Security in Retrieval-Augmented Generation
Research on security vulnerabilities, attack vectors, and defensive mechanisms specific to retrieval-augmented generation (RAG) systems that integrate external knowledge with LLMs
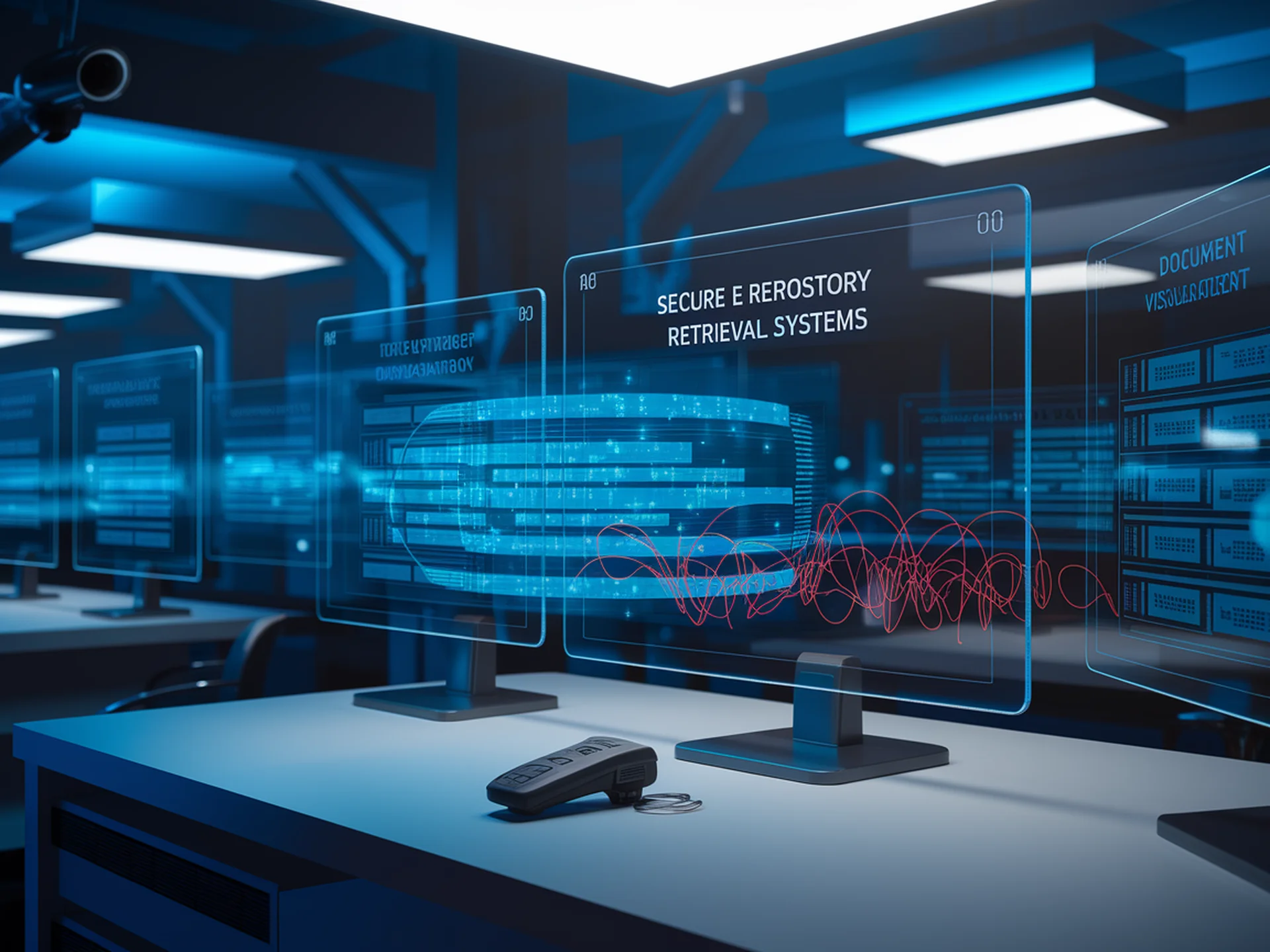
Security in Retrieval-Augmented Generation
Research on Large Language Models in Security in Retrieval-Augmented Generation
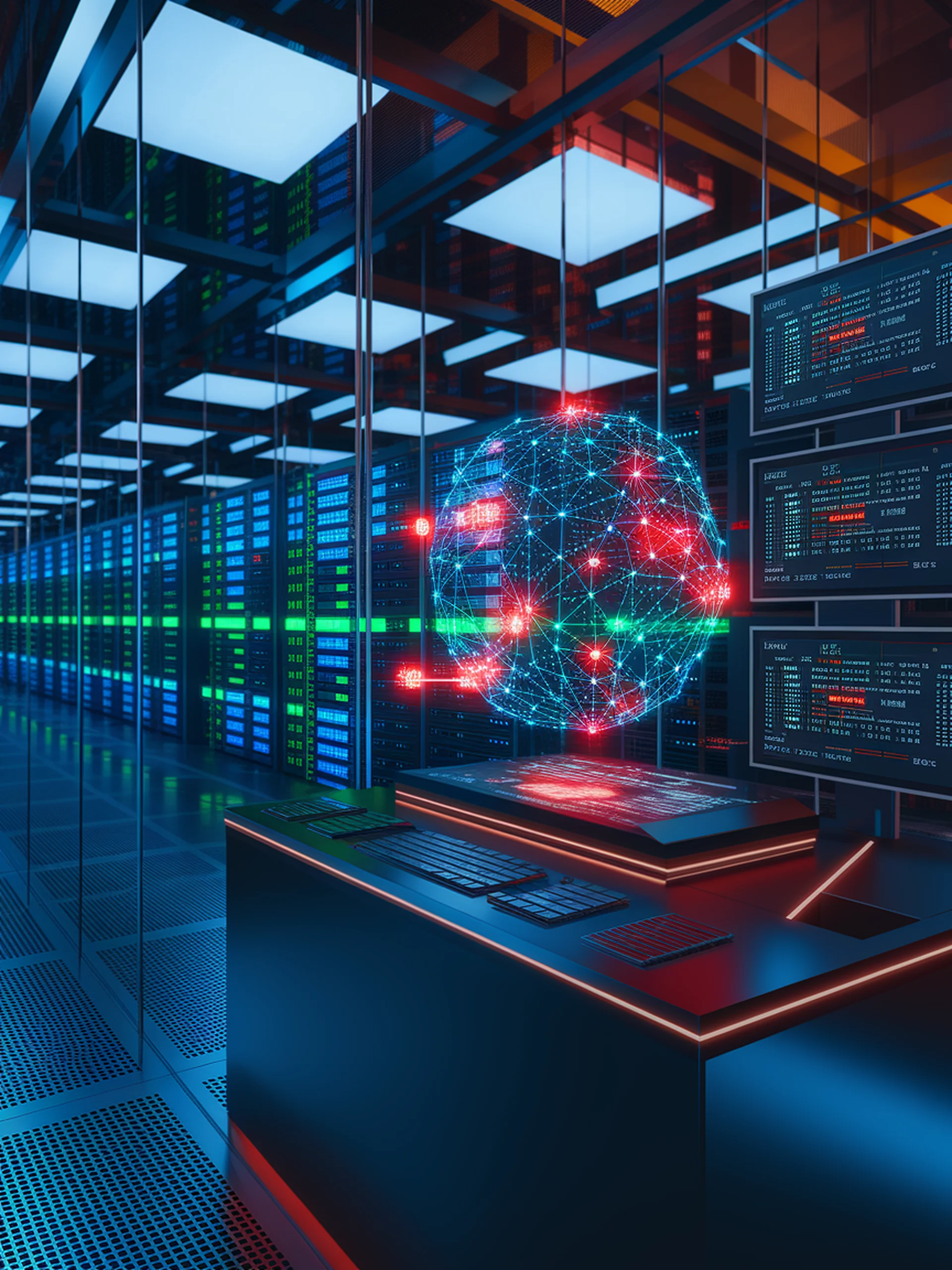
