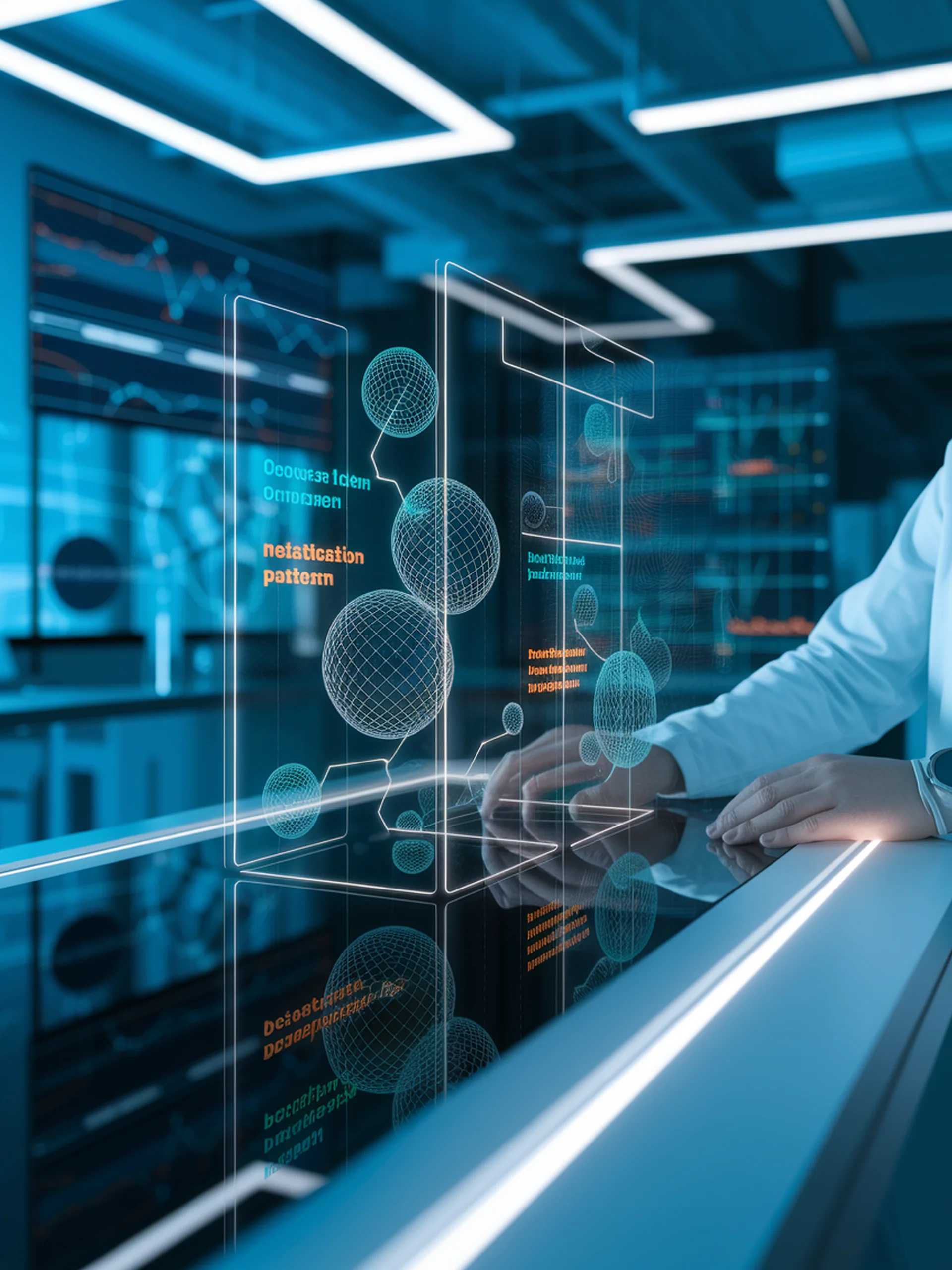
Enhancing Causal Discovery with AI
Leveraging LLMs to integrate expert knowledge in causal inference
This research introduces Statistical Causal Prompting (SCP), a novel method that combines statistical causal discovery with LLM-powered knowledge inference to create more robust causal models.
- Addresses a critical challenge in causal discovery: systematically incorporating domain expertise
- Synthesizes statistical approaches with LLM capabilities to generate consistent, meaningful causal relationships
- Enables practical application of causal discovery in complex domains without requiring extensive manual expert input
- Creates a feedback loop between statistical evidence and knowledge-based inference
Medical Impact: In healthcare settings, this approach could significantly improve the identification of causal relationships in patient data, leading to better treatment protocols and more accurate diagnostic models while reducing the burden on medical experts to manually encode domain knowledge.
Integrating Large Language Models in Causal Discovery: A Statistical Causal Approach