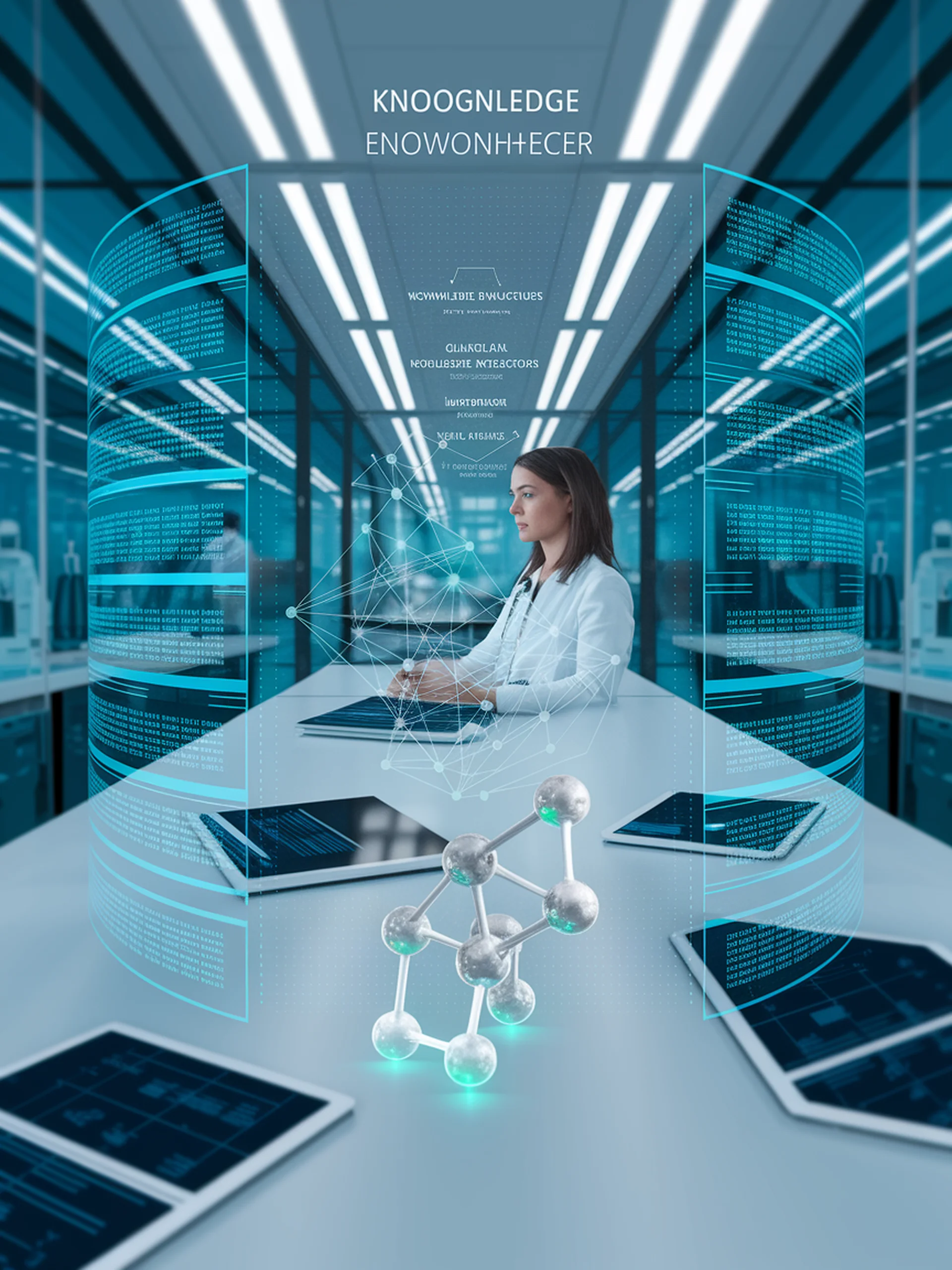
Advancing RAG: Multi-Level Knowledge Integration
Enhancing LLM responses with hierarchical knowledge retrieval
Multiple Abstraction Level RAG (MALRAG) improves on traditional Retrieval-Augmented Generation by retrieving information at different levels of abstraction, providing more comprehensive and contextually appropriate responses.
- Creates a hierarchical knowledge structure from textbooks to enable retrieval at multiple abstraction levels
- Demonstrates superior performance compared to conventional RAG and fine-tuning approaches
- Achieves more nuanced responses by leveraging both high-level concepts and specific details
- Proves particularly effective in specialized domains like Glycoscience
This research has significant implications for medical applications by enabling more precise and medically-accurate responses in specialized healthcare domains, while maintaining the flexibility to adapt to new medical knowledge without expensive retraining.