Securing the Knowledge Pipeline
Evaluating and Addressing Security Vulnerabilities in RAG Systems
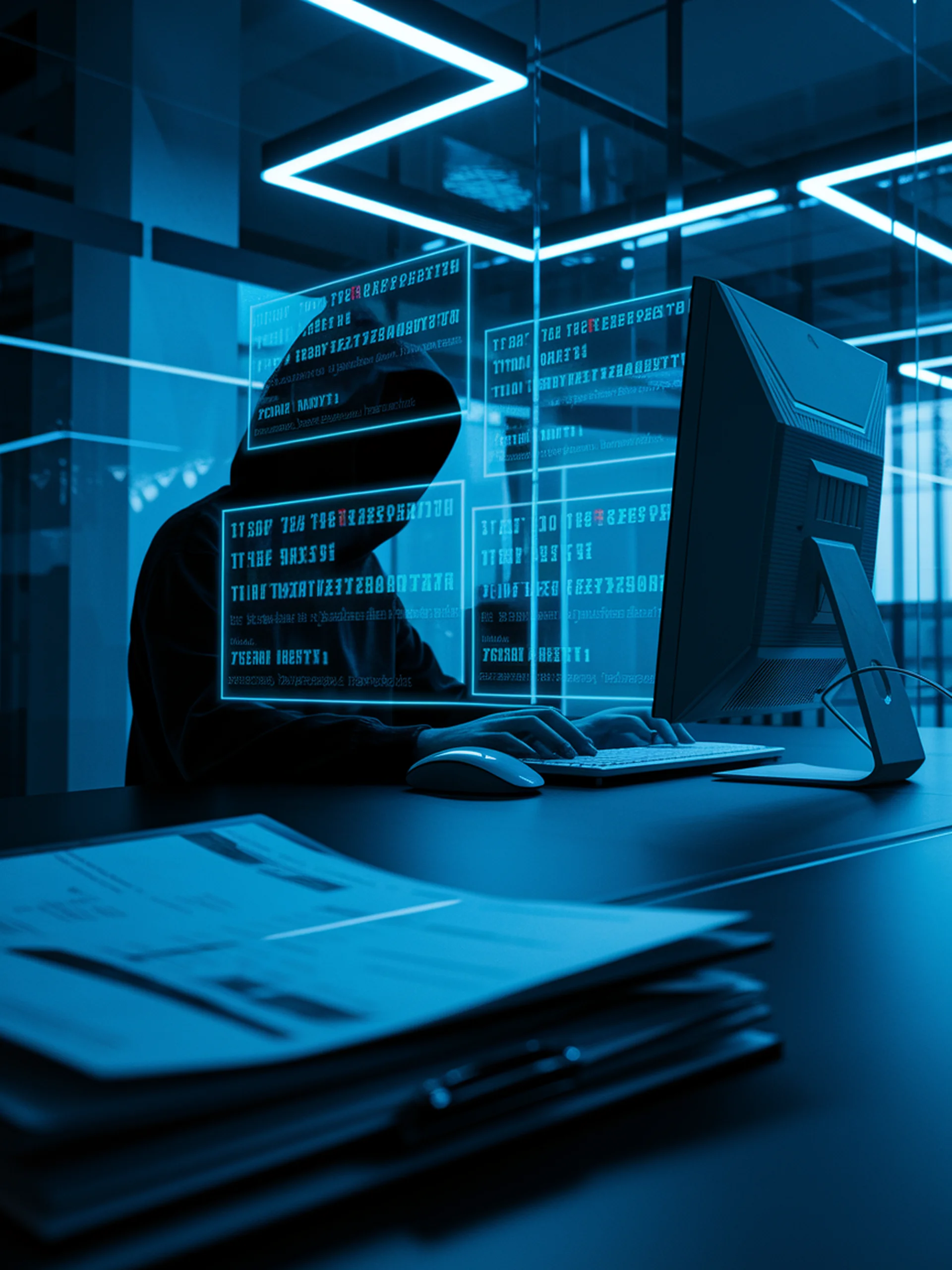
The Security Blind Spot in RAG Systems
How attackers can stealthily extract sensitive data from retrieval-augmented LLMs
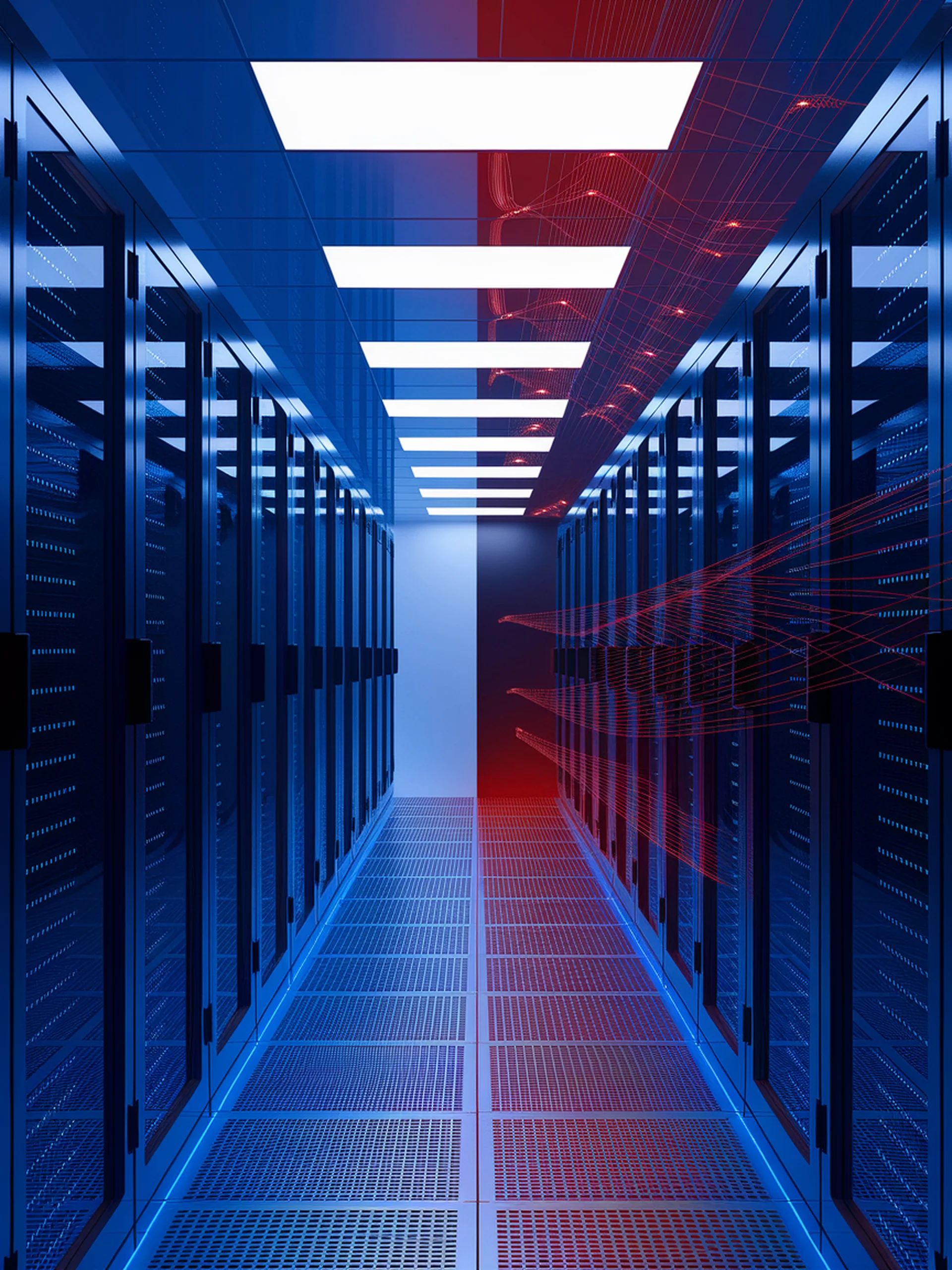
Exploiting RAG Systems: Topic-Based Opinion Manipulation
Uncovering new vulnerabilities in retrieval-augmented LLMs
