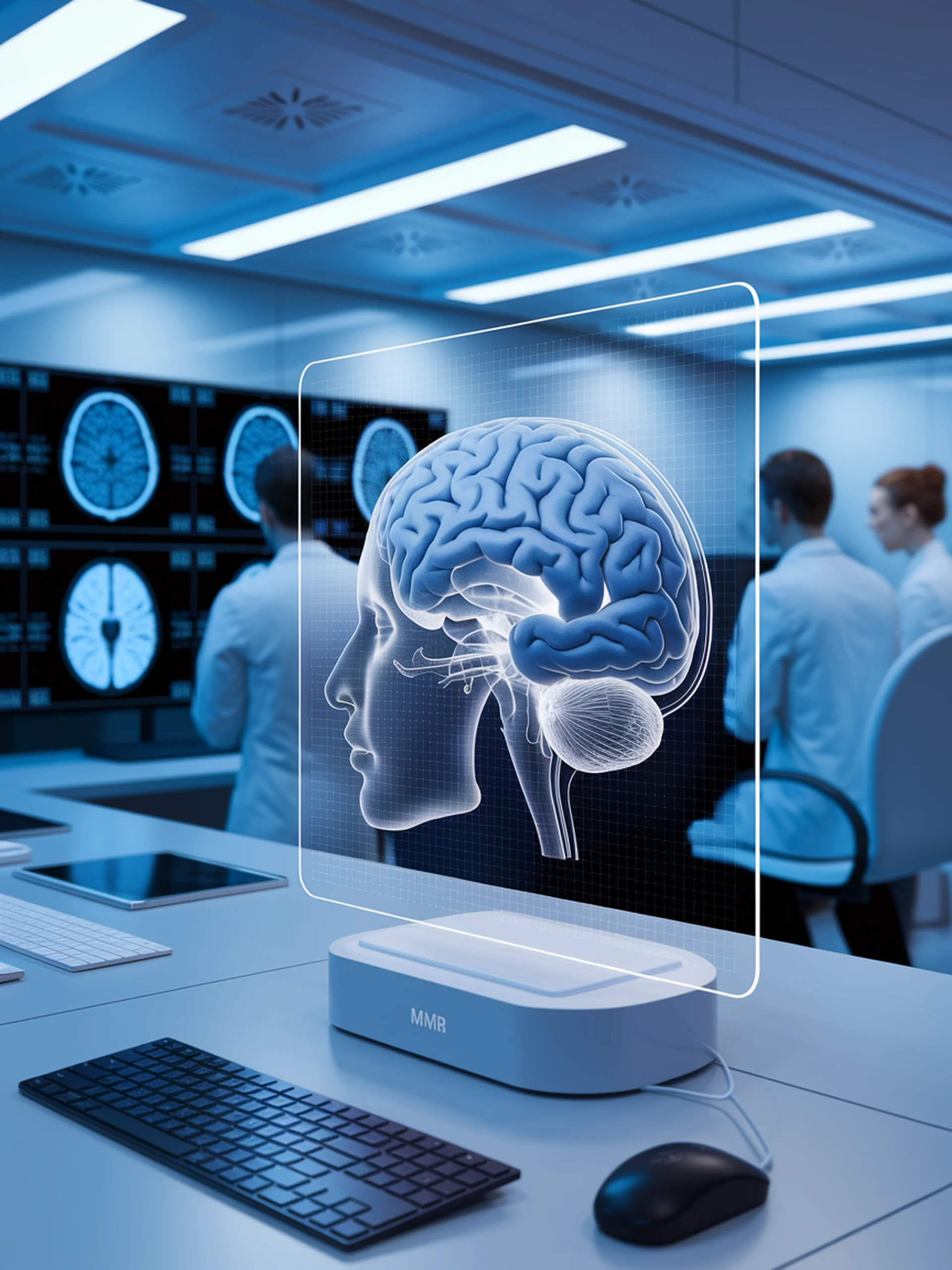
Unlocking 3D Medical Imaging with Self-Supervised Learning
Advancing MAE pre-training for more accurate medical image segmentation
This research demonstrates how Masked Autoencoders (MAE) can be optimized for 3D medical image segmentation, overcoming key limitations in current approaches.
- Addresses three critical pitfalls: small pre-training datasets, inadequate 3D architectures, and insufficient evaluation protocols
- Achieves superior segmentation accuracy on brain MRI volumes
- Demonstrates effective knowledge transfer from unlabeled to labeled medical imaging data
- Provides a framework for leveraging vast untapped clinical datasets
This advancement matters because it helps unlock the potential of extensive unlabeled medical imaging data, potentially reducing the need for manual annotation while improving diagnostic capabilities in clinical settings.
Revisiting MAE pre-training for 3D medical image segmentation