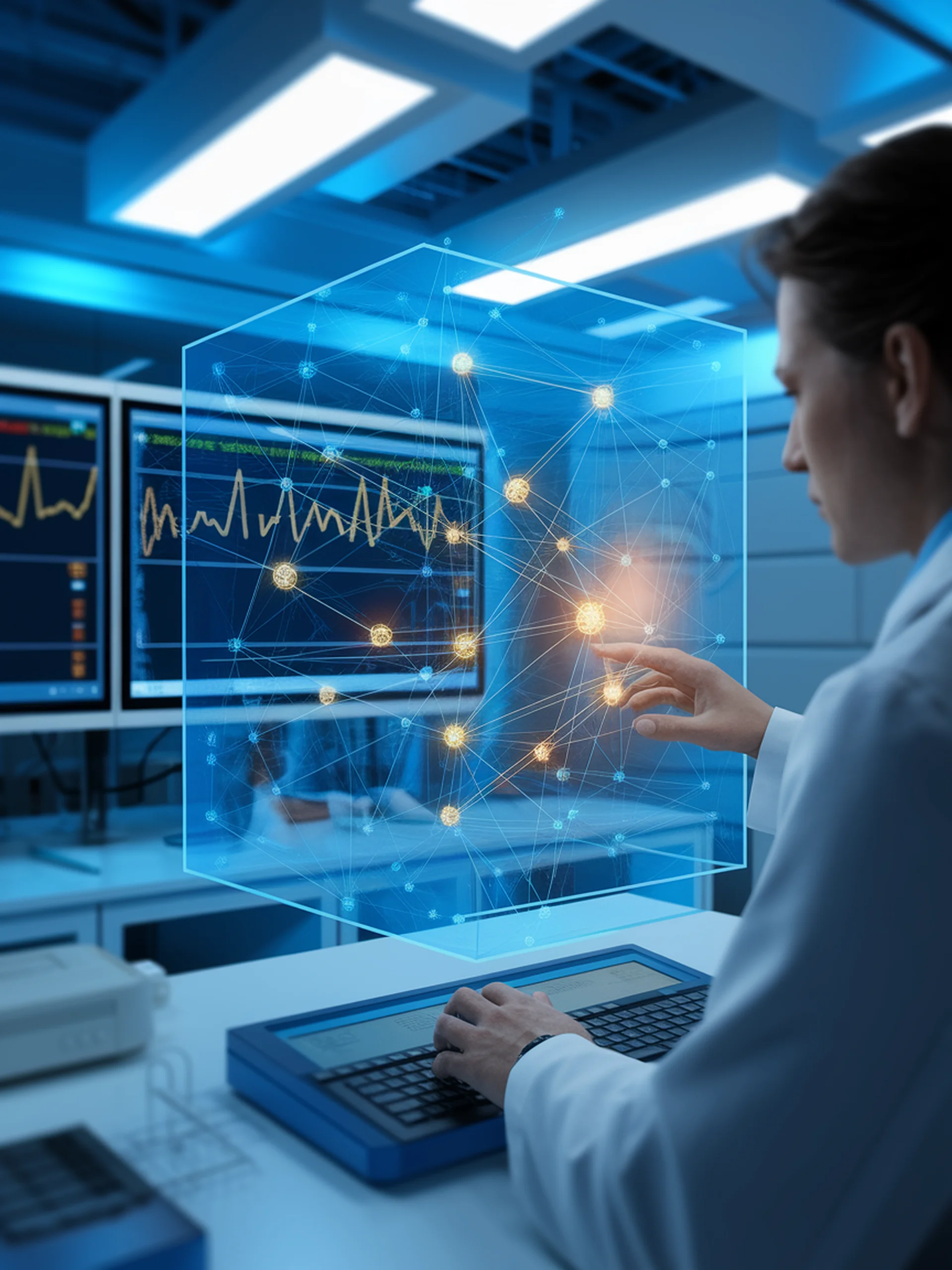
Enhancing LLMs with Causal Reasoning
Graph-Augmented Models for Better Medical Decision Making
This research introduces a novel approach combining causal graphs with large language models to enhance complex reasoning capabilities in knowledge-intensive domains.
- Improves medical question-answering accuracy by up to 10%
- Enhances reasoning transparency through explicit causal relationships
- Reduces hallucinations by grounding LLM outputs in structured knowledge
- Enables better incorporation of domain-specific knowledge
For healthcare applications, this advancement means more reliable clinical decision support, improved diagnostic reasoning, and better explainability of AI-generated medical insights—critical for high-stakes medical scenarios where understanding causal relationships is essential.
Causal Graphs Meet Thoughts: Enhancing Complex Reasoning in Graph-Augmented LLMs