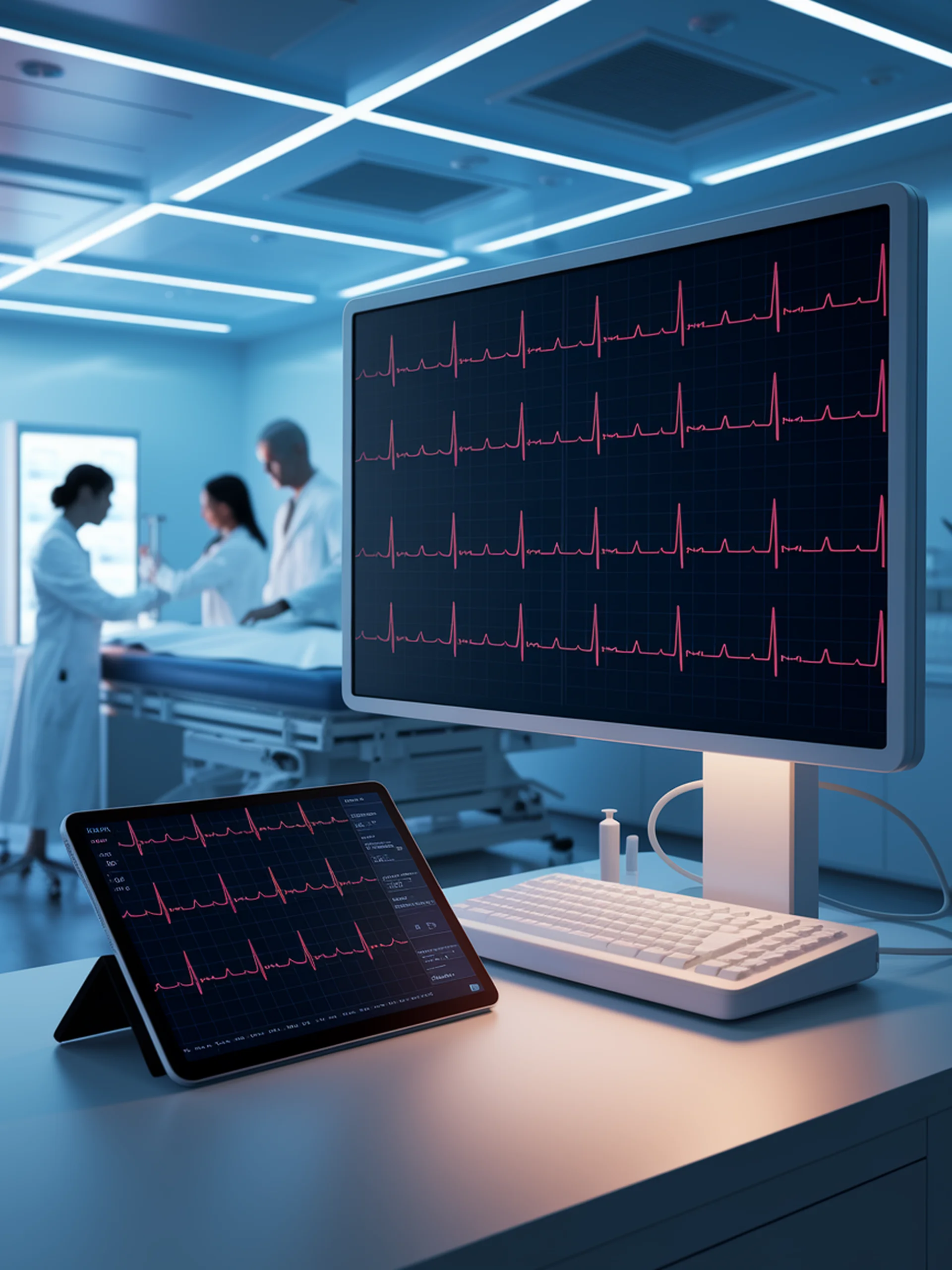
GEM: Next-Generation ECG Interpretation
Advancing healthcare with multimodal AI that grounds diagnoses in ECG evidence
GEM is the first multimodal large language model that unifies ECG time series data, 12-lead ECG images, and text for improved diagnostic accuracy and explainability.
- Solves key limitations in current ECG AI through enhanced multimodal synergy between signal data and visual representations
- Enables feature-grounded analysis by linking diagnoses directly to specific waveform evidence
- Provides clinician-aligned interpretations that match how medical professionals analyze ECGs
- Improves diagnostic transparency critical for clinical adoption and trust
This breakthrough matters because it addresses a fundamental gap in medical AI: the need for explainable, evidence-based diagnostic tools that integrate seamlessly with clinical workflows and decision-making processes.
Original Paper: GEM: Empowering MLLM for Grounded ECG Understanding with Time Series and Images