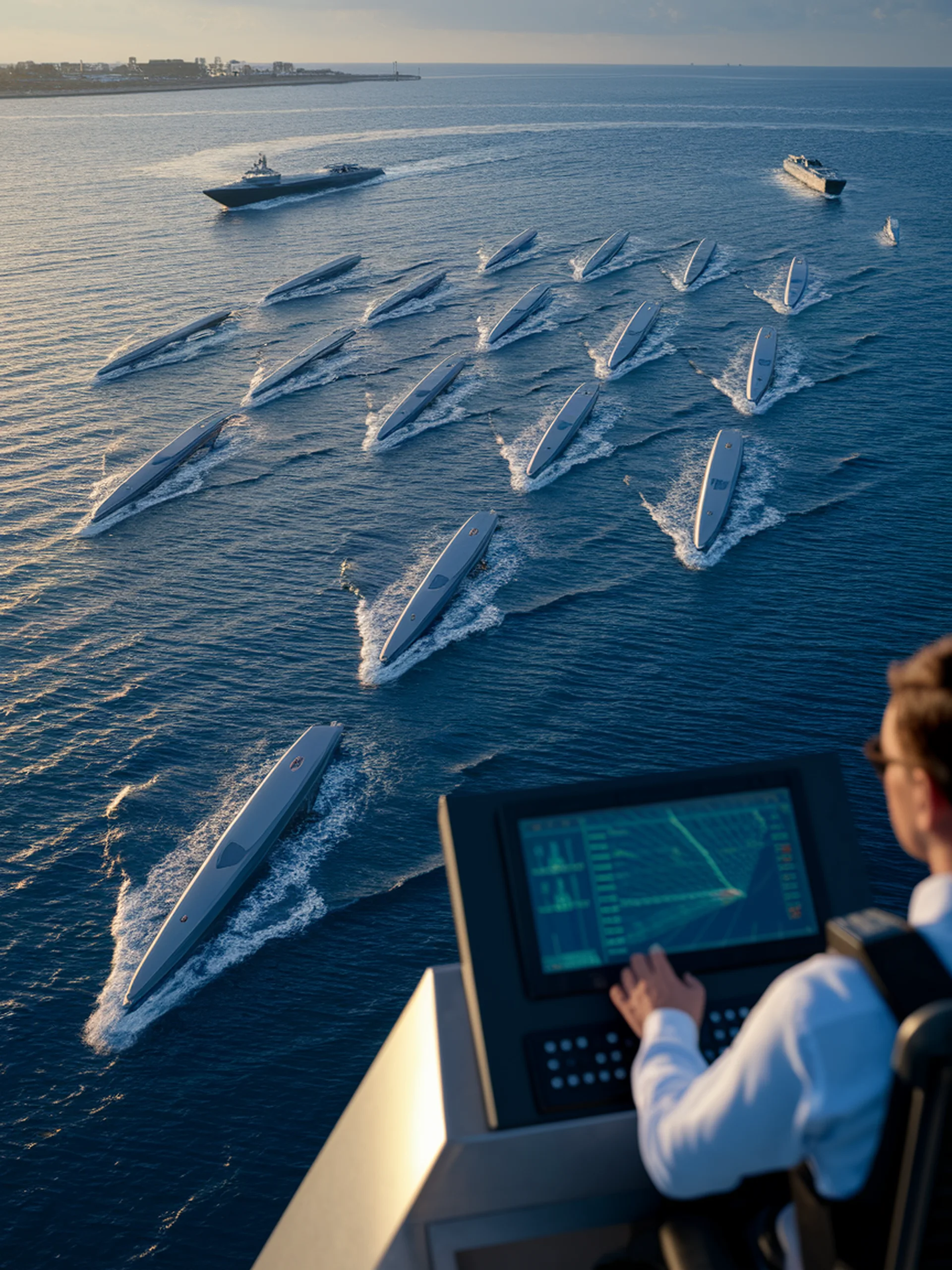
Enhancing USV Swarms with Human Preference
Aligning Multi-Agent Systems with User Preferences for Security Operations
This research introduces a novel approach to fine-tune multi-agent reinforcement learning systems for unmanned surface vehicle (USV) swarms based on human implicit preferences, improving their effectiveness in security applications.
- Addresses the challenge of encoding expert intuition into reward functions for complex multi-agent systems
- Implements Reinforcement Learning with Human Feedback (RLHF) specifically for Multi-Agent Reinforcement Learning scenarios
- Enhances USV swarm performance for critical security applications including surveillance and vessel protection
- Demonstrates a practical method to align autonomous system behavior with human operational preferences
This advancement matters for security applications by enabling more intuitive control of autonomous vehicle swarms in maritime defense, surveillance, and protection operations, potentially improving mission success rates while reducing the expertise barrier for deployment.