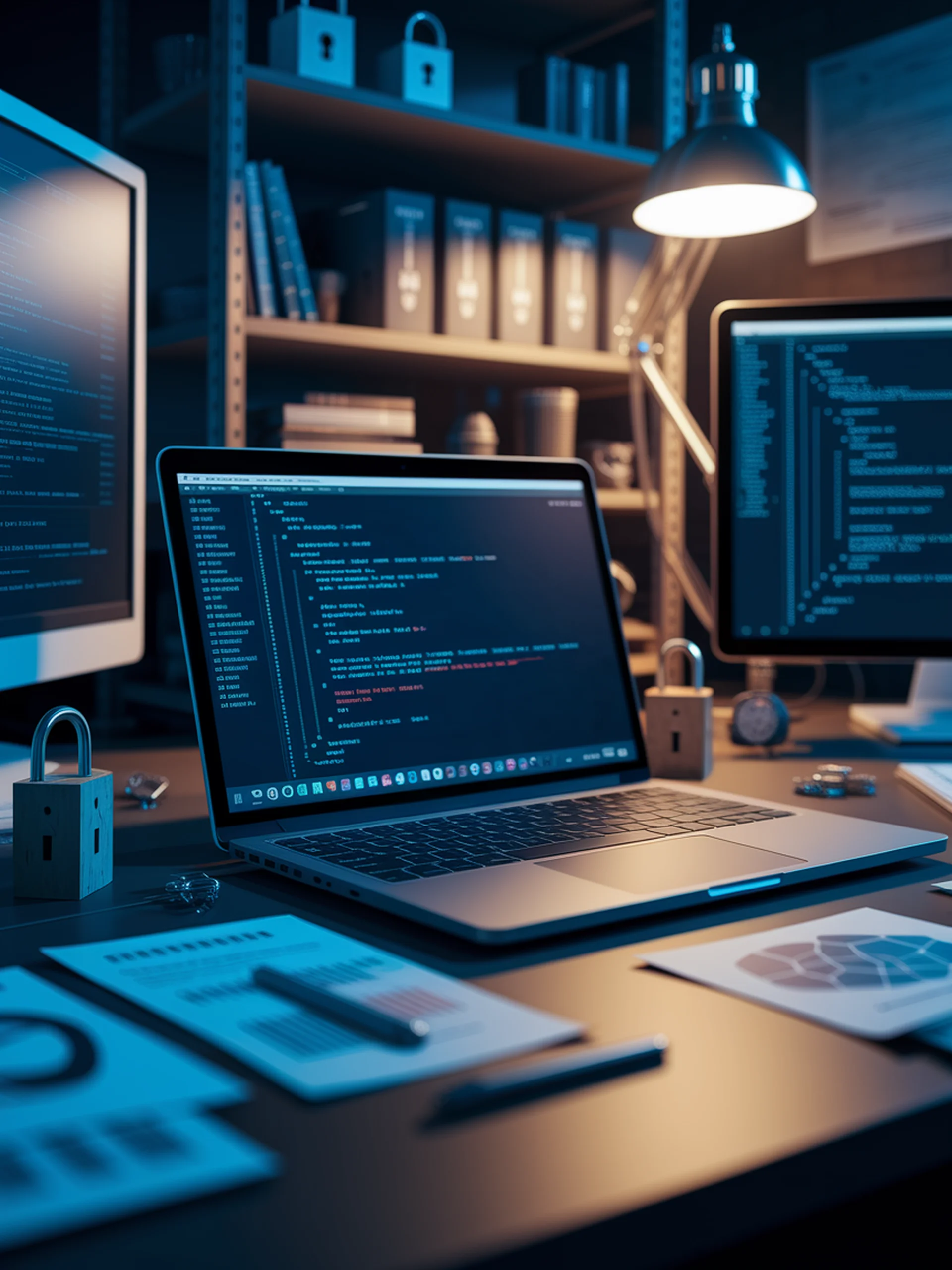
Security Vulnerabilities in AI-Assisted Code Generation
How poisoned knowledge bases compromise code security
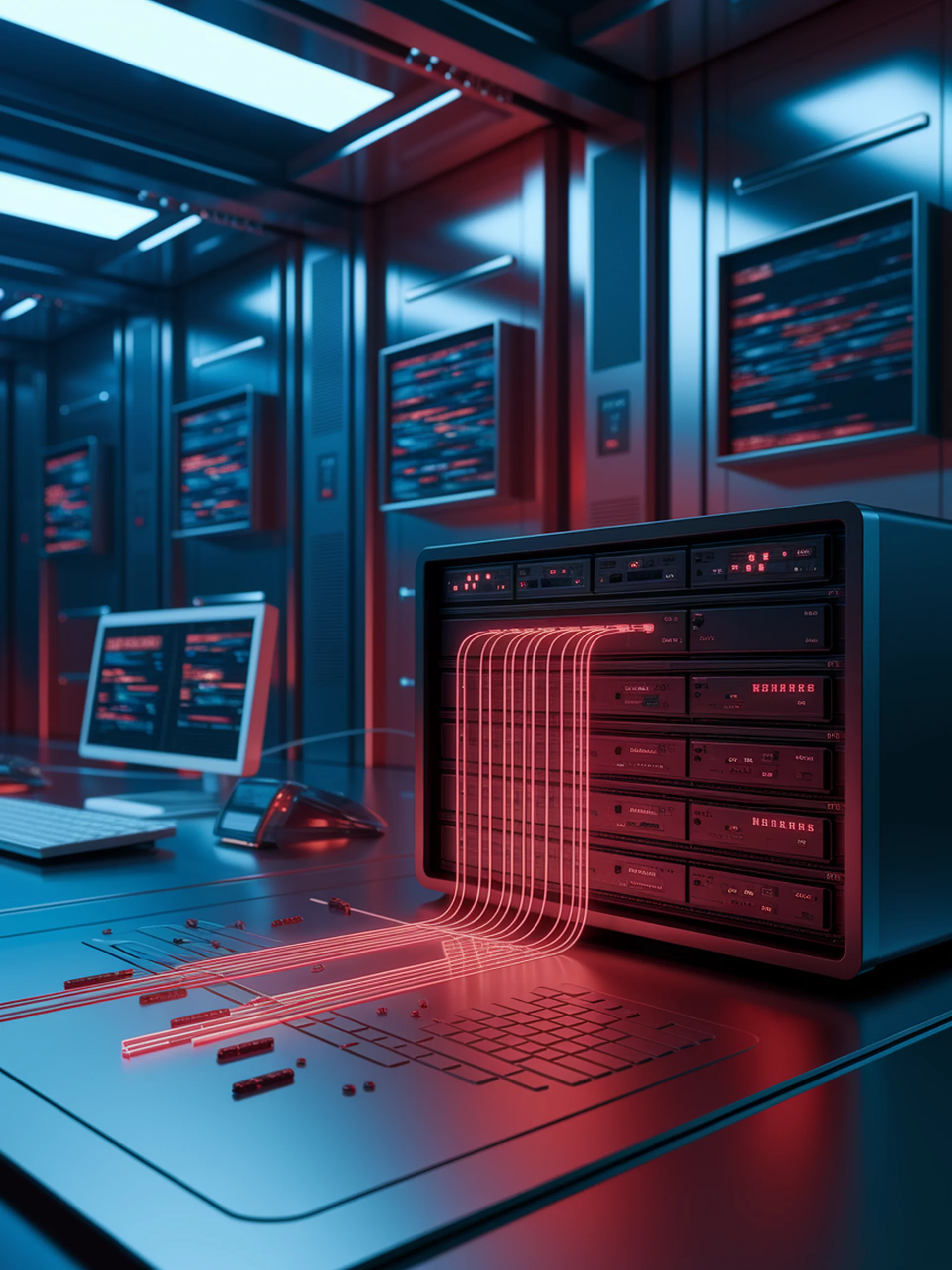
Attacking the Knowledge Base
New transferable adversarial attacks against RAG systems
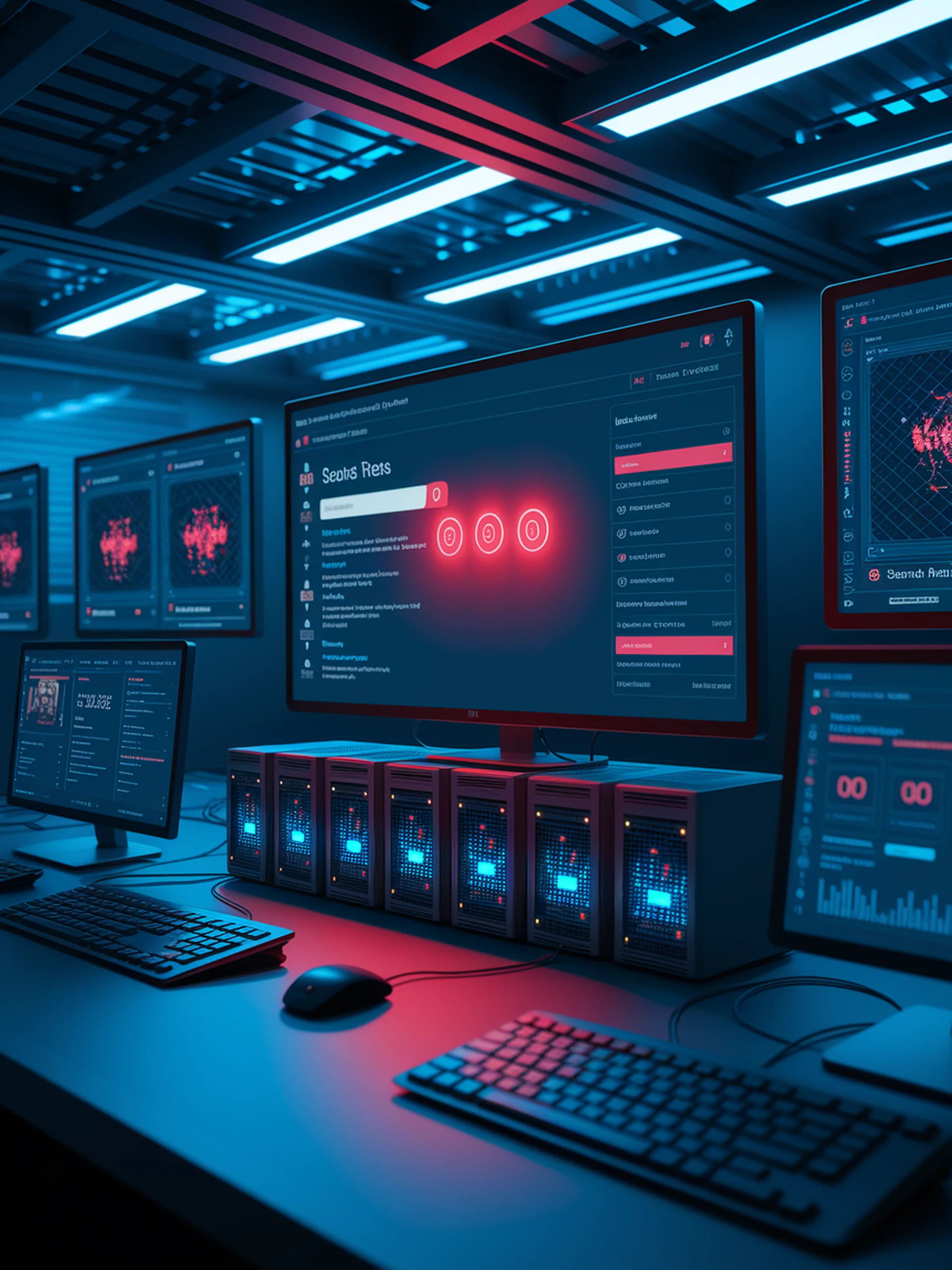
Security Vulnerabilities in AI Search Engines
Quantifying and mitigating emerging threats in AI-powered search
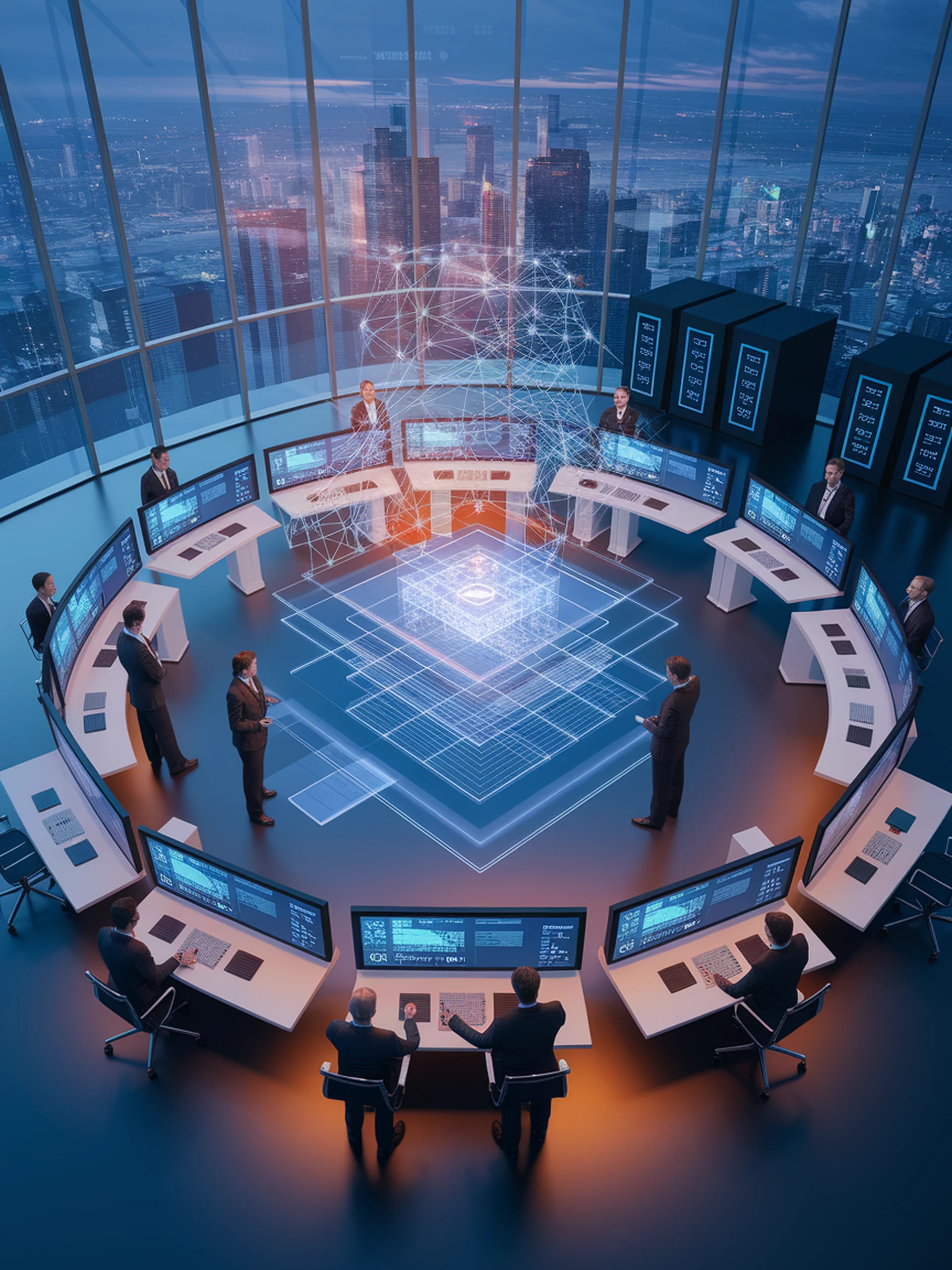
