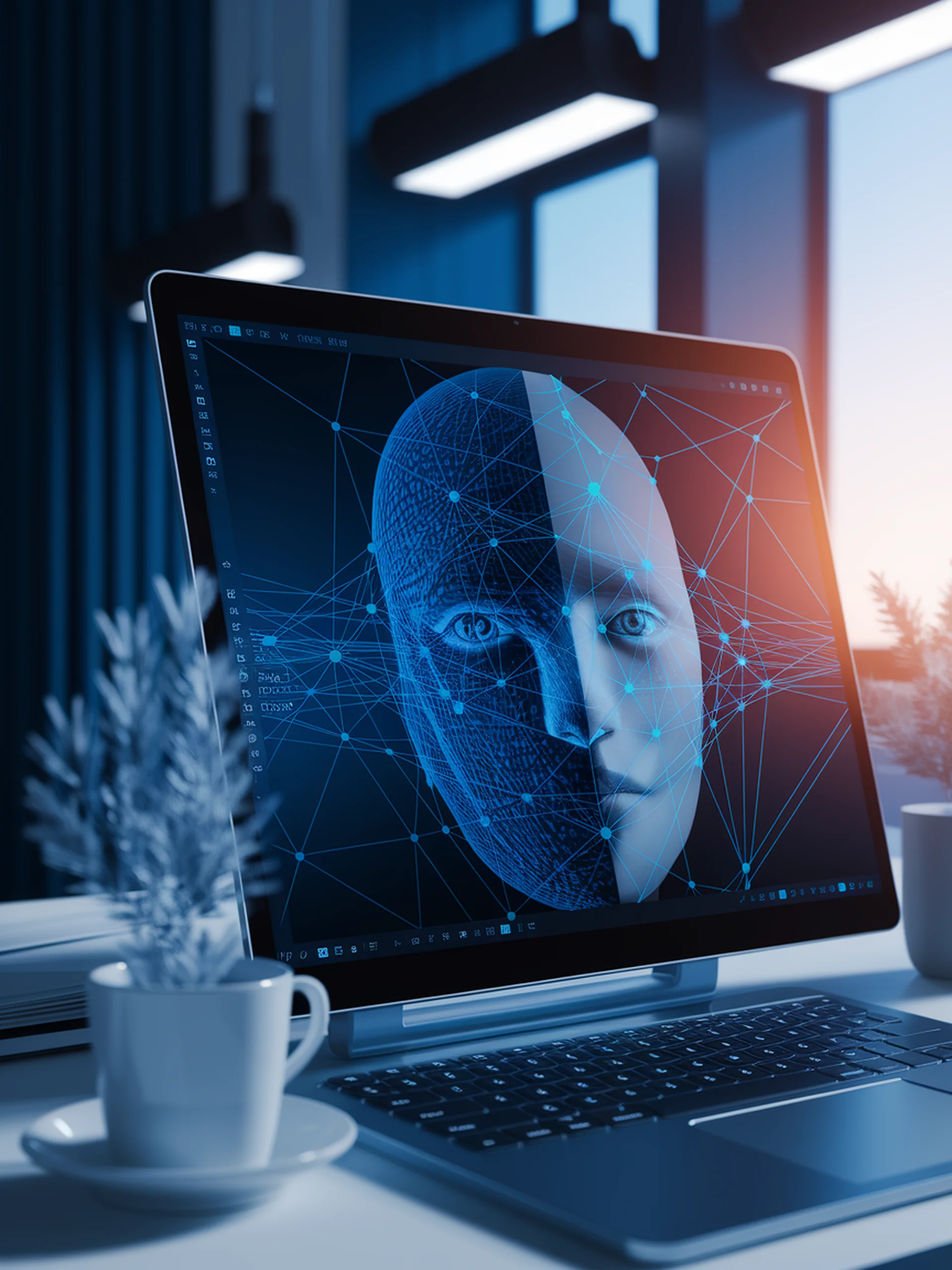
Smart Vision Pruning for Efficient MLLMs
Boosting performance while reducing computational costs
LVPruning introduces a language-guided approach to intelligently reduce vision tokens in multi-modal large language models, significantly decreasing computational burden without sacrificing performance.
- Reduces computational overhead by selectively pruning less important vision tokens
- Achieves up to 50% reduction in computational costs while maintaining model capabilities
- Implements an elegant, lightweight solution that requires minimal changes to existing MLLM architectures
- Enables more efficient deployment of MLLMs in resource-constrained environments
This engineering innovation addresses a critical challenge for multi-modal AI deployment, making sophisticated vision-language models more accessible for real-world applications with limited computational resources.