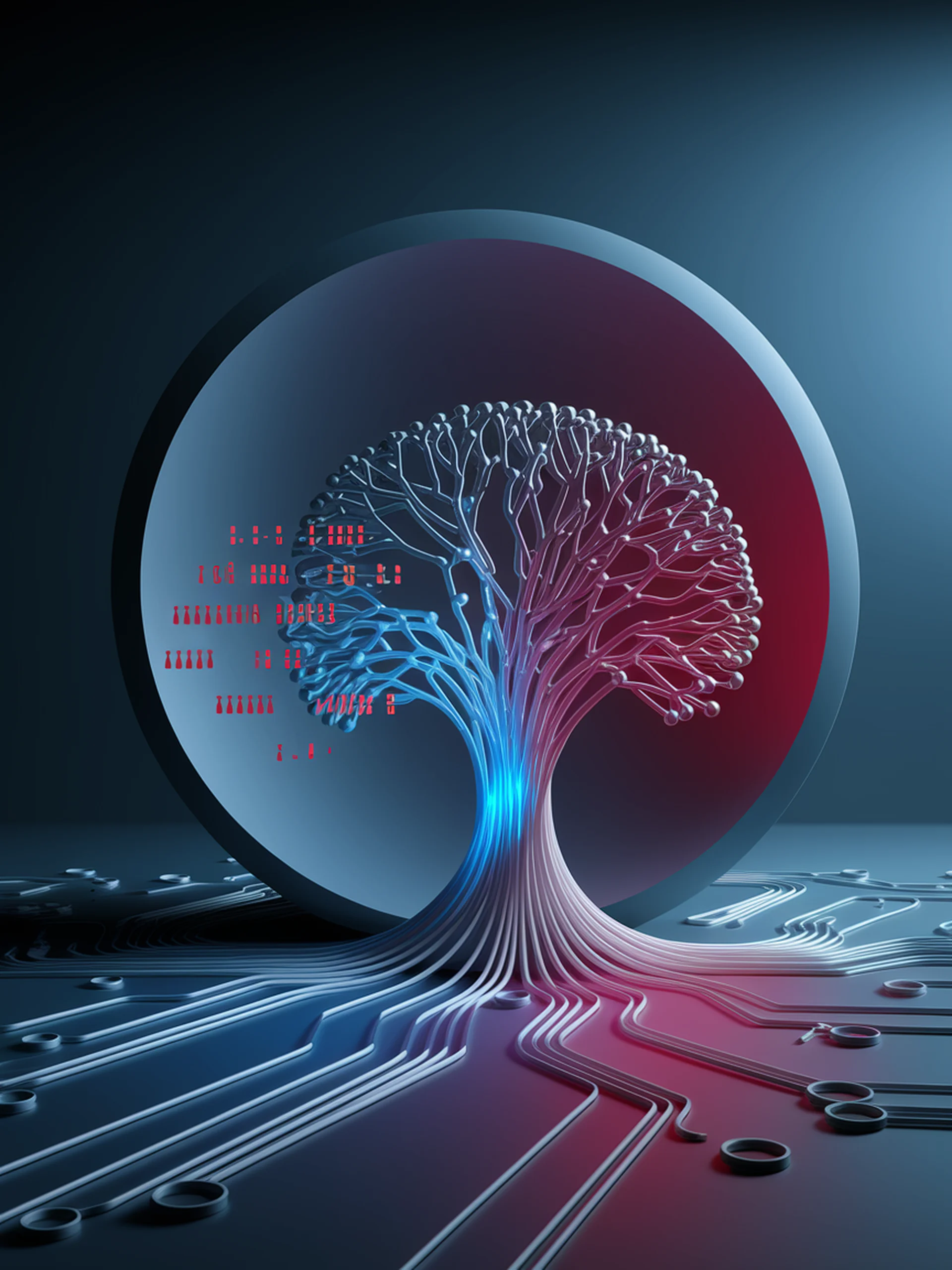
Smarter LLM Pruning with Entropy
Reducing model size while preserving performance
This research introduces a novel entropy-based pruning strategy that significantly reduces computational and storage demands of large language models.
- Identifies redundancy patterns in Transformer blocks by tracking entropy changes
- Strategically removes less important blocks based on entropy measurements
- Maintains model performance while improving efficiency and deployment feasibility
- Provides a systematic approach for LLM optimization applicable across model architectures
For engineering teams, this approach offers practical solutions to the resource constraints that currently limit LLM deployment in production environments.
Entropy-Based Block Pruning for Efficient Large Language Models