Building Trust in AI: RAG Systems
A comprehensive framework for secure Retrieval-Augmented Generation
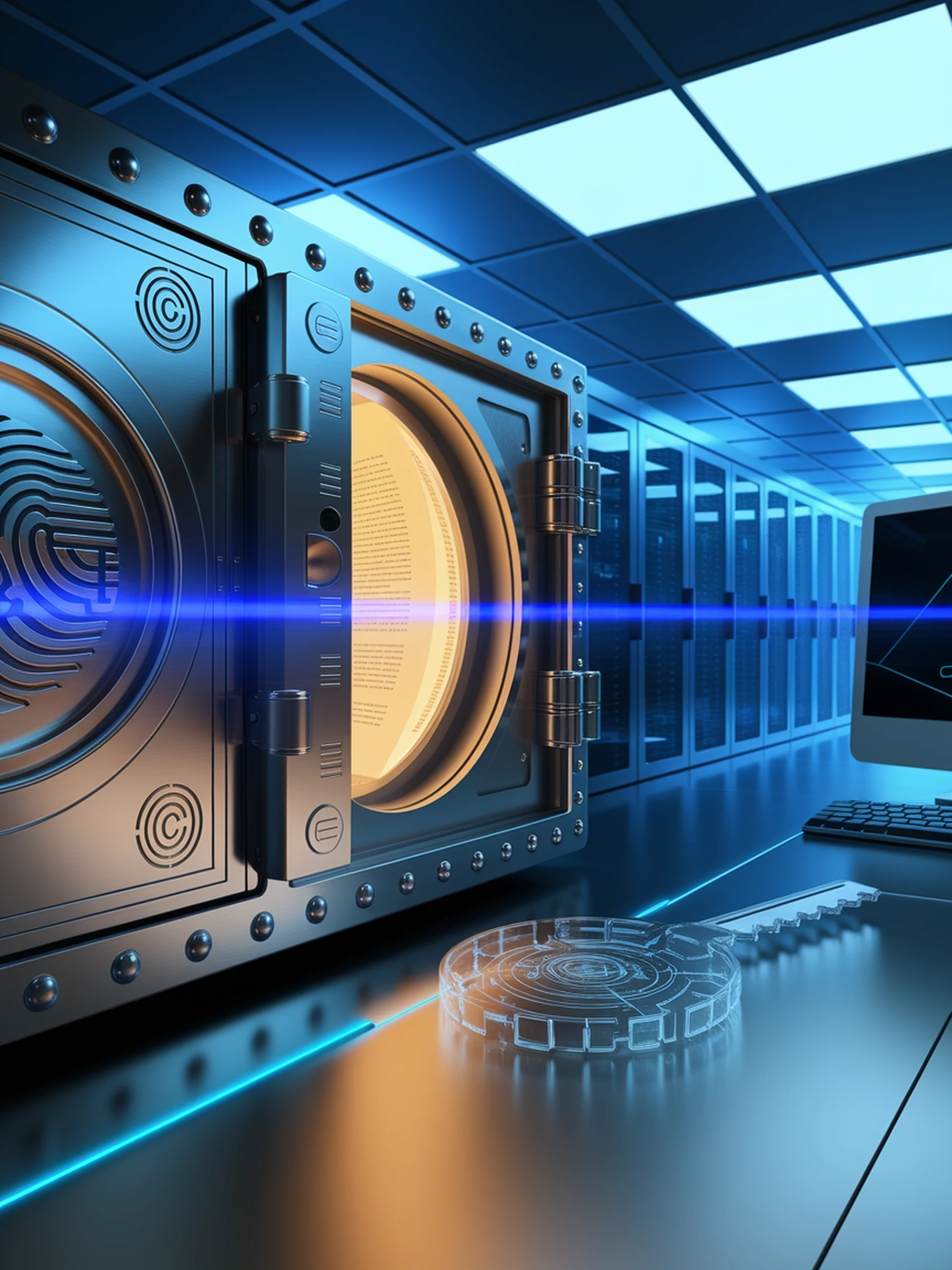
Protecting Proprietary Knowledge in RAG Systems
A copyright protection approach for retrieval-augmented LLMs
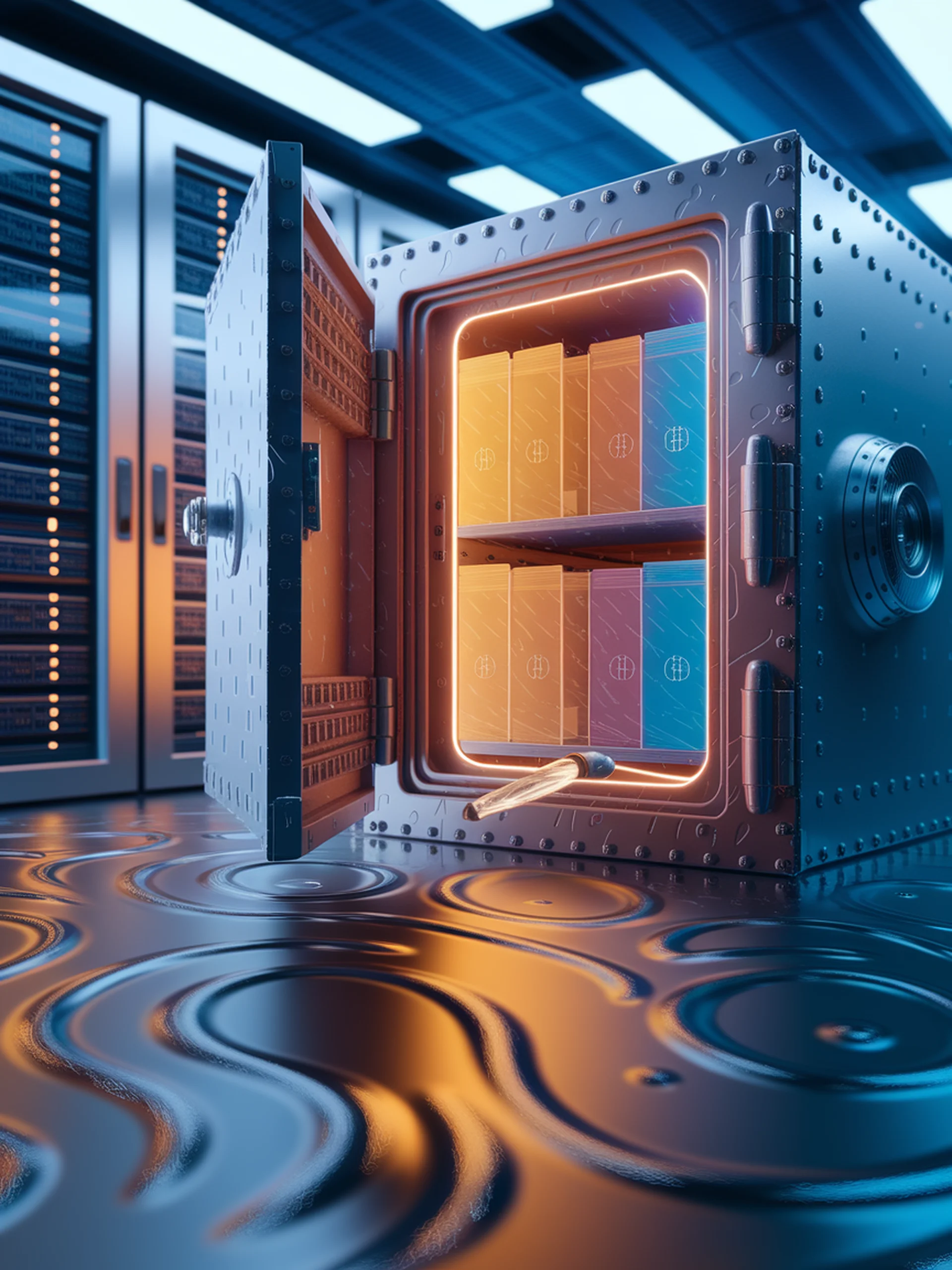
Protecting Data Ownership in RAG Systems
Watermarked Canaries: A New Defense Against IP Theft in LLMs
