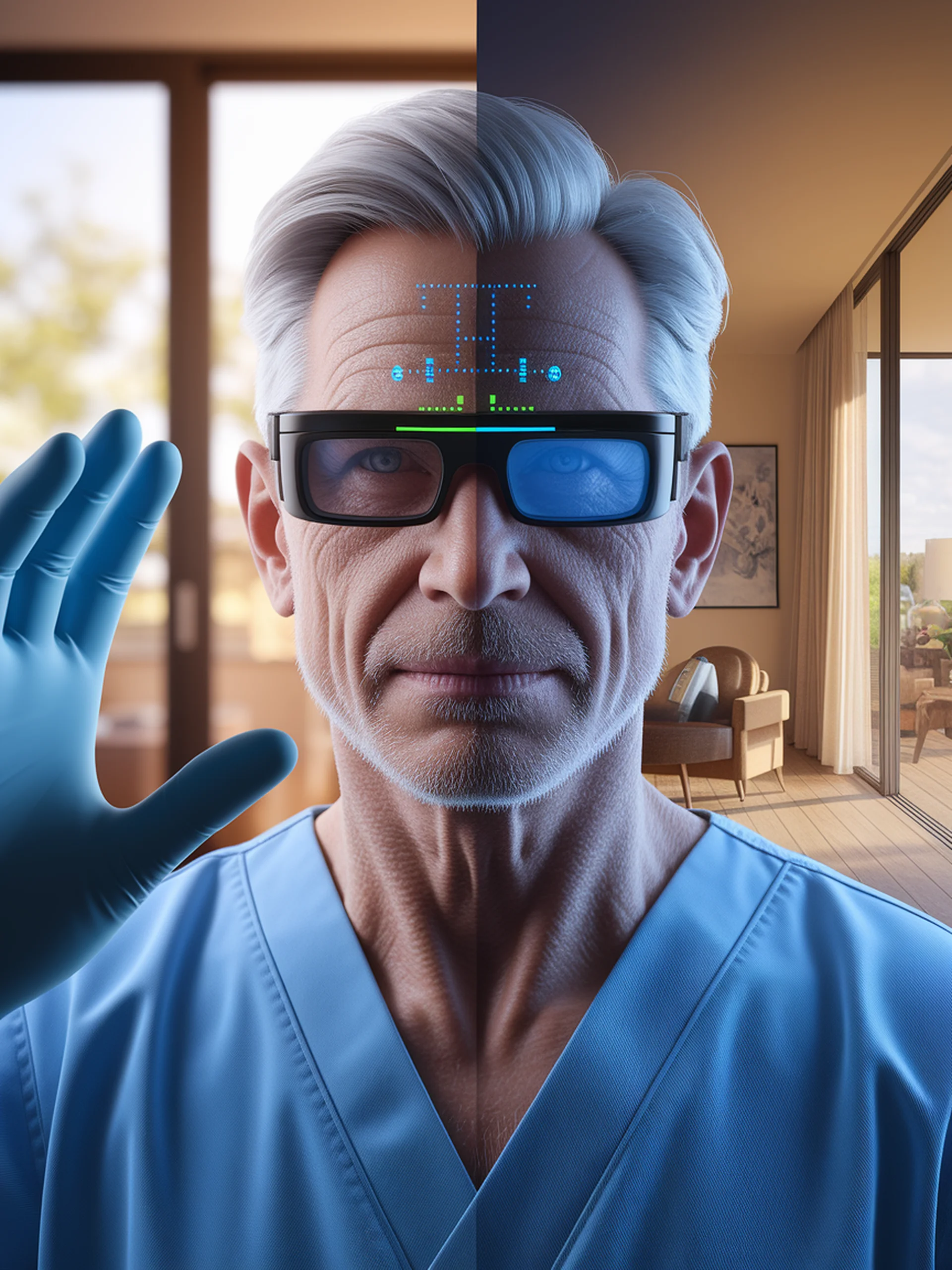
Enhancing AI Vision with Ego-Augmented Learning
Using Egocentric Perspectives to Improve Understanding of Daily Activities
This research introduces a novel approach that enhances Large Vision Language Models' ability to understand human activities by integrating egocentric (first-person) views with exocentric (third-person) perspectives.
Key Innovations:
- Proposes ego2exo knowledge distillation to create more comprehensive representations of daily activities
- Leverages the complementary nature of first-person perspectives to capture fine-grained interactions
- Addresses critical limitations in current LVLMs for understanding Activities of Daily Living (ADL)
- Improves spatial relationship recognition and object interaction detection
Medical Impact: This advancement enables more accurate monitoring and assessment of Activities of Daily Living—critical for healthcare applications in rehabilitation, elder care, and patient independence evaluation. Better ADL understanding can lead to improved remote patient monitoring systems and more effective care protocols.