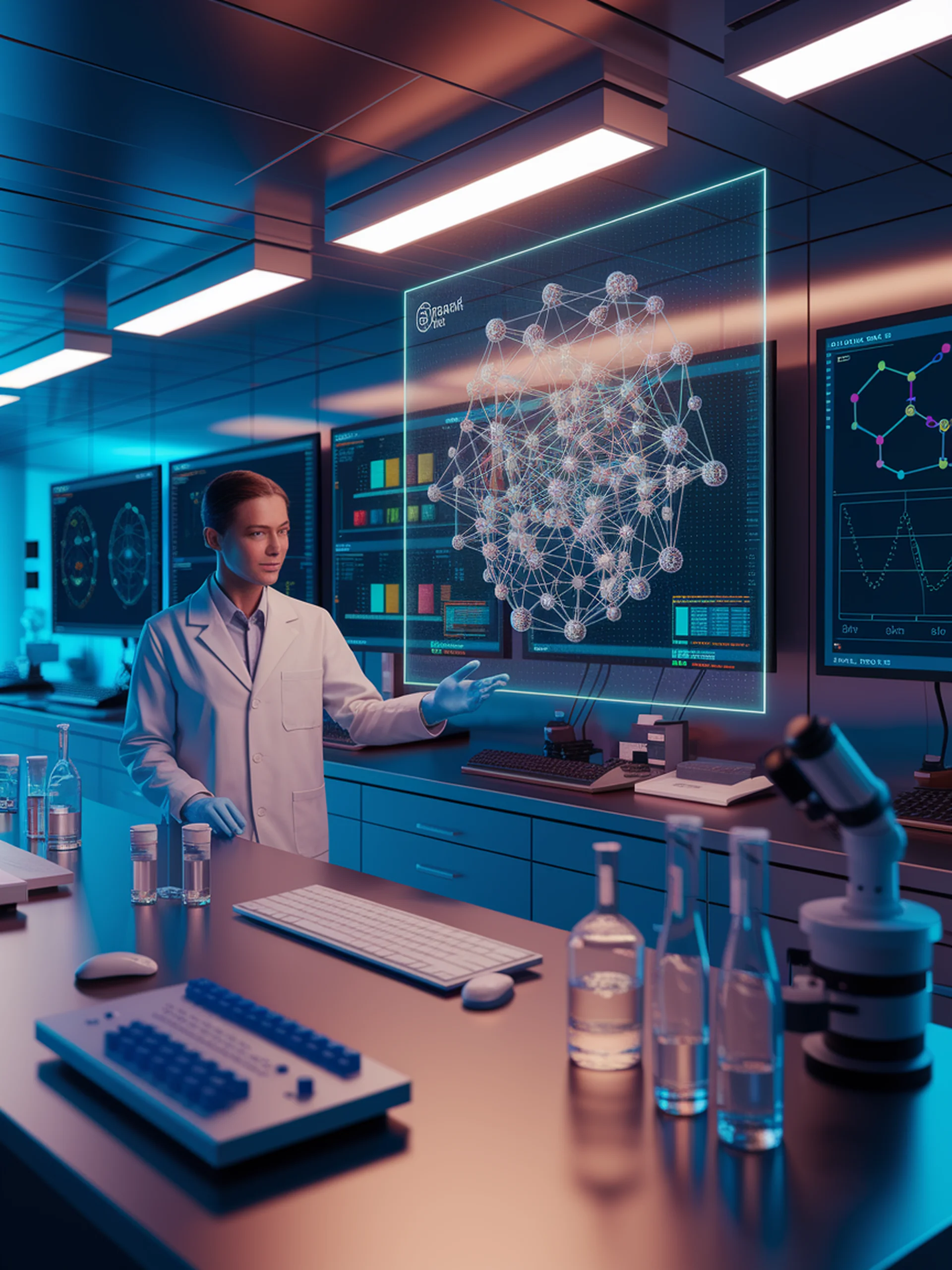
Scaling GNNs for Materials Science Breakthroughs
Optimizing Graph Neural Networks for Atomistic Modeling
Research revealing how to scale Graph Neural Networks (GNNs) for superior performance in atomistic materials modeling, with direct applications in engineering and materials science.
- GNNs represent the state-of-the-art approach for modeling complex atomic structures
- The research establishes scaling laws showing how model size, data volume, and computational resources relate to prediction accuracy
- Findings enable more efficient model design for materials discovery applications
- Results can accelerate innovations in structural materials, electronics, and drug discovery
For engineering teams, these insights provide a framework for optimizing computational resources when designing new materials, potentially reducing R&D cycles and costs.
Scaling Laws of Graph Neural Networks for Atomistic Materials Modeling