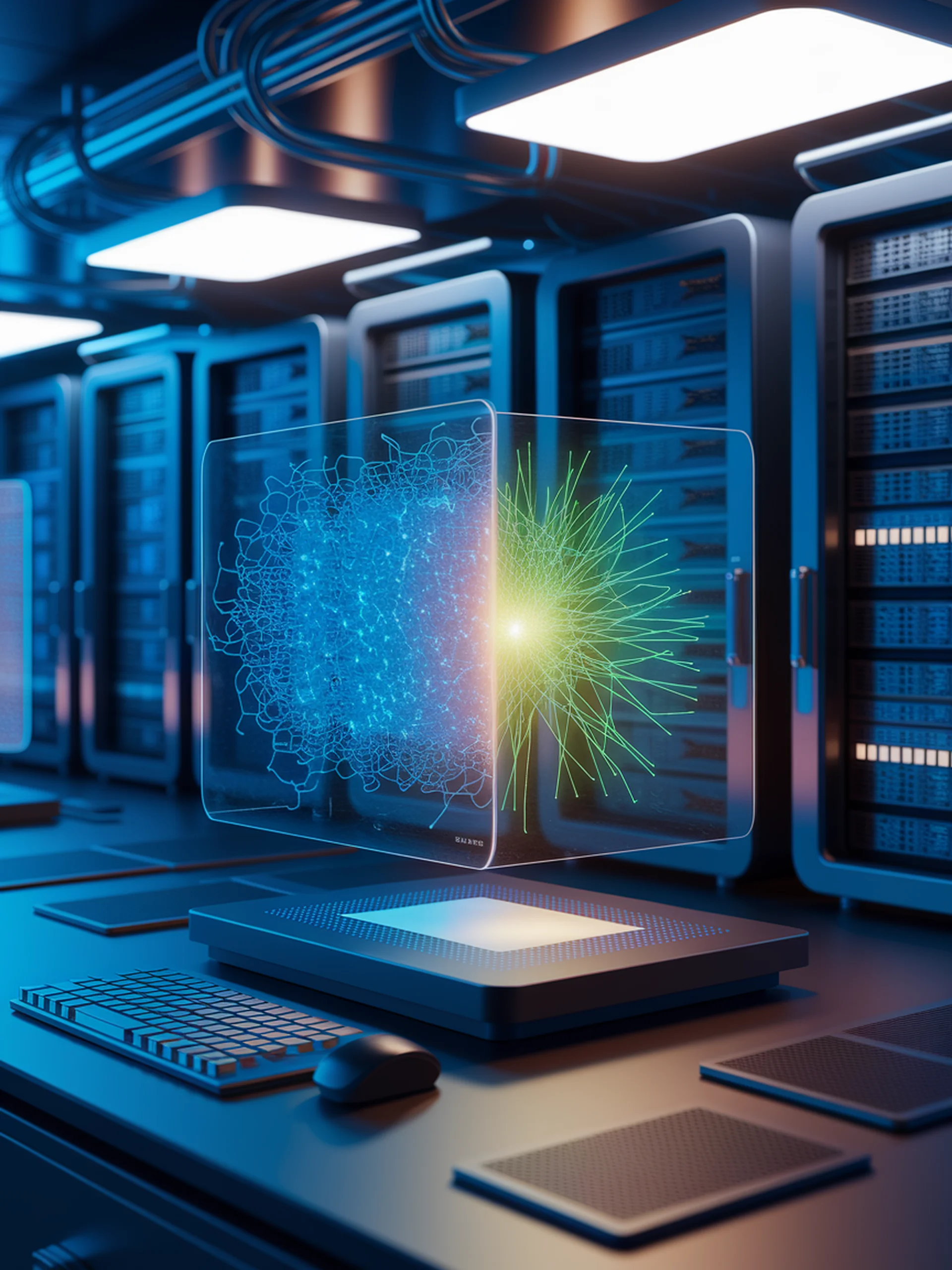
Accelerating Spiking Neural Networks for LLMs
A two-stage conversion approach that maintains performance and reduces computational costs
This research introduces Fast ANN-SNN Conversion (FAS), a novel strategy that efficiently transforms traditional Large Language Models into energy-efficient Spiking Neural Networks while preserving performance.
- Uses a two-stage conversion process with full-parameter fine-tuning of pre-trained models
- Applies specialized calibration methods to minimize conversion errors
- Achieves better performance than existing conversion approaches with lower computational overhead
- Demonstrates practical viability for energy-efficient AI deployment
This engineering advancement matters because it makes neuromorphic computing more practical for large-scale language tasks, potentially enabling more sustainable AI deployments in resource-constrained environments.
FAS: Fast ANN-SNN Conversion for Spiking Large Language Models