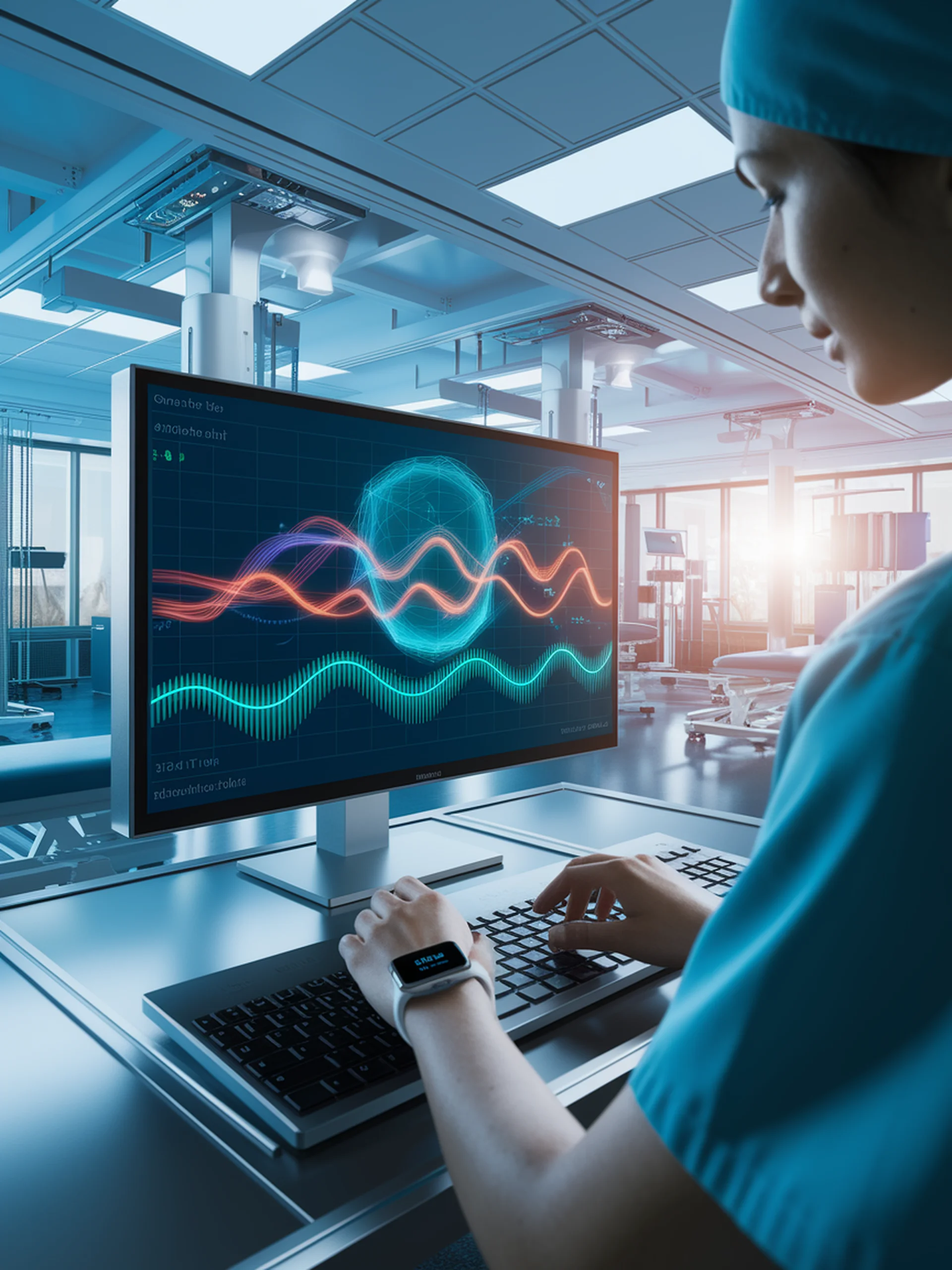
SensorLLM: Bridging Language Models and Activity Recognition
Teaching LLMs to understand human movement through sensor data
SensorLLM is a novel two-stage framework that enables Large Language Models (LLMs) to interpret motion sensor data for human activity recognition, opening new possibilities for sensor-based applications.
- Creates a fusion between powerful language models and numerical sensor data
- Addresses key limitations through specialized sensor-language alignment techniques
- Overcomes computational constraints for practical implementation
- Enables LLMs to process and interpret numerical time-series inputs
In medical applications, this breakthrough allows for enhanced patient monitoring, rehabilitation tracking, and health status assessment through wearable sensors—providing continuous, interpretable activity data that can inform clinical decisions and improve patient care.
SensorLLM: Aligning Large Language Models with Motion Sensors for Human Activity Recognition