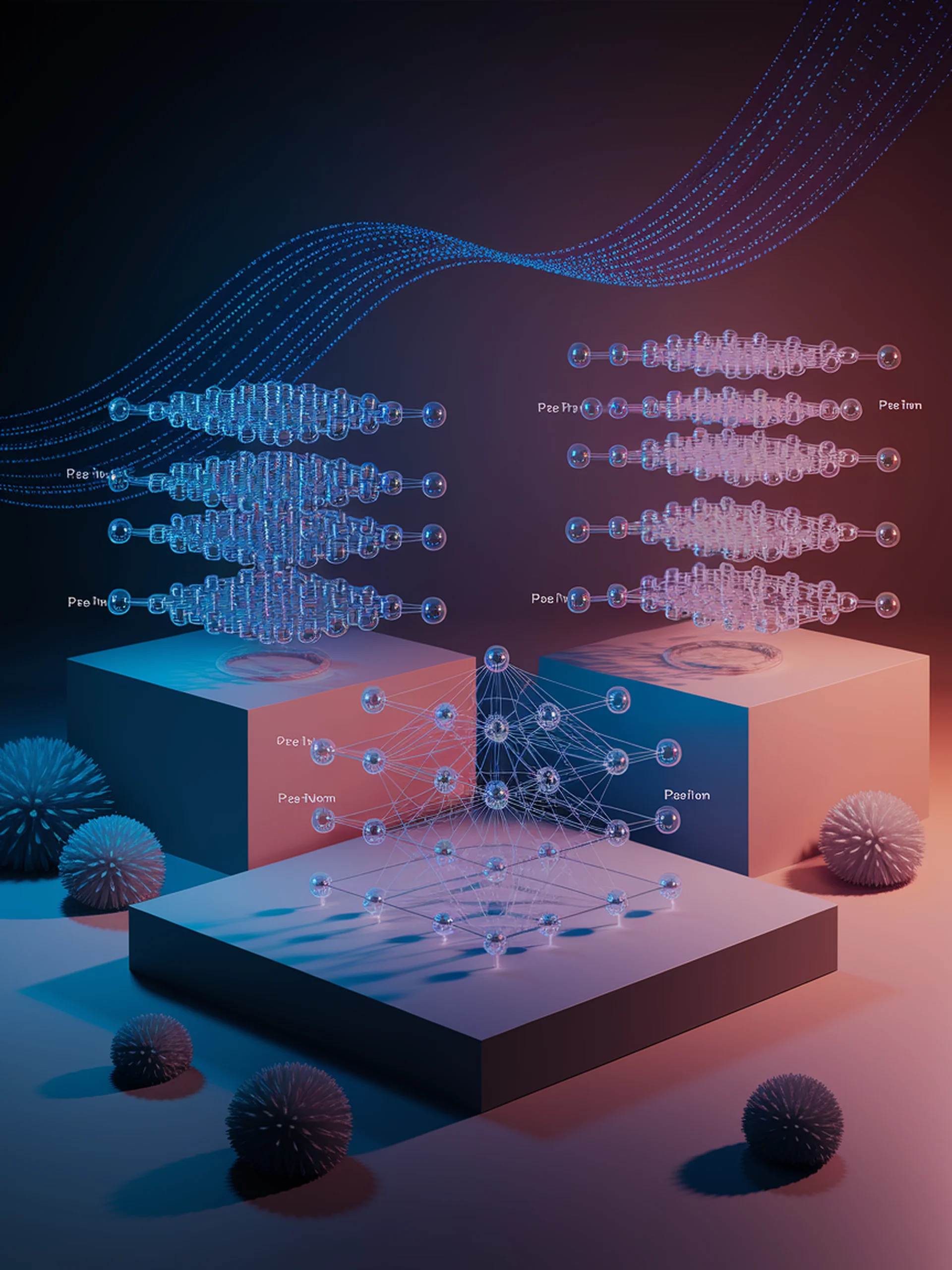
HybridNorm: Stabilizing Transformer Training
A novel normalization approach that combines the best of Pre-Norm and Post-Norm architectures
HybridNorm introduces a new approach to layer normalization in transformer architectures, addressing the stability-performance tradeoff in large language models.
- Combines advantages of Pre-Norm (training stability) and Post-Norm (performance) approaches
- Enables more stable training for deep transformer networks
- Improves efficiency of training resource utilization
- Particularly beneficial for engineering more robust large language models
This innovation matters for engineering because it offers a practical solution to a fundamental architectural challenge in transformer models, potentially enabling more efficient development of high-performing language models.
HybridNorm: Towards Stable and Efficient Transformer Training via Hybrid Normalization