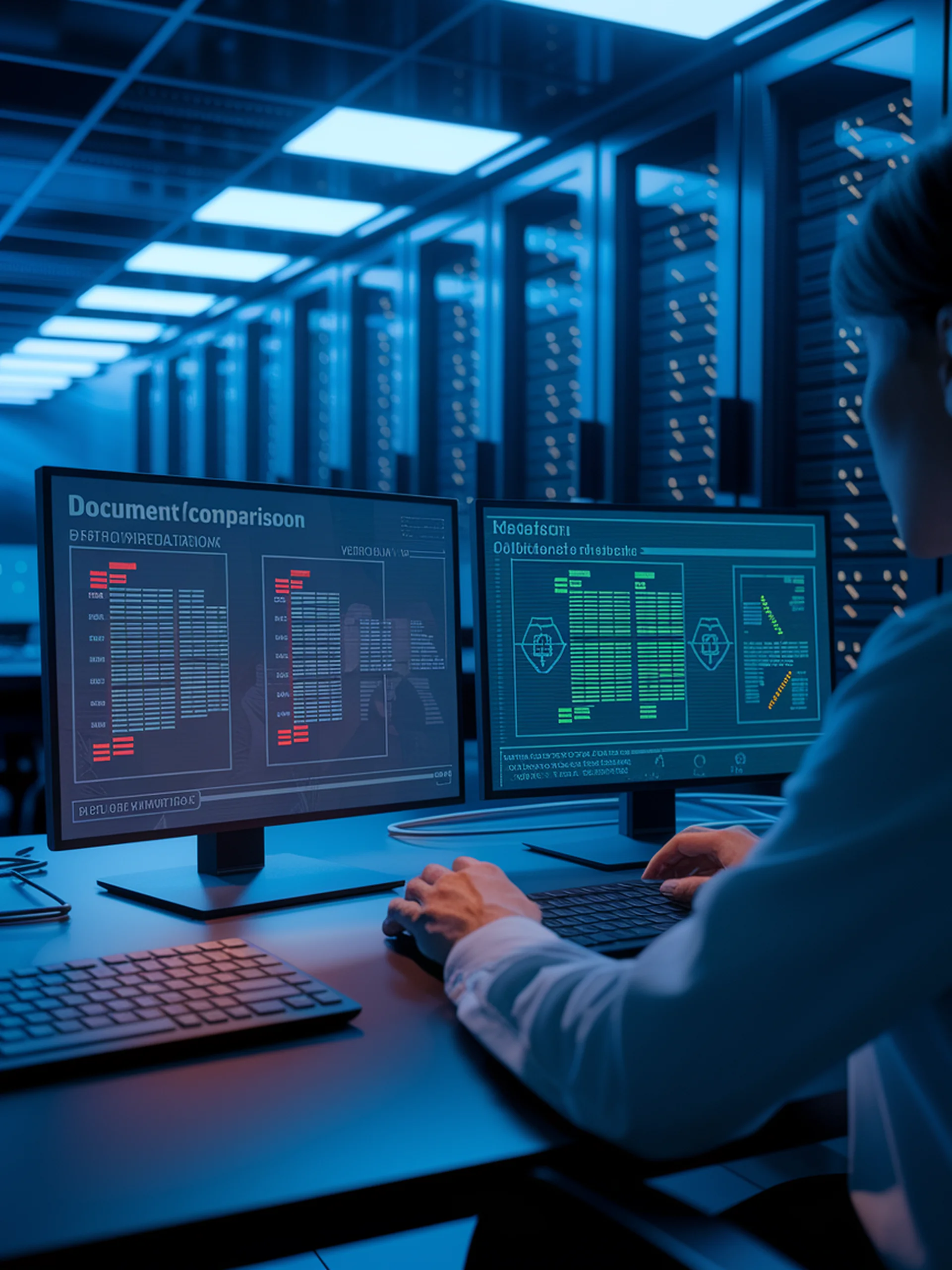
Combating Hallucinations in LLMs
A Retrieval-Augmented Approach to Detect Factual Errors
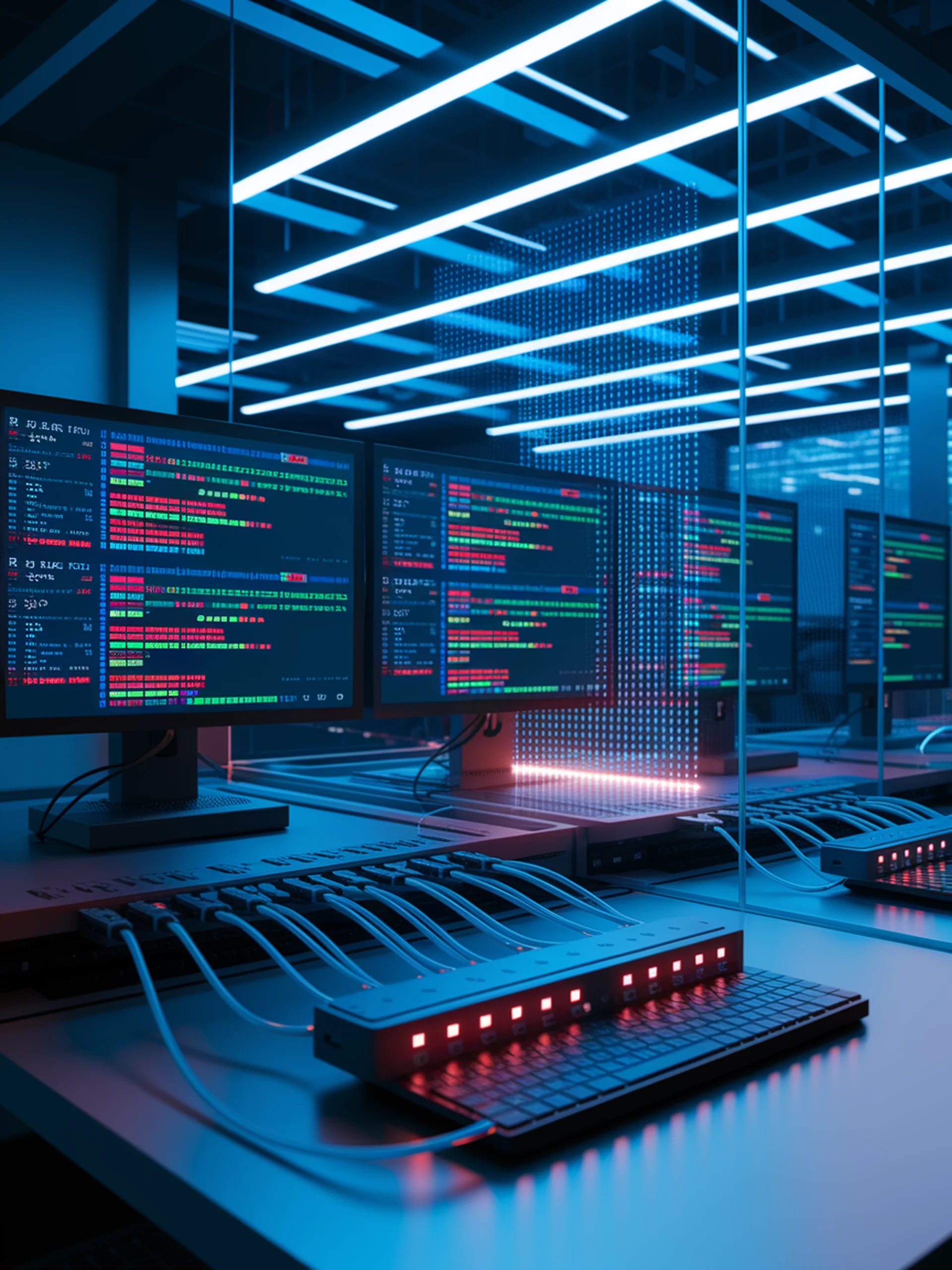
Enhancing Network Fuzzing with LLM Agents
RAG-based LLMs with Chain-of-Thought for Superior Protocol Security Testing
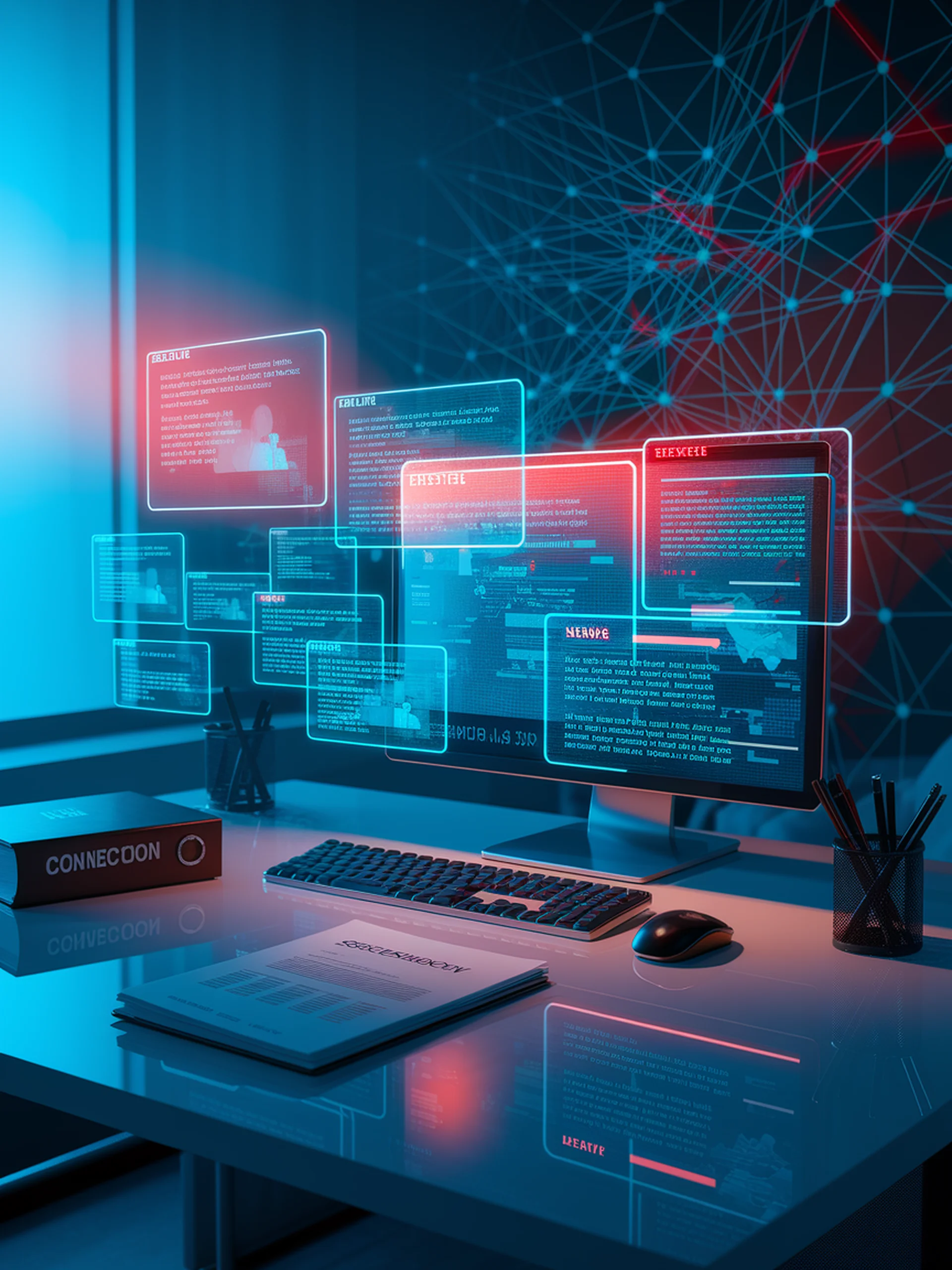
When RAG Goes Wrong: The Danger of Misleading Retrievals
Evaluating RAG's vulnerability to misinformation with RAGuard
