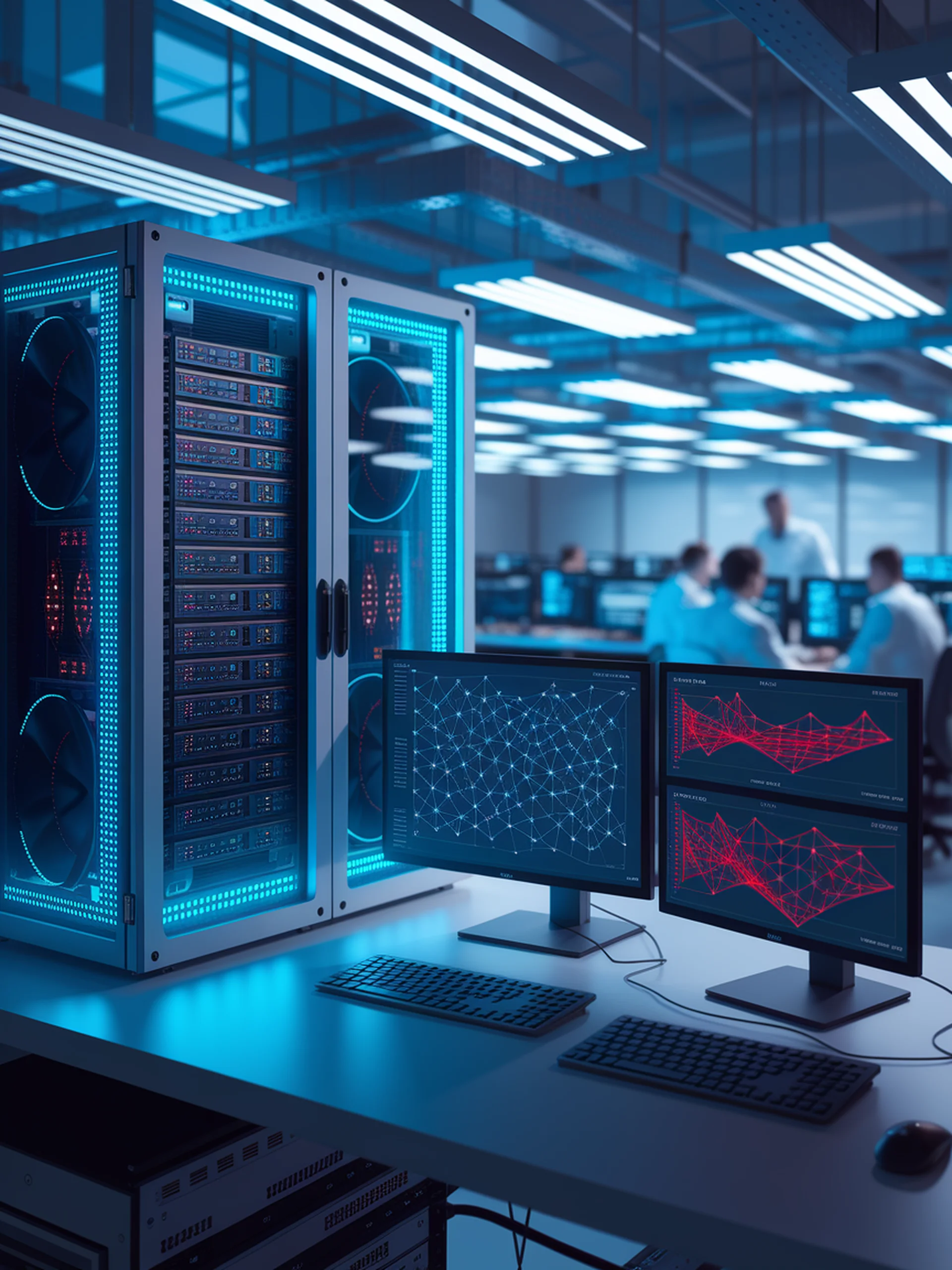
PIFA: Revolutionizing LLM Compression
A Novel Low-Rank Pruning Approach for Efficient AI Deployment
Pivoting Factorization (PIFA) introduces a breakthrough in LLM compression that maintains performance while significantly reducing computational demands.
- Addresses the limitations of traditional low-rank pruning techniques
- Matches or exceeds semi-structured pruning performance at similar densities
- Provides better tensor coherence and GPU compatibility across all density levels
- Enables more efficient deployment of large language models in resource-constrained environments
This engineering innovation is critical for organizations seeking to deploy advanced AI capabilities with lower infrastructure costs and energy consumption, making sophisticated LLMs accessible for wider commercial applications.