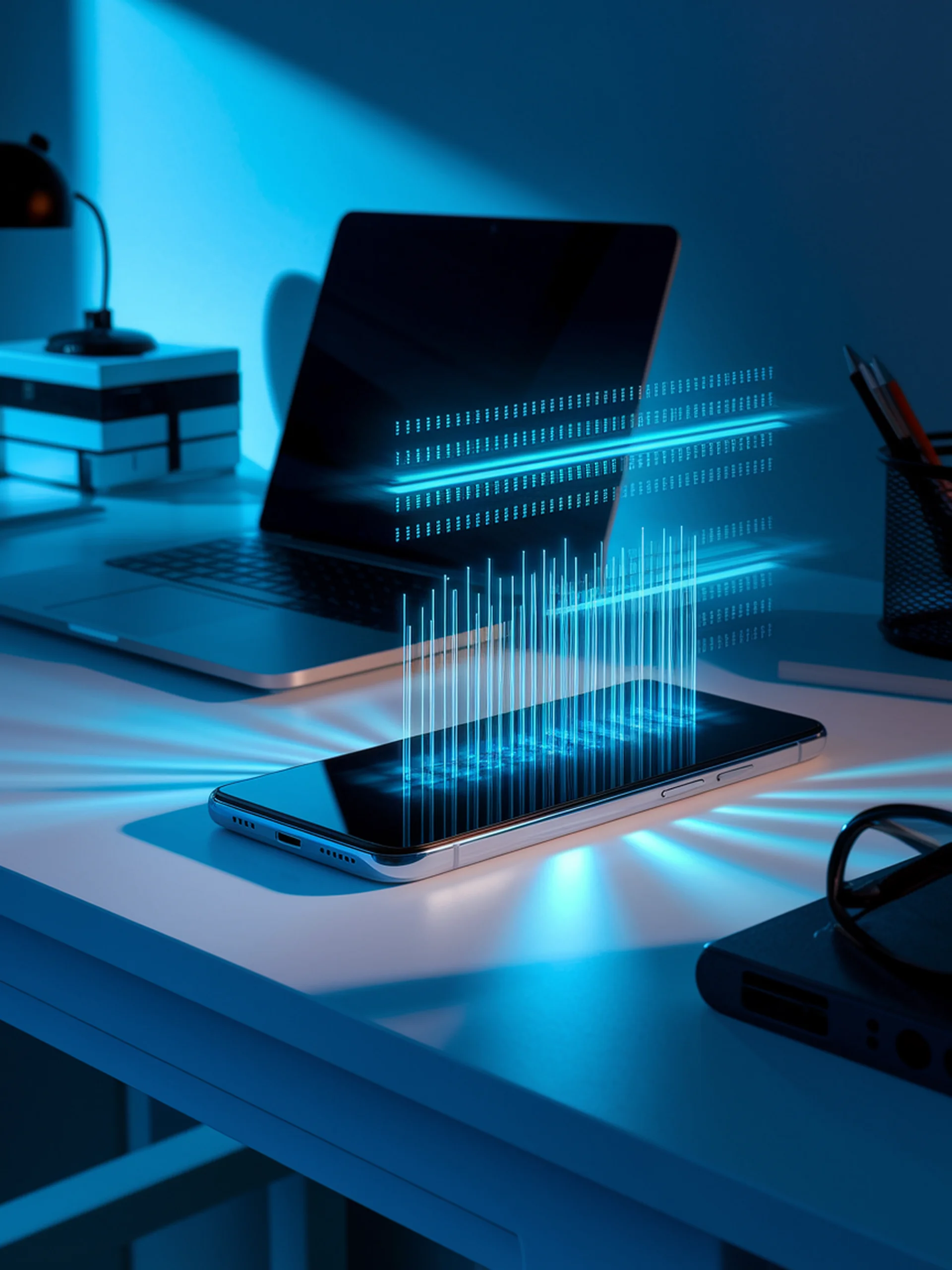
Boosting LLM Speed on Mobile Devices
Overcoming Memory Bottlenecks with Smart Pruning Techniques
This research tackles the critical memory bandwidth limitations that slow down large language models on mobile devices, introducing innovative pruning techniques for modern LLMs.
- Addresses the DRAM bandwidth bottleneck that restricts LLM performance on memory-constrained devices
- Introduces dynamic input pruning optimized for SwiGLU-activated models that lack natural activation sparsity
- Implements cache-aware masking to improve memory efficiency during token generation
- Demonstrates significant performance gains while maintaining model accuracy
These engineering advancements enable faster, more efficient LLM deployment on mobile platforms without hardware upgrades, potentially democratizing access to powerful AI capabilities on everyday devices.
Efficient LLM Inference using Dynamic Input Pruning and Cache-Aware Masking