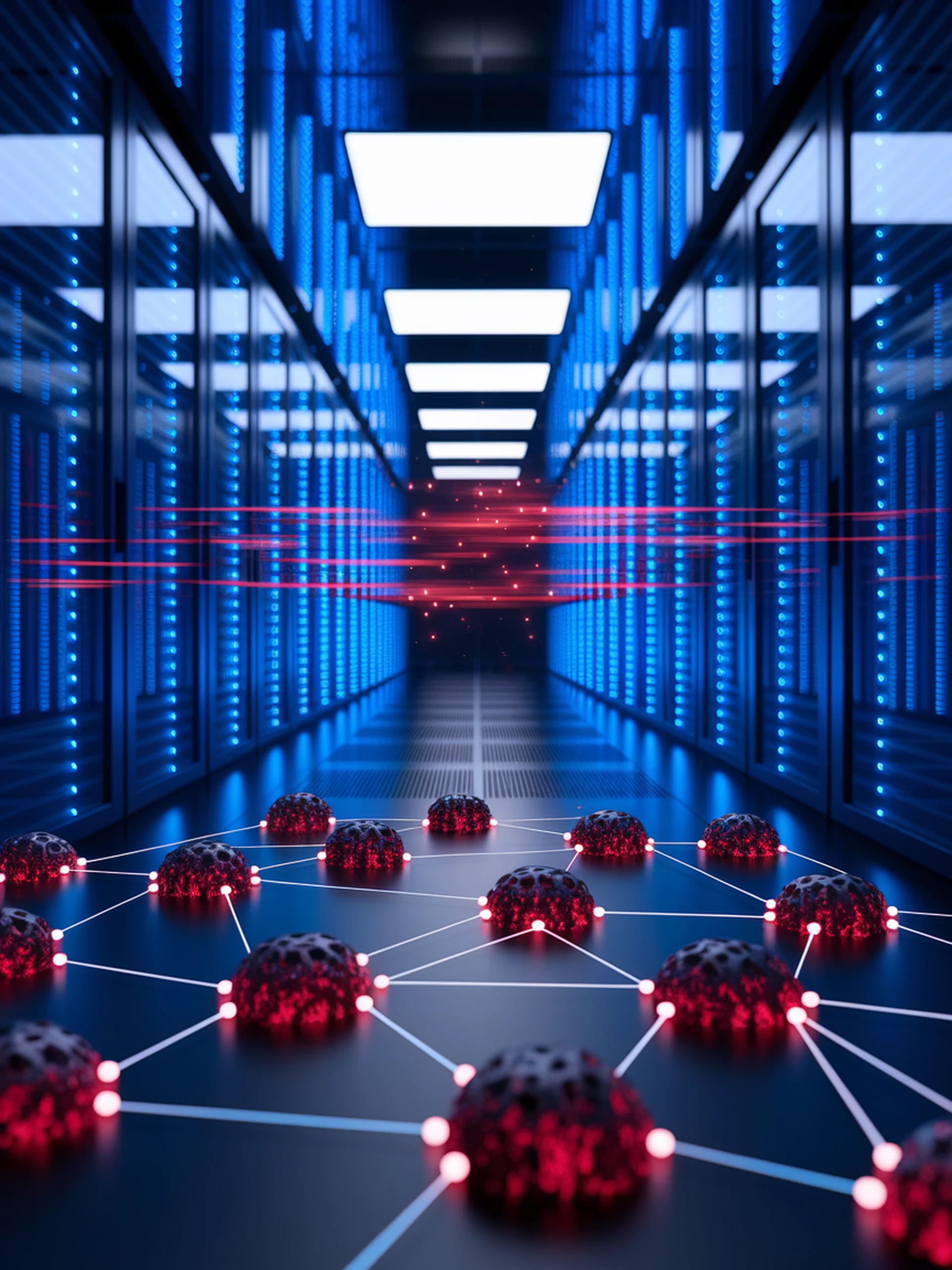
Poisoning Attacks Against Multimodal RAG
How attackers can manipulate MLLMs through knowledge poisoning
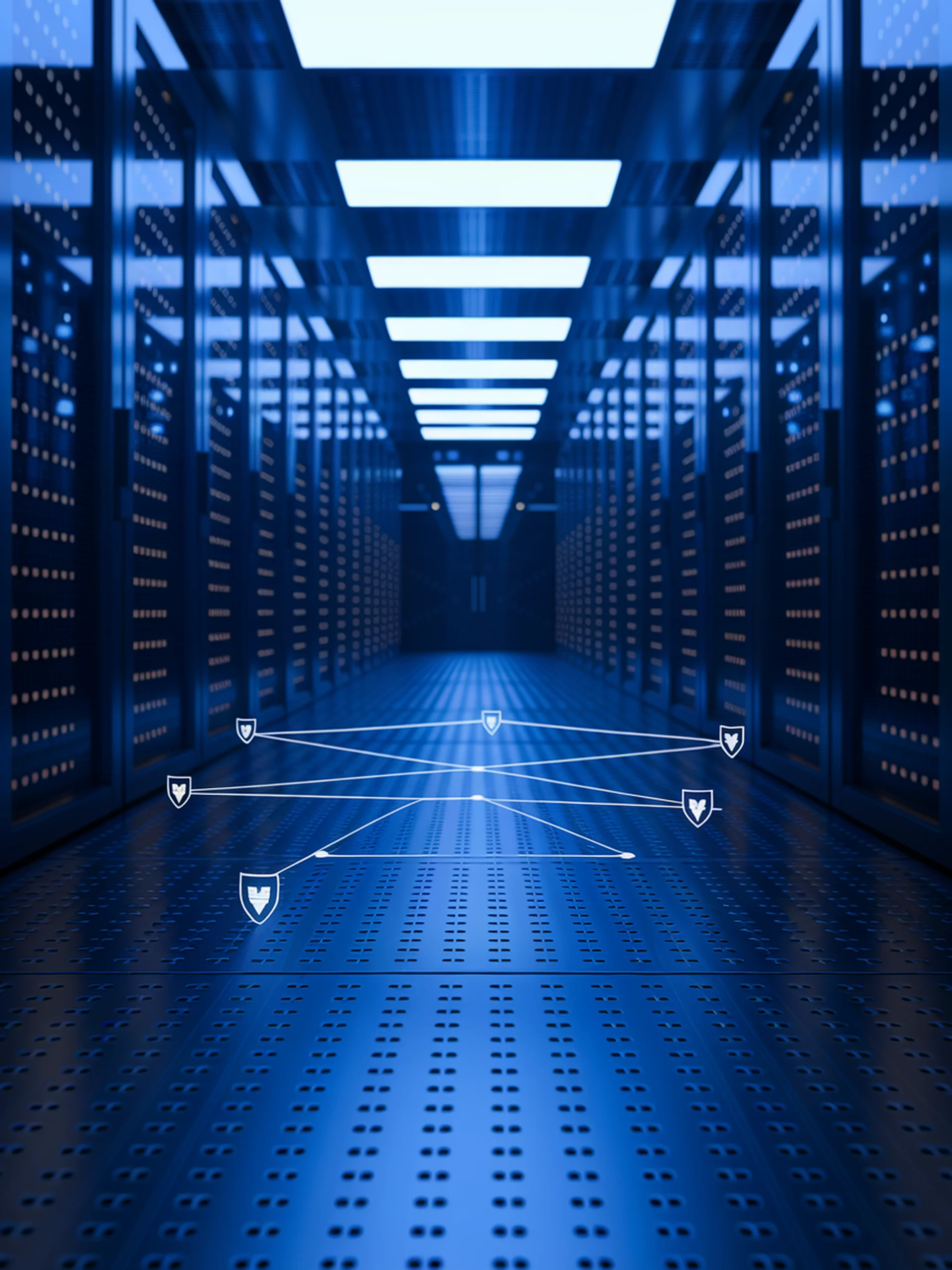
Vector Database Testing: The Security Imperative
Building a roadmap for reliable AI infrastructure through 2030
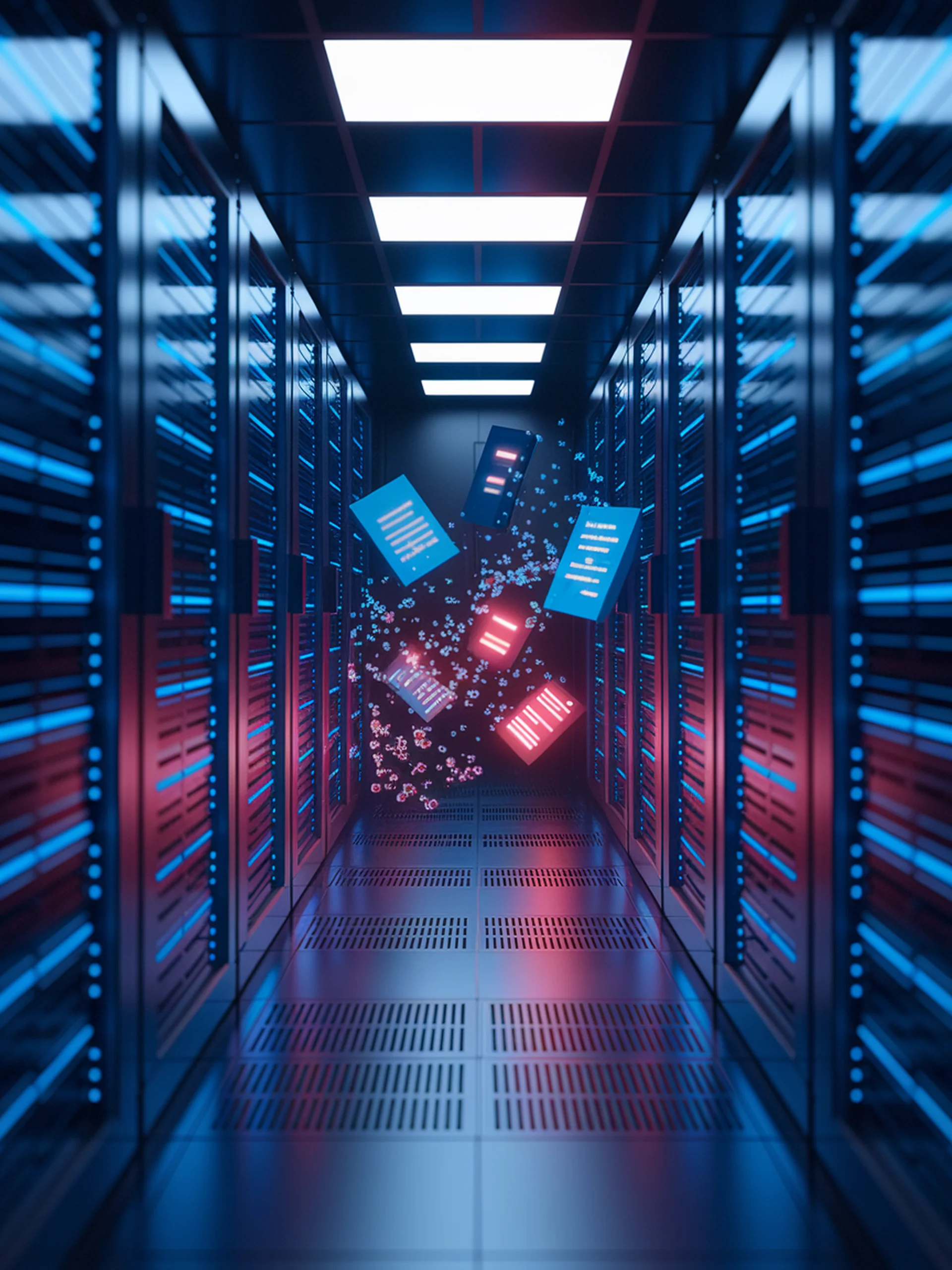
Exploiting RAG Systems: The CtrlRAG Attack
A novel black-box adversarial attack method targeting retrieval-augmented LLMs
