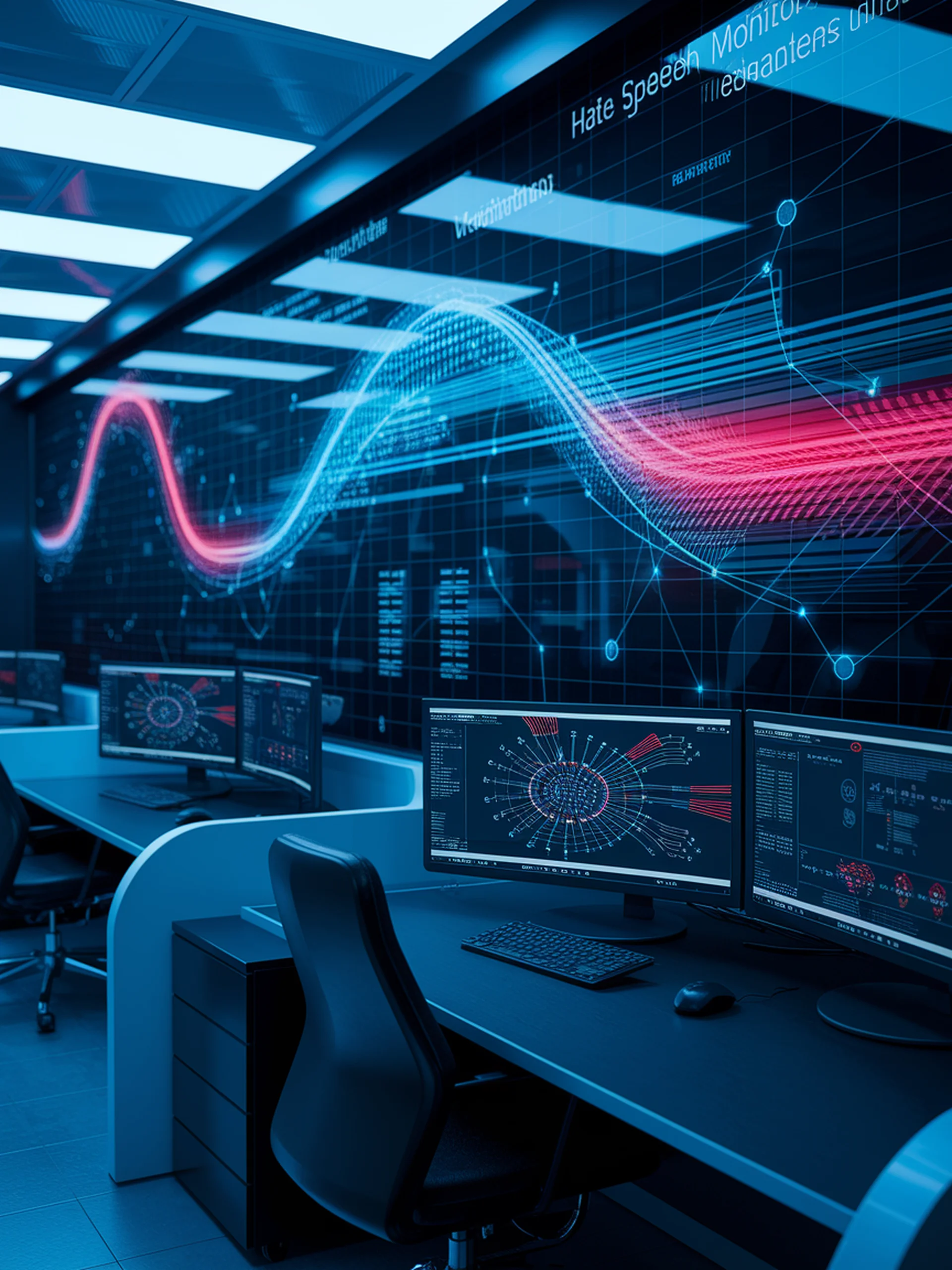
HateBench: Evaluating Hate Speech Detection Against LLM Threats
First comprehensive benchmark for testing detectors against LLM-generated hate content
HateBench presents a novel framework for evaluating hate speech detectors against increasingly sophisticated LLM-generated content, revealing critical security gaps in current detection systems.
- Testing across 7,838 hate speech samples from six popular LLMs exposed significant detection weaknesses
- Most detectors perform poorly against subtle LLM-generated hate speech and coordinated hate campaigns
- Current systems show high vulnerability to adversarial attacks, with some detectors dropping to near-zero effectiveness
- Framework provides a standardized testing approach to improve detector robustness against evolving threats
This research is crucial for security professionals as it highlights urgent needs to fortify defenses against LLM-powered hate campaigns and provides a systematic way to evaluate and improve protective measures.
HateBench: Benchmarking Hate Speech Detectors on LLM-Generated Content and Hate Campaigns