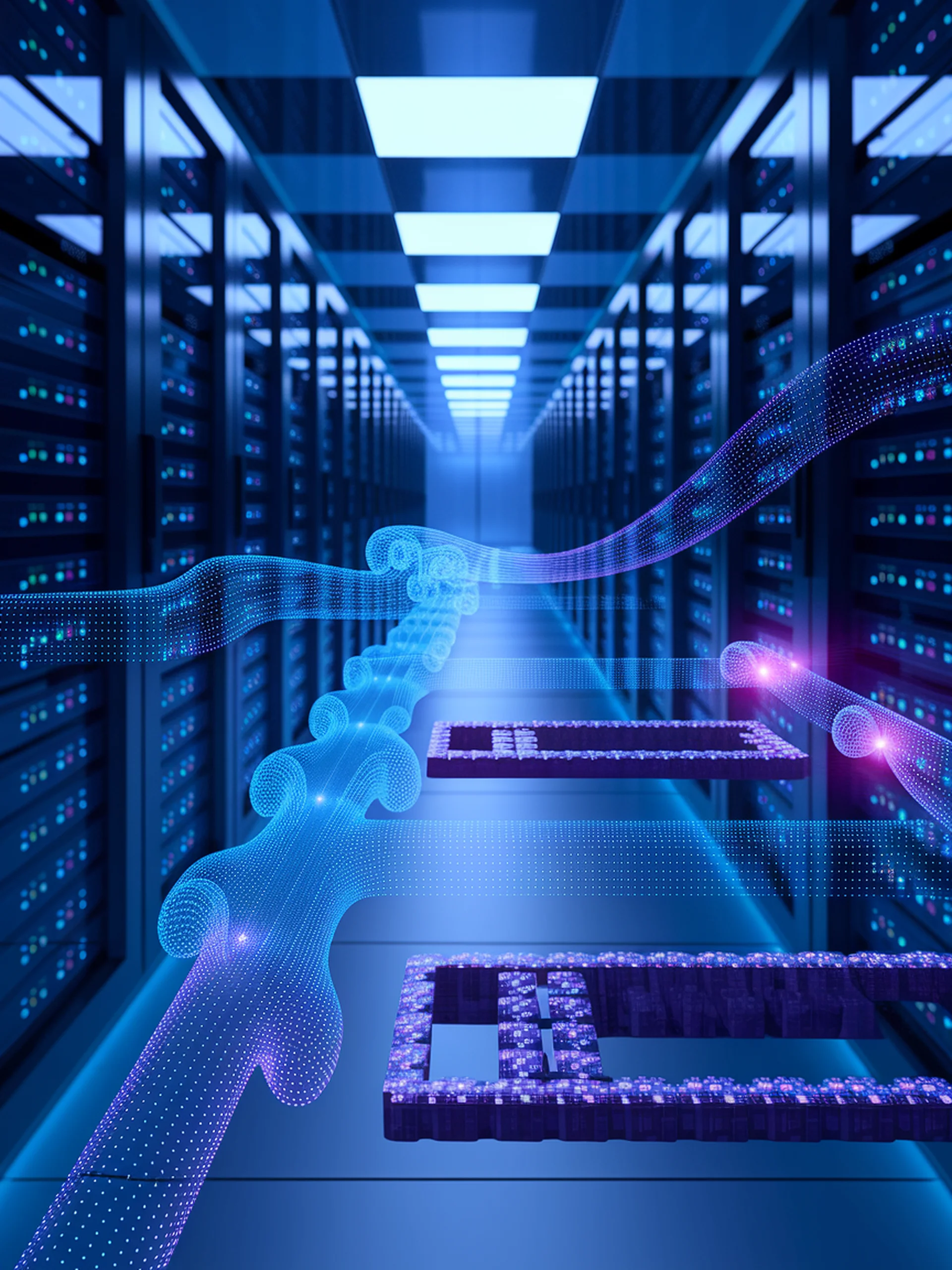
Boosting LLM Performance Under Constraints
A fluid-dynamic approach to optimizing LLM inference with limited memory
This research introduces a novel online scheduling framework for LLM inference that maximizes throughput while operating under tight memory constraints.
- Formulates LLM inference as a multi-stage online scheduling problem that handles dynamic KV cache growth
- Develops a fluid-guided algorithm that outperforms conventional scheduling approaches
- Demonstrates significant efficiency improvements for real-world LLM applications
- Addresses critical bottlenecks in computational resource management for language model deployment
This engineering breakthrough offers practical solutions for organizations deploying LLMs at scale, potentially reducing infrastructure costs while maintaining performance quality.
Optimizing LLM Inference: Fluid-Guided Online Scheduling with Memory Constraints