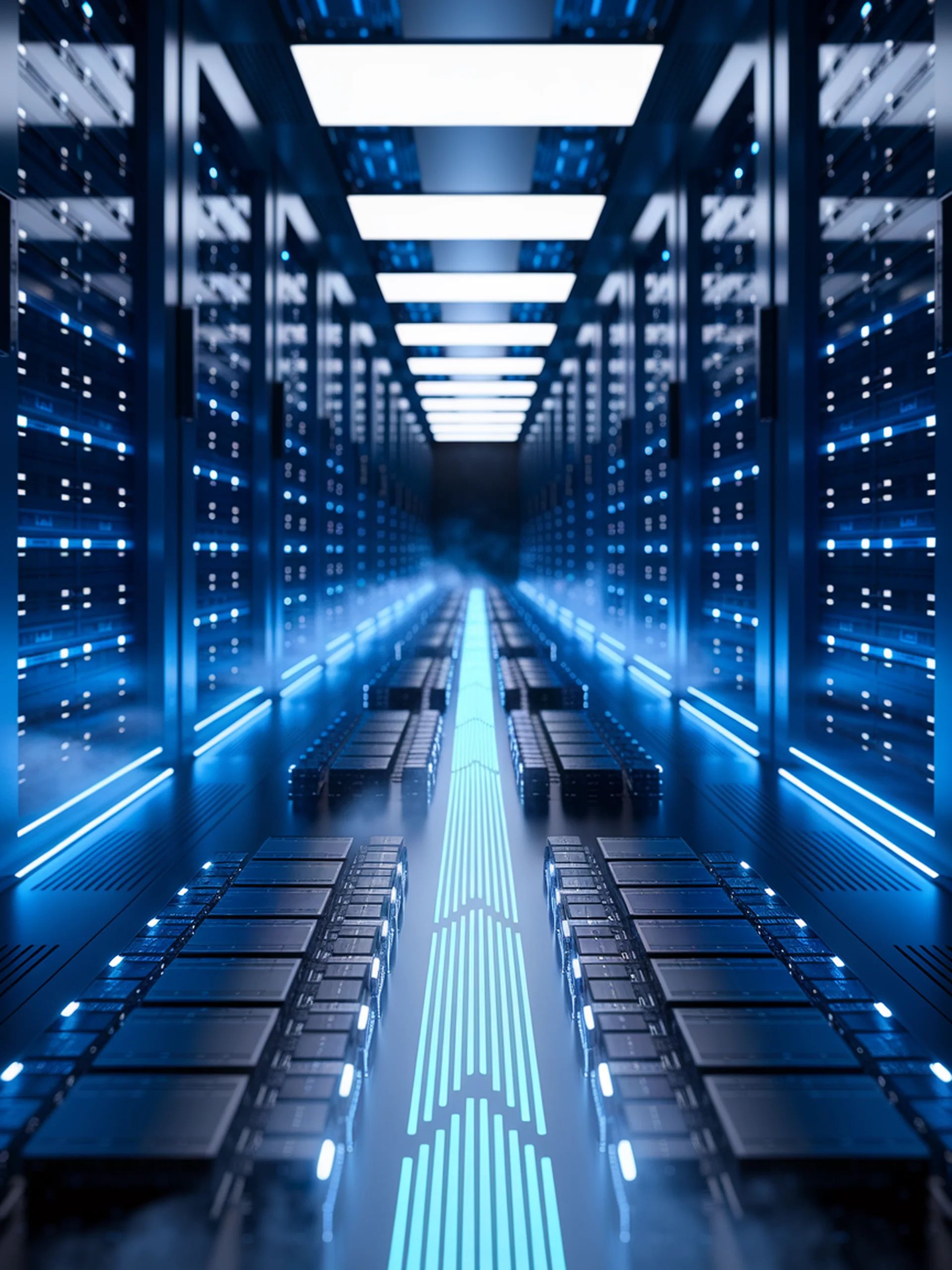
LogQuant: Transforming LLM Memory Efficiency
2-Bit Quantization for KV Cache with Minimal Performance Loss
LogQuant introduces an innovative approach to memory optimization in LLM inference by using log-distributed 2-bit quantization for KV Cache, significantly reducing memory requirements while maintaining model performance.
- Achieves superior accuracy preservation compared to existing quantization methods
- Uses a log-based distribution that more effectively captures value ranges in attention mechanisms
- Enables efficient LLM deployment on memory-constrained devices without sacrificing quality
- Addresses performance bottlenecks present in previous token-importance prediction approaches
This engineering advancement has significant implications for Enterprise AI deployment, making high-quality LLM inference more accessible and cost-effective at scale.
LogQuant: Log-Distributed 2-Bit Quantization of KV Cache with Superior Accuracy Preservation