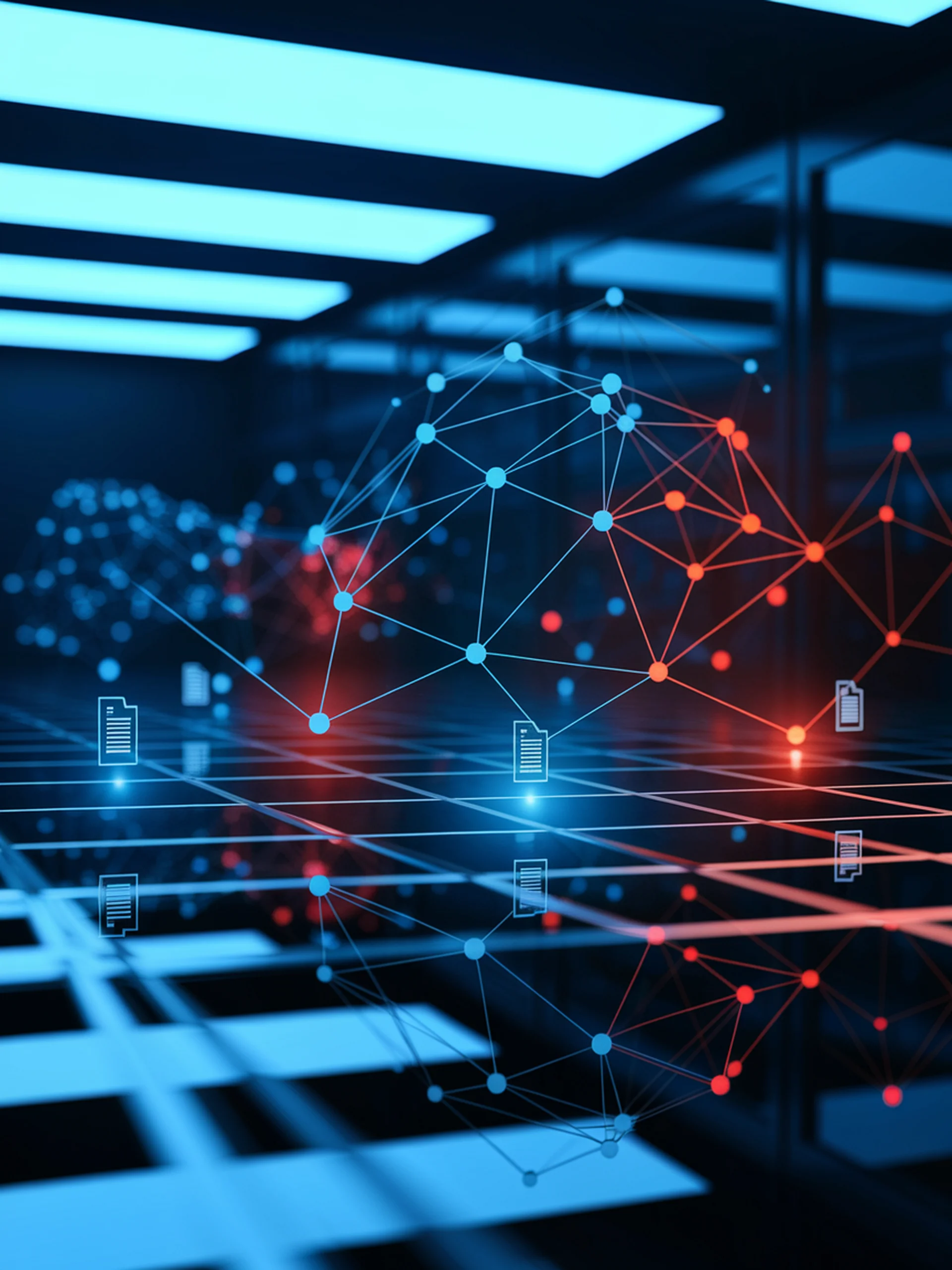
Trust Propagation in RAG Systems
A PageRank-inspired approach to combat misinformation
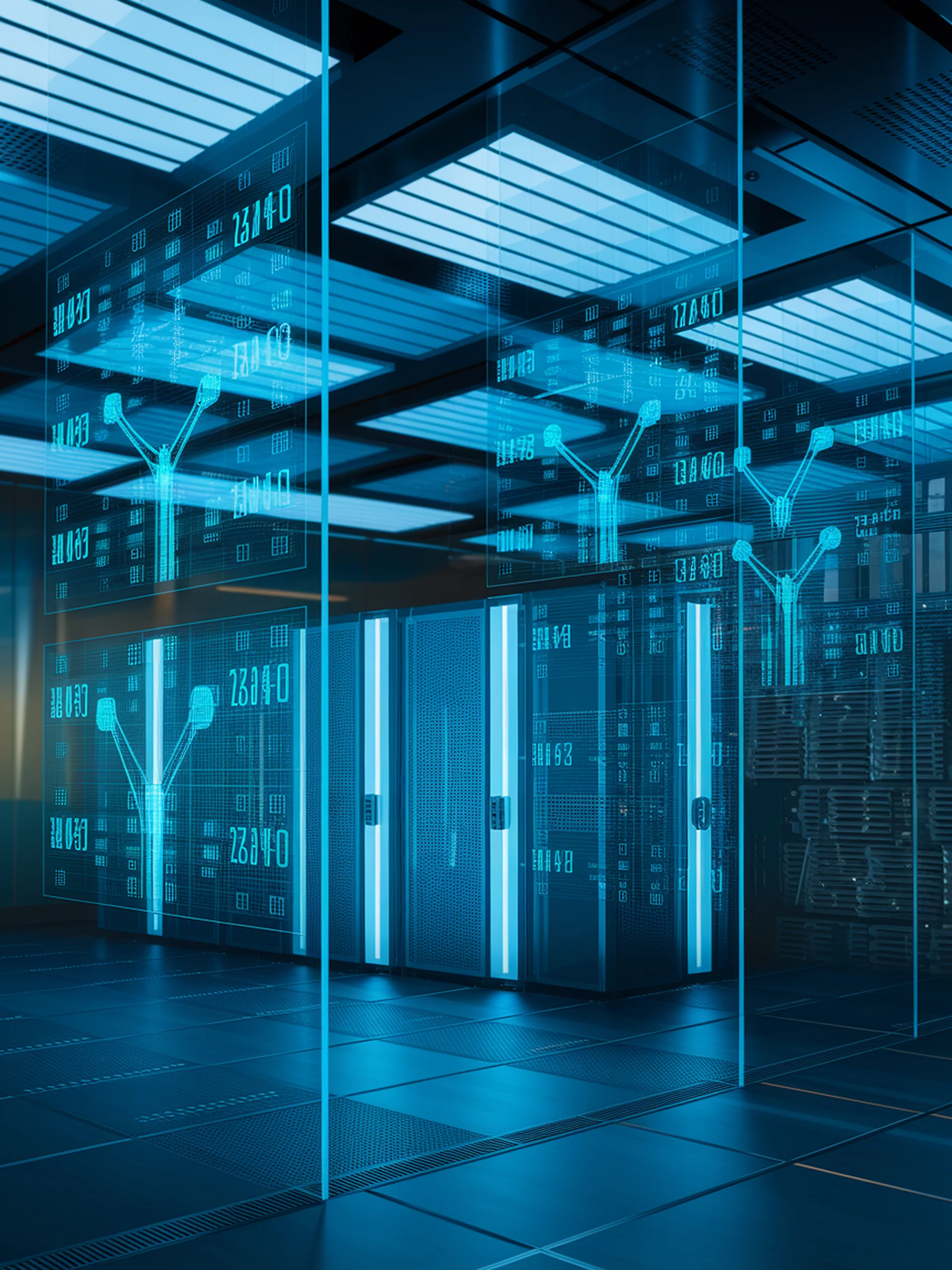
Securing RAG Systems with MES-RAG
Enhanced entity retrieval with built-in security
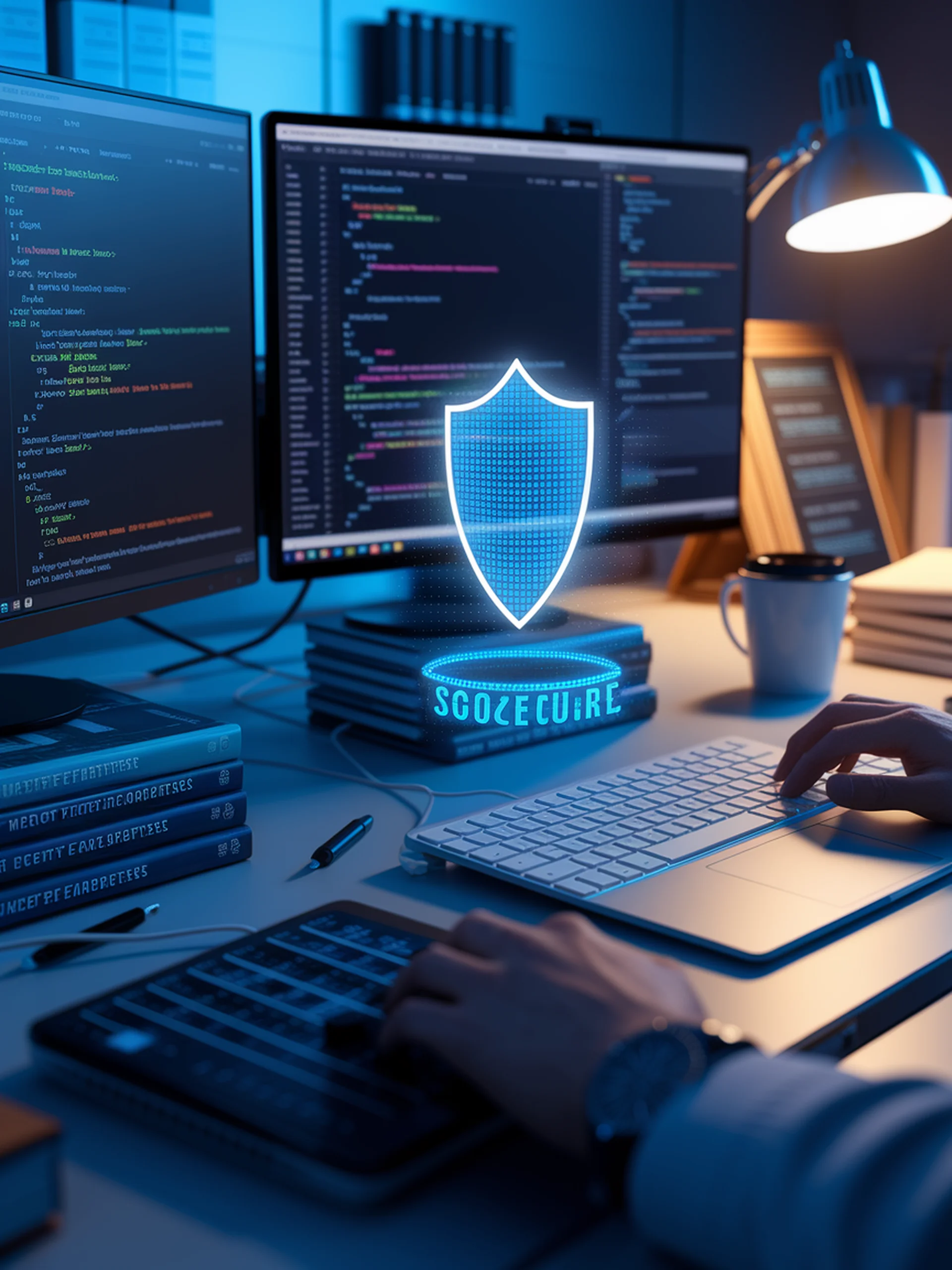
Securing AI Code Generation
Leveraging Stack Overflow to address security vulnerabilities in LLM-generated code
