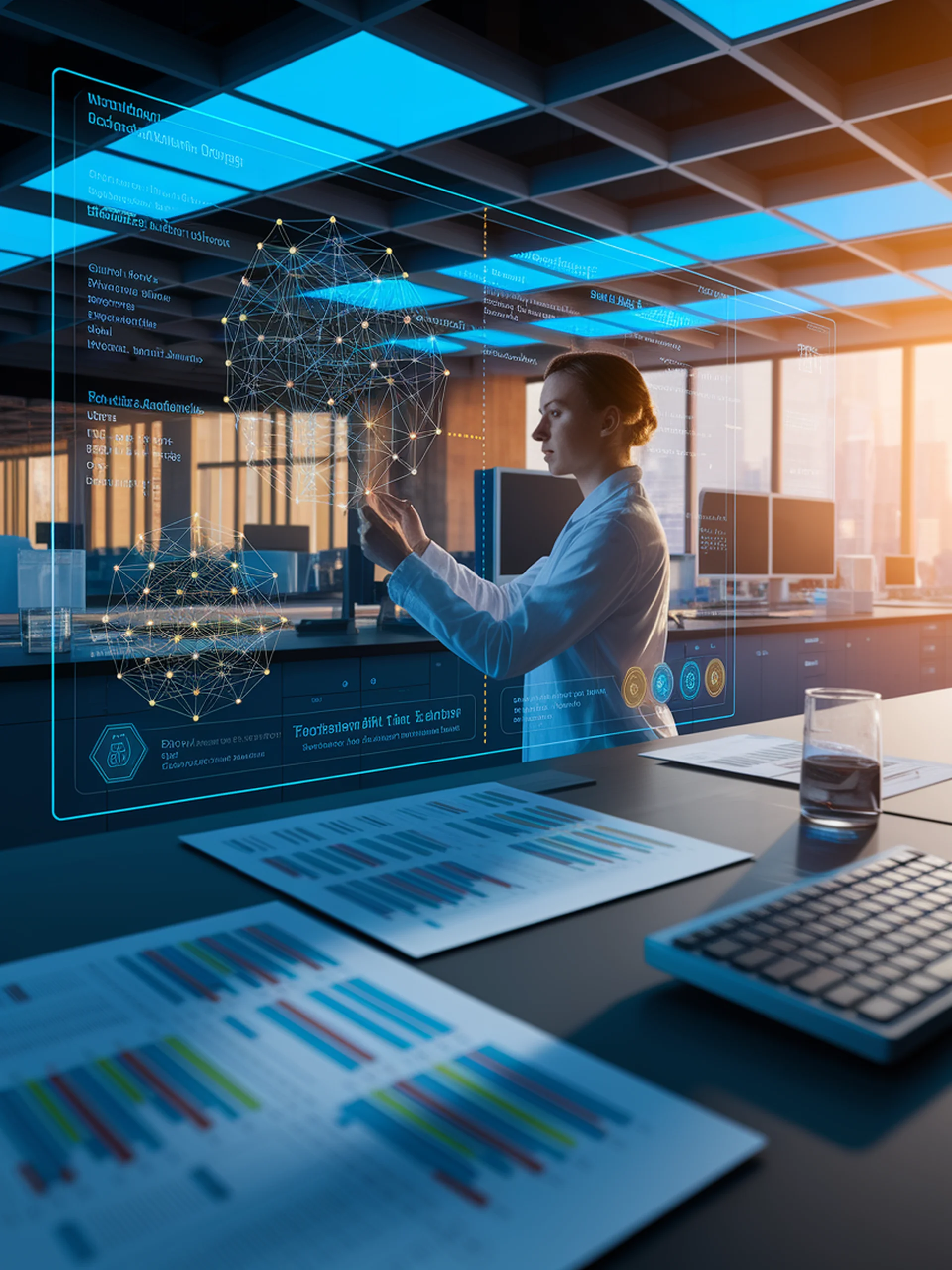
Unlocking LLM Reasoning for Feature Generation
How advanced LLM reasoning techniques enhance machine learning capabilities
This research explores how Large Language Models' reasoning abilities can be leveraged to create more effective feature generation rules for machine learning tasks.
- Examines four key reasoning approaches: Chain of Thought, Tree of Thoughts, Retrieval-Augmented Generation, and Thought Space Exploration
- Demonstrates how these techniques can identify effective feature generation rules without extensive domain knowledge
- Offers practical applications across multiple domains including medical, finance, engineering, and education
In the medical domain, these approaches enable efficient extraction of critical information from clinical notes and radiology reports, potentially transforming healthcare data utilization and analysis.
Applications of Large Language Model Reasoning in Feature Generation