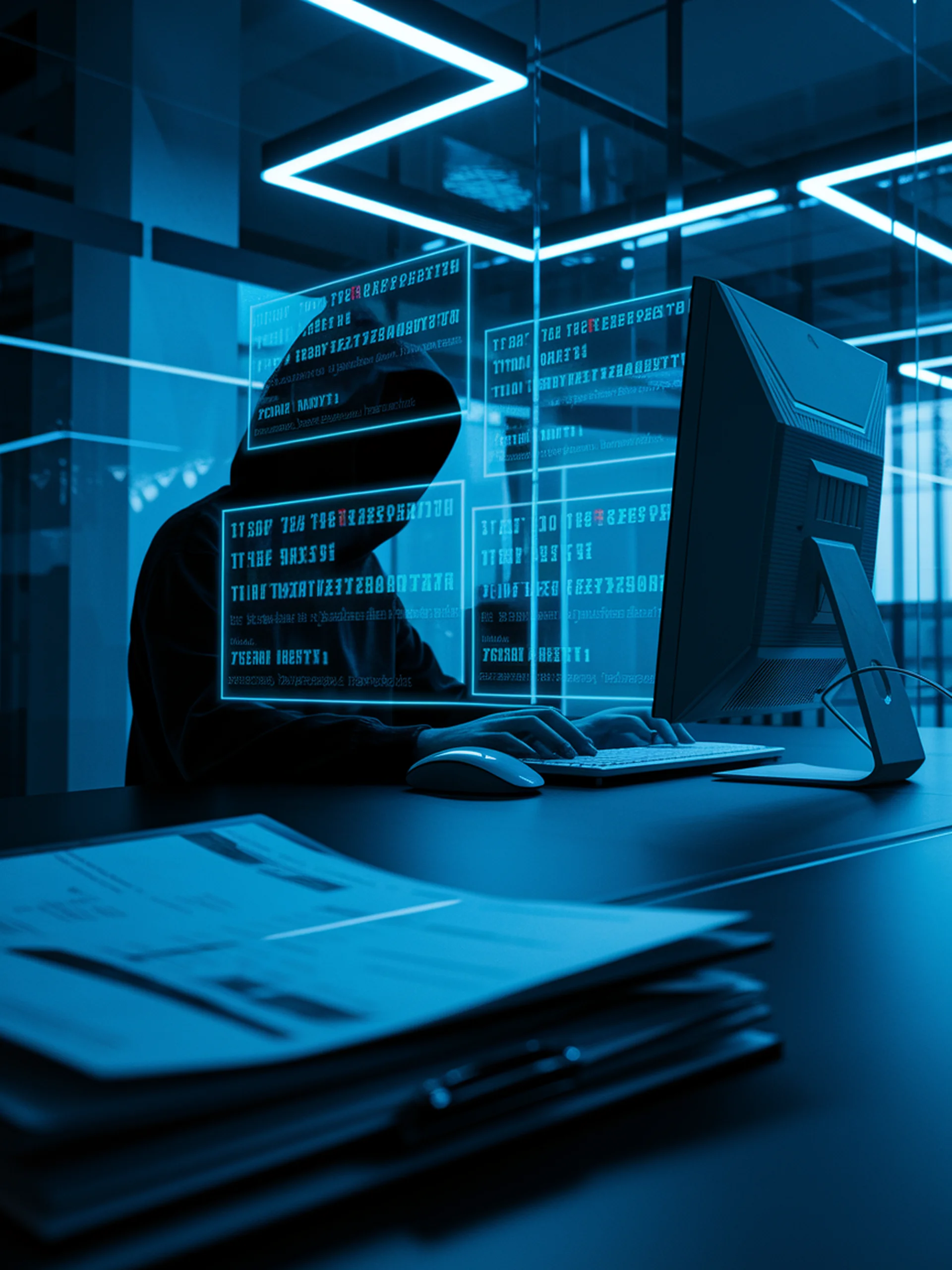
The Security Blind Spot in RAG Systems
How attackers can stealthily extract sensitive data from retrieval-augmented LLMs
This research reveals how Retrieval-Augmented Generation (RAG) systems are vulnerable to subtle membership inference attacks that can extract sensitive information from knowledge bases without detection.
- Attackers can craft natural-sounding questions that force RAG systems to reveal private data
- Even complex riddle-based queries can bypass content filters while extracting protected information
- Standard defensive measures like perplexity detection fail against these nuanced attacks
- Proposed attack methods achieved high success rates across various RAG implementations
This research matters for security professionals because it exposes critical vulnerabilities in systems many organizations rely on for knowledge management while handling sensitive data, requiring new protective measures beyond existing content filters.
Riddle Me This! Stealthy Membership Inference for Retrieval-Augmented Generation