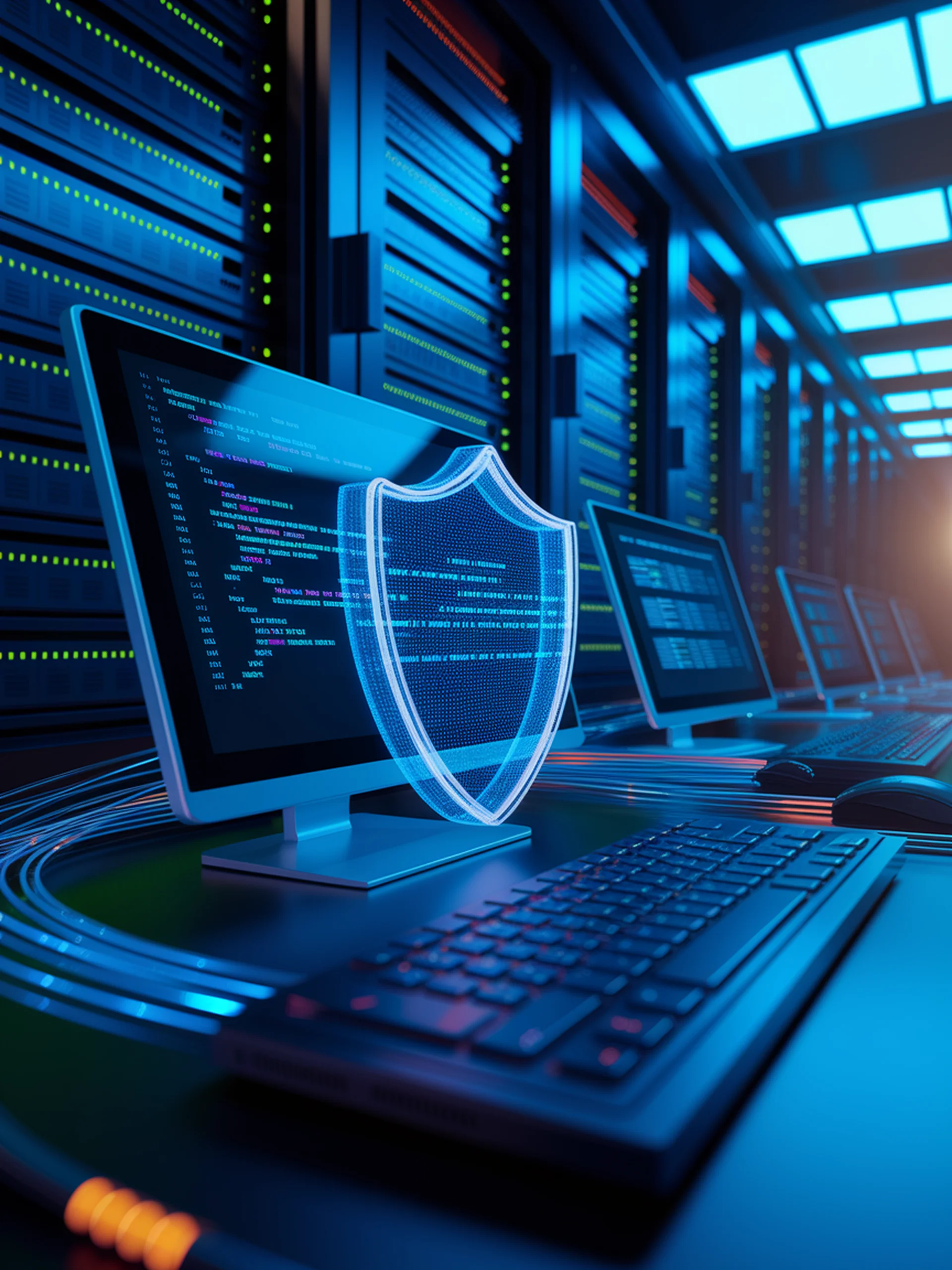
Defending Against Dead Code Poisoning
Novel detection techniques to secure code generation models
Researchers developed DePA (Deeper Perplexity Analysis), a more effective method for detecting malicious code insertions in LLM training datasets.
- Identifies dead code poisoning that traditional perplexity analysis misses
- Achieves 93% accuracy in poisoning detection through structural code analysis
- Helps protect against attacks that could bias or compromise AI code suggestions
- Addresses a critical security gap in the current ML pipeline for code-related models
This research matters because it safeguards the integrity of code generation systems that increasingly power developer tools and automated programming environments, preventing attackers from manipulating AI-generated code recommendations to introduce vulnerabilities.
Beyond Natural Language Perplexity: Detecting Dead Code Poisoning in Code Generation Datasets