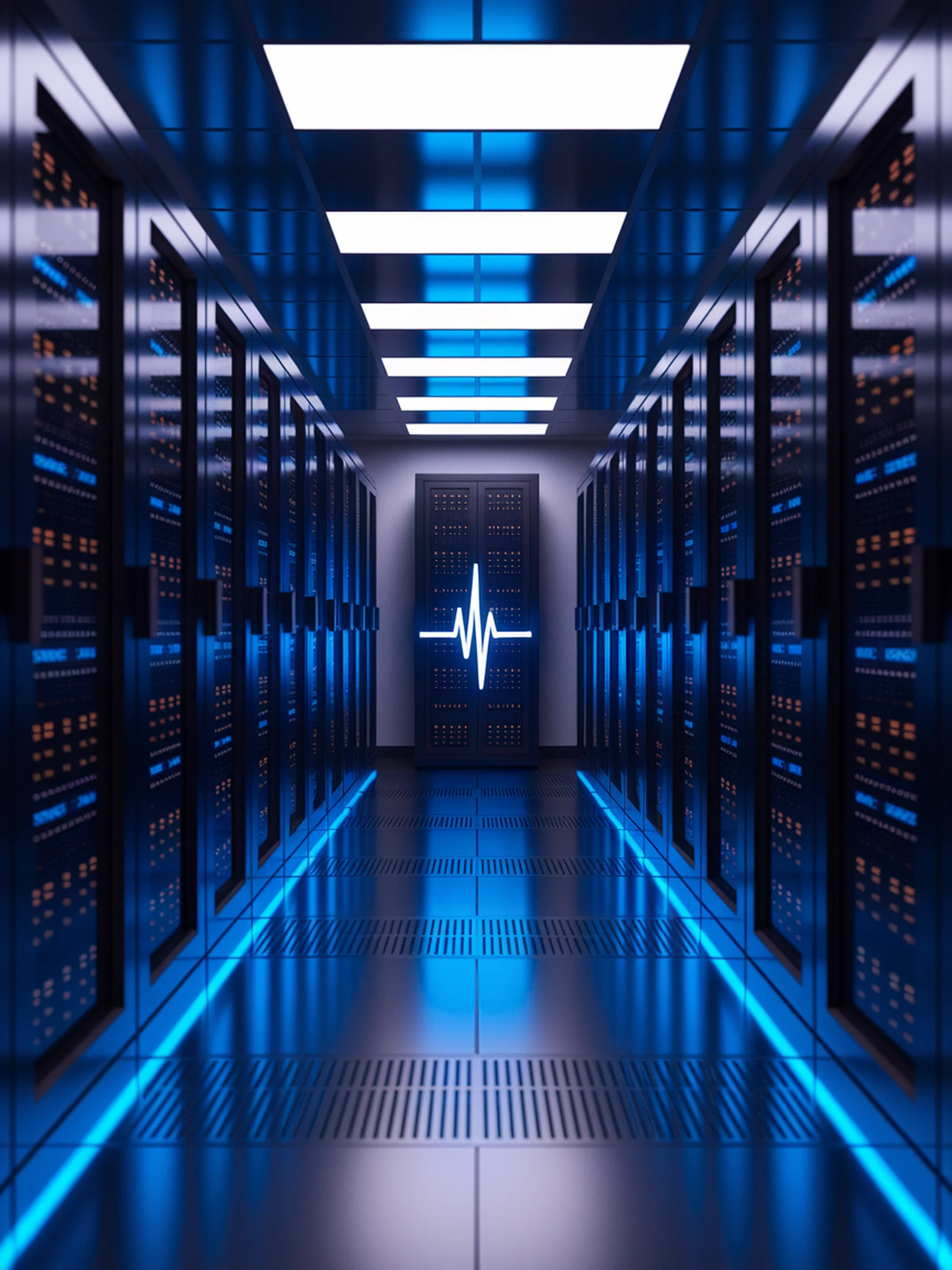
Taming the Spikes in LLM Training
A novel Adam optimizer that dramatically improves training stability
SPAM (Spike-Aware Adam with Momentum) introduces a groundbreaking approach to improve stability and efficiency in large language model training by intelligently handling gradient spikes.
Key innovations:
- Automatic spike detection and momentum resetting mechanism that prevents training instability
- Reduced need for manual interventions like checkpoint recoveries and experiment restarts
- Demonstrated 15-40% improvement in training throughput across various model sizes
- Compatible with existing optimization frameworks with minimal computational overhead
This research addresses critical engineering challenges in LLM development, making training more robust and cost-effective while maintaining or improving model quality.
SPAM: Spike-Aware Adam with Momentum Reset for Stable LLM Training