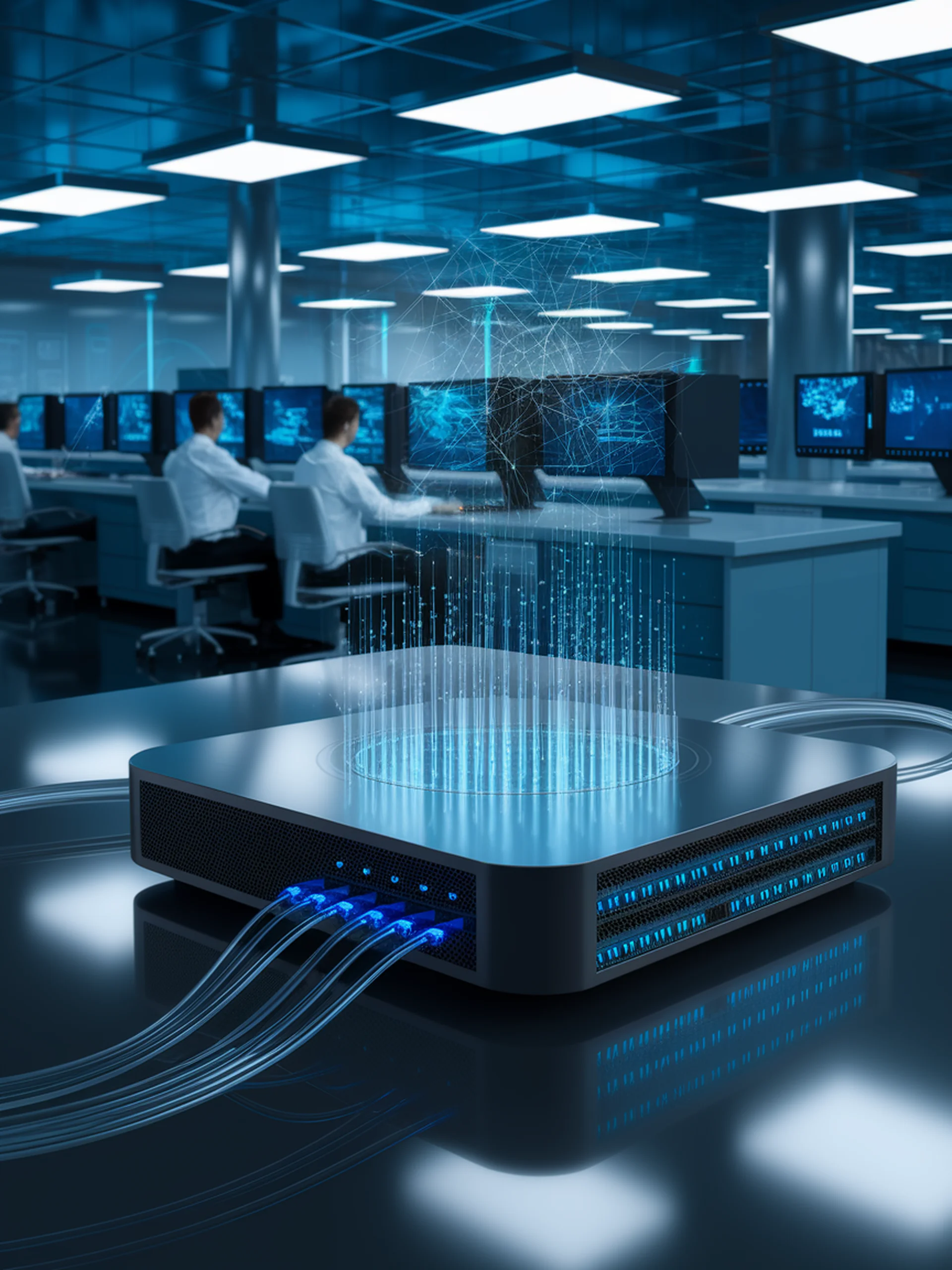
Quantum-Enhanced Attention for LLMs
Reducing computational costs with quantum annealing
QAMA introduces the first quantum annealing-based multi-head attention mechanism that seamlessly integrates with classical deep learning frameworks, addressing the exponential growth in memory and energy costs of traditional attention mechanisms.
- Combines quantum annealing computing with classical deep learning architectures
- Achieves computational efficiency and reduced energy consumption
- Provides a practical pathway for quantum-classical hybrid AI systems
- Demonstrates how quantum computing can address scaling limitations in large language models
This engineering breakthrough opens new opportunities for sustainable AI scaling, potentially enabling more efficient large language models without the prohibitive computational costs of conventional approaches.
QAMA: Quantum annealing multi-head attention operator with classical deep learning framework