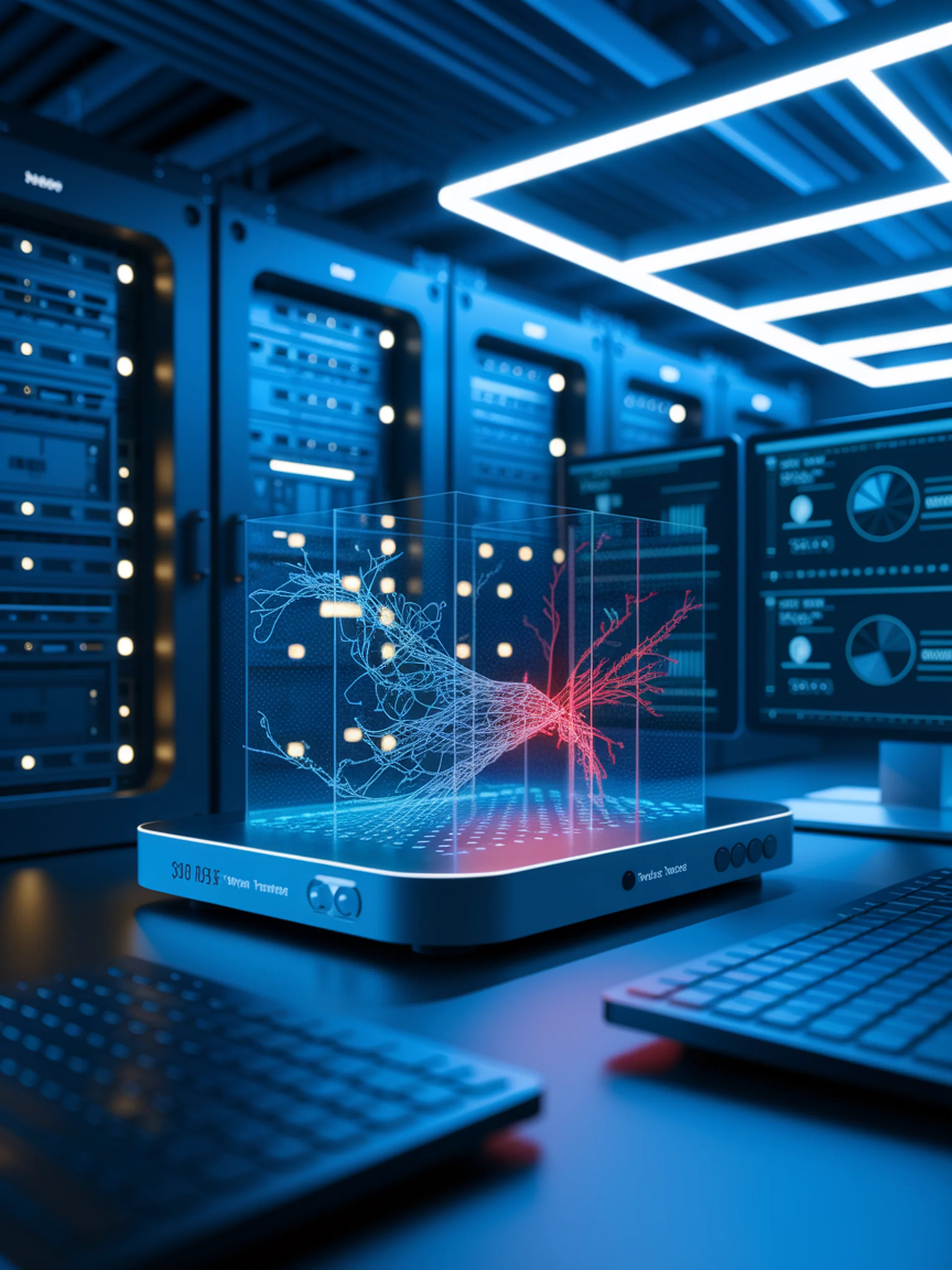
Evolutionary Pruning for Efficient LLMs
A novel approach to optimize LLMs for resource-constrained environments
EvoP introduces an evolutionary pruning framework that dynamically optimizes large language models by removing redundant structures while preserving performance.
- Addresses the challenge of deploying massive LLMs in resource-limited settings
- Improves upon traditional heuristic pruning methods with an evolutionary approach
- Considers data characteristics during optimization to maintain model functionality
- Enables more robust LLM inference with lower computational requirements
This research matters for engineering because it offers a practical pathway to deploy powerful language models on devices with limited computational capacity, potentially expanding LLM applications across more platforms and use cases.