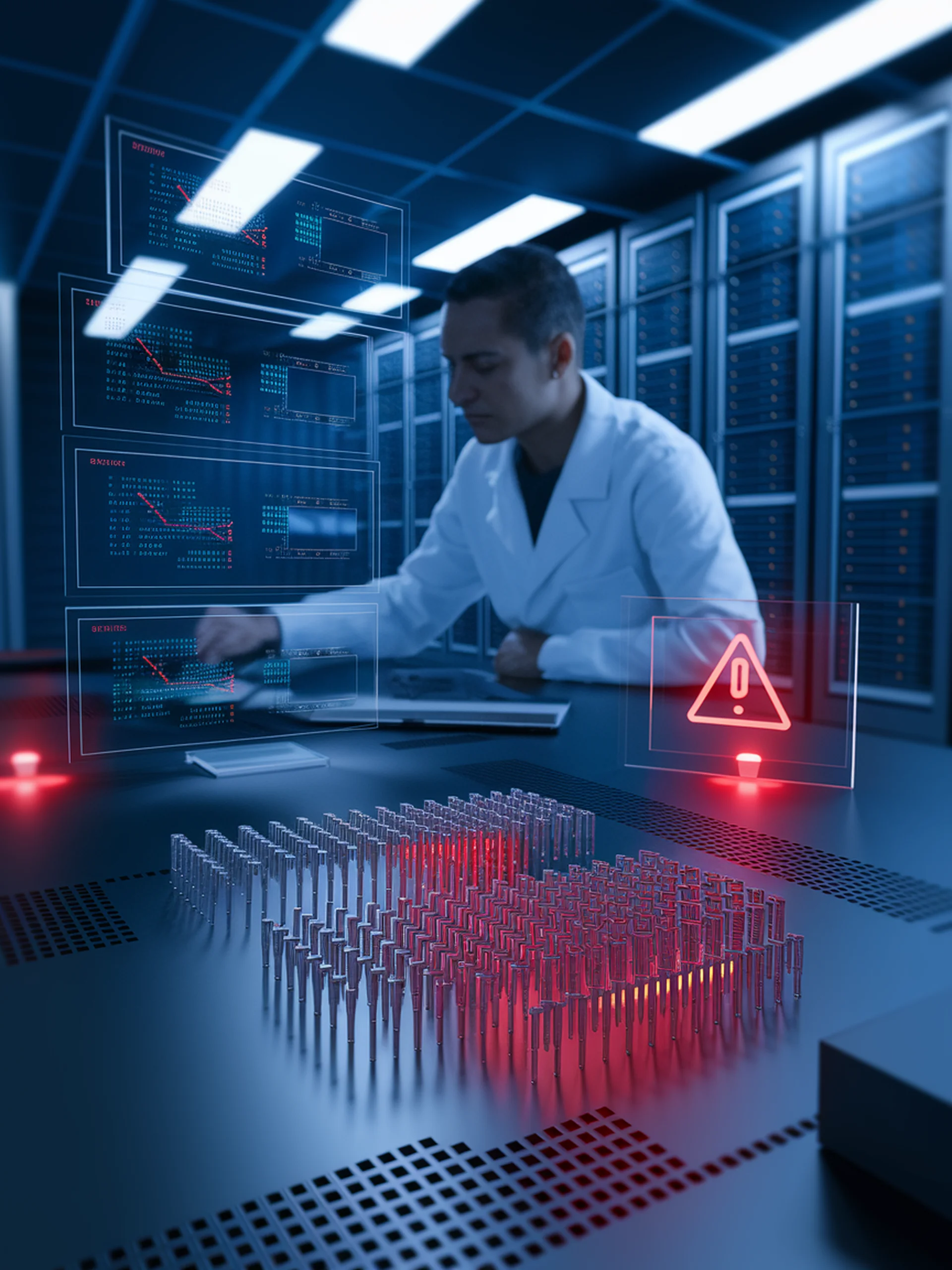
Poison Pills in LLMs: Hidden Vulnerabilities
How targeted data poisoning compromises AI security
This research reveals how poison pill attacks can manipulate specific knowledge in large language models while preserving overall model performance.
Key findings:
- Achieved 54.6% increased retrieval inaccuracy on long-tail knowledge vs. dominant topics
- Compressed models showed 25.5% greater vulnerability than original architectures
- Attacks exploit inherent architectural properties of LLMs
- Vulnerability disparities exist across different model configurations
Security Implications: These findings are critical for AI security as they demonstrate how targeted data poisoning can selectively corrupt factual information without noticeably degrading overall model utility, making such attacks hard to detect through standard quality checks.
Swallowing the Poison Pills: Insights from Vulnerability Disparity Among LLMs