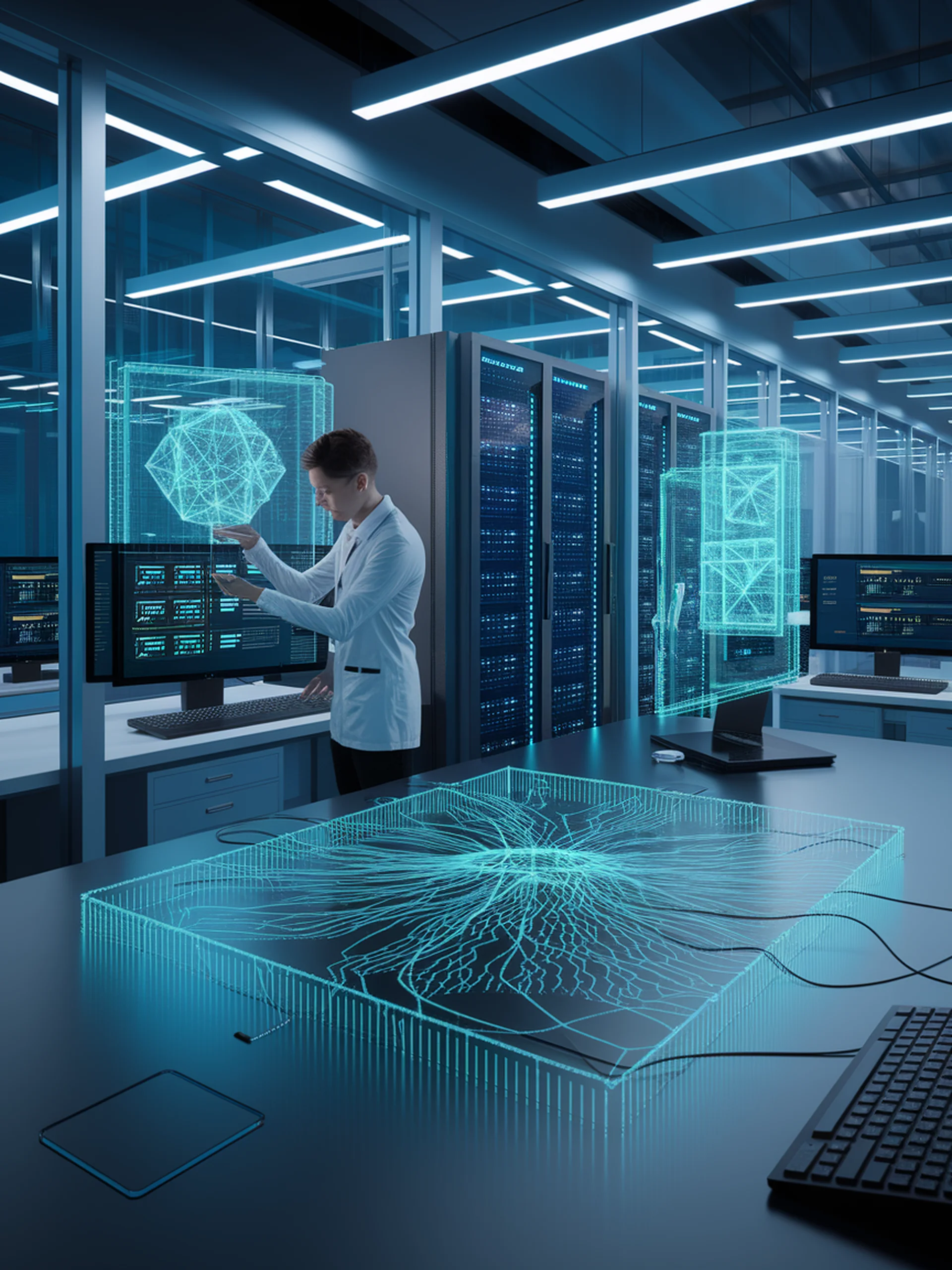
Safeguarding AI Evaluation Integrity
Detecting benchmark contamination with innovative watermarking techniques
Researchers introduce a novel watermarking method to detect when large language models have been inappropriately trained on test benchmarks, addressing a critical integrity issue in AI evaluation.
- Uses watermarked LLMs to reformulate benchmark questions while preserving their utility
- Detects "radioactivity" (traces of watermarking) in model outputs
- Provides a practical solution to validate evaluation integrity in AI systems
- Establishes a security mechanism to ensure fair comparisons between competing models
This research is vital for maintaining trust in AI benchmarking systems and preventing models from gaining unfair advantages through test data contamination. The approach helps security professionals verify that performance claims are legitimate and based on genuine generalization abilities rather than memorization.
Original Paper: Detecting Benchmark Contamination Through Watermarking