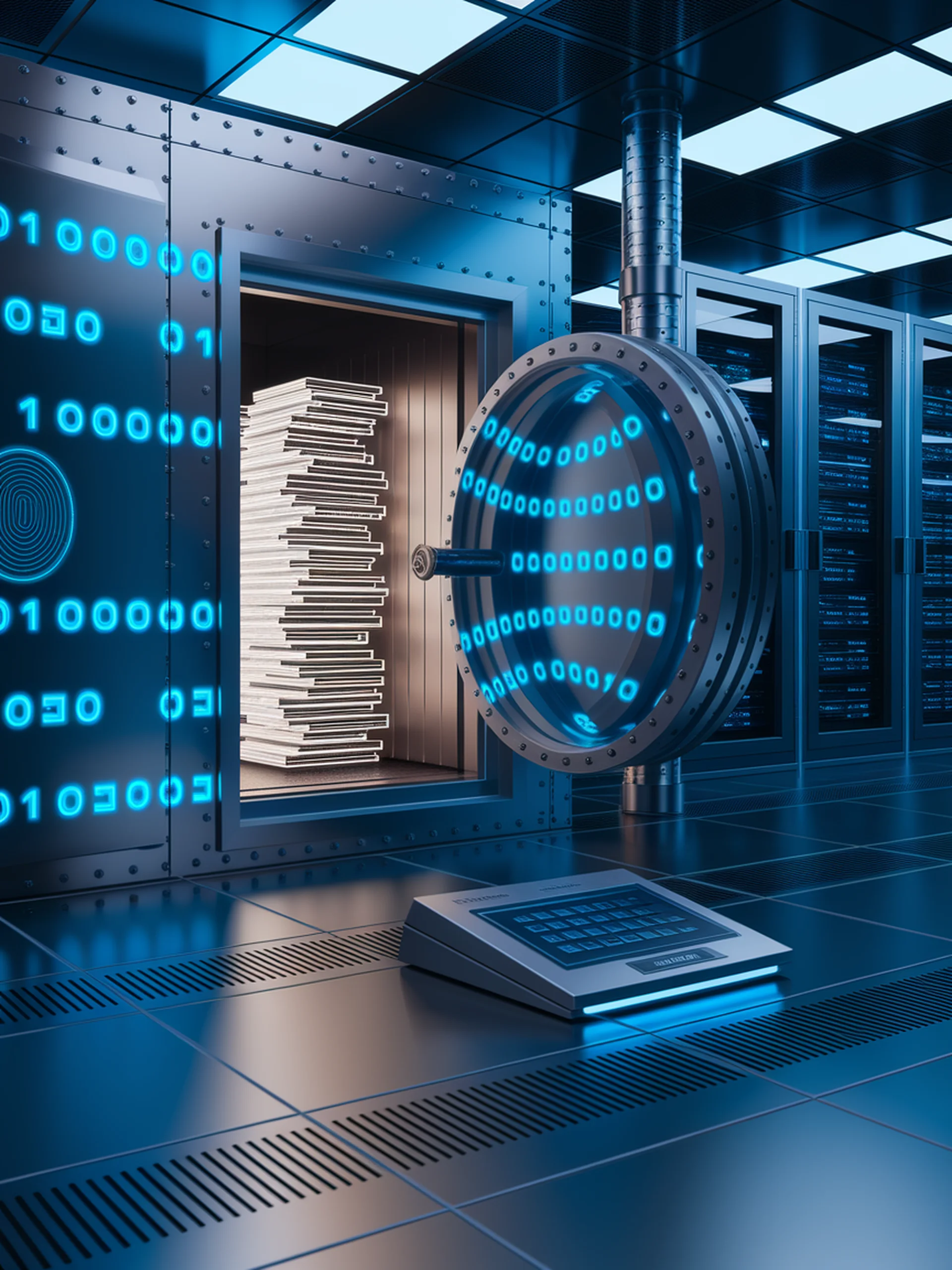
Securing RAG Systems
Advanced encryption for protecting proprietary knowledge bases
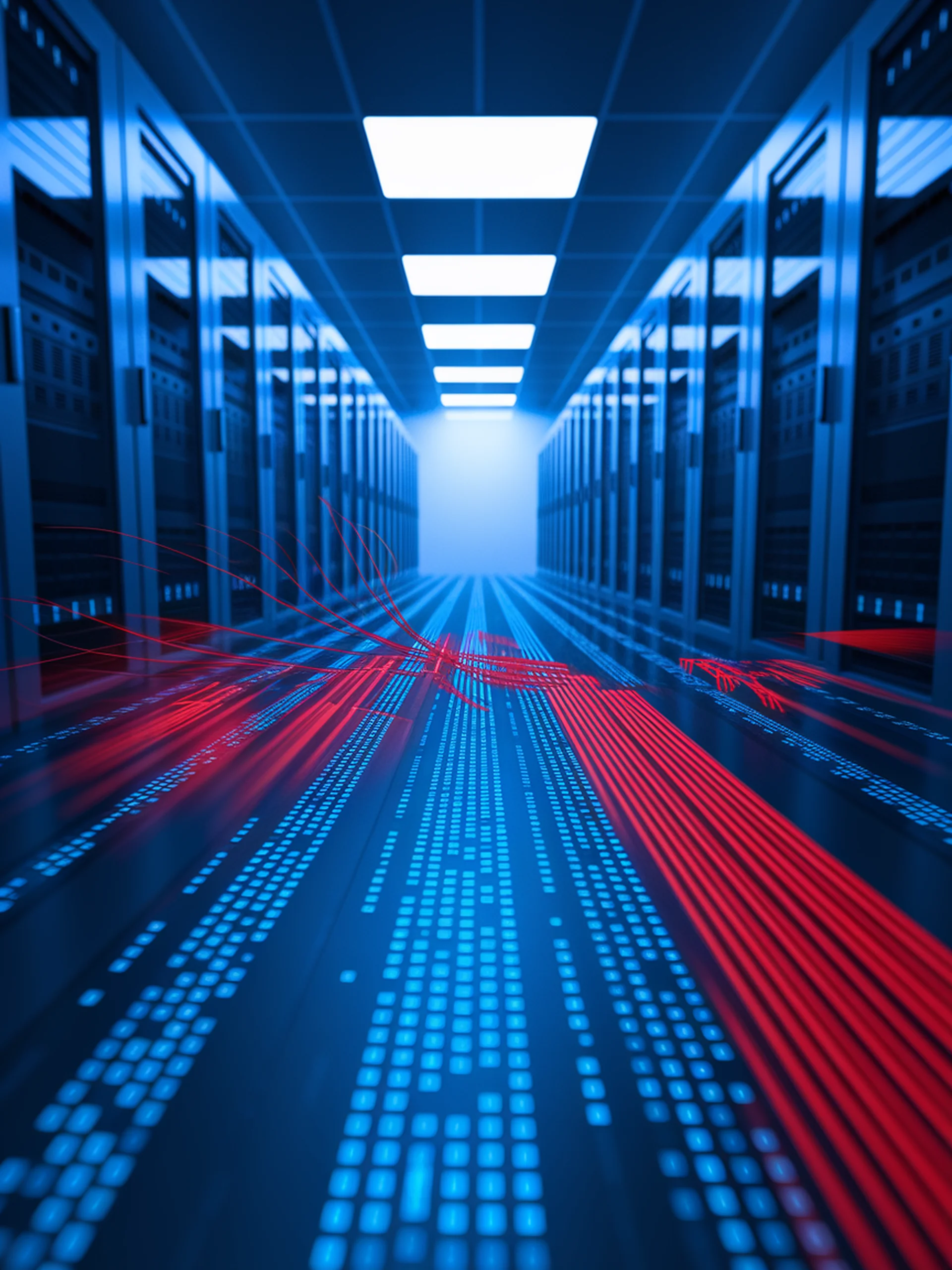
Poisoning the Well: RAG System Vulnerabilities
A new efficient attack method threatens retrieval-based AI systems
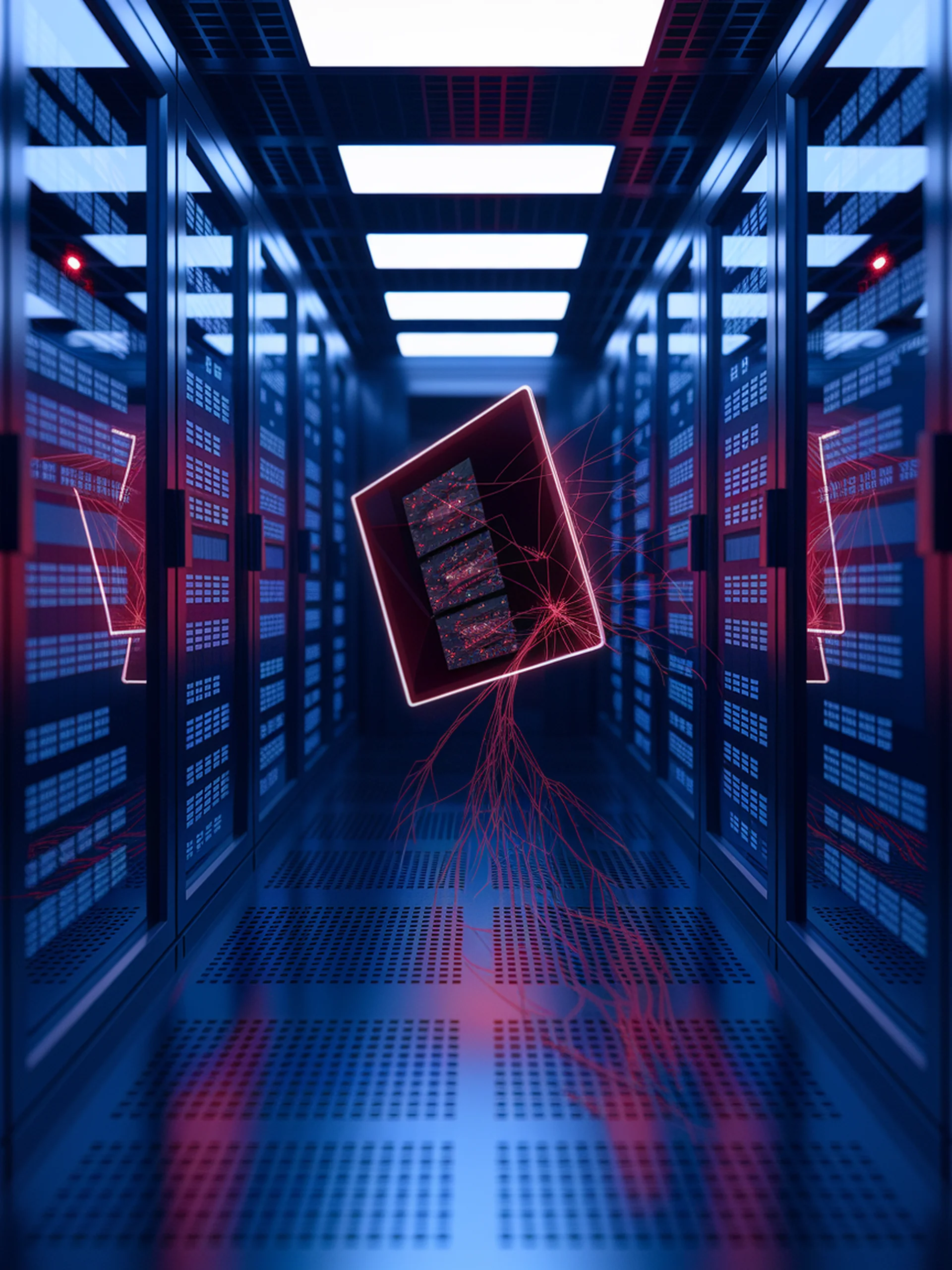
Visual Poisoning Attacks on RAG Systems
How a single malicious image can compromise document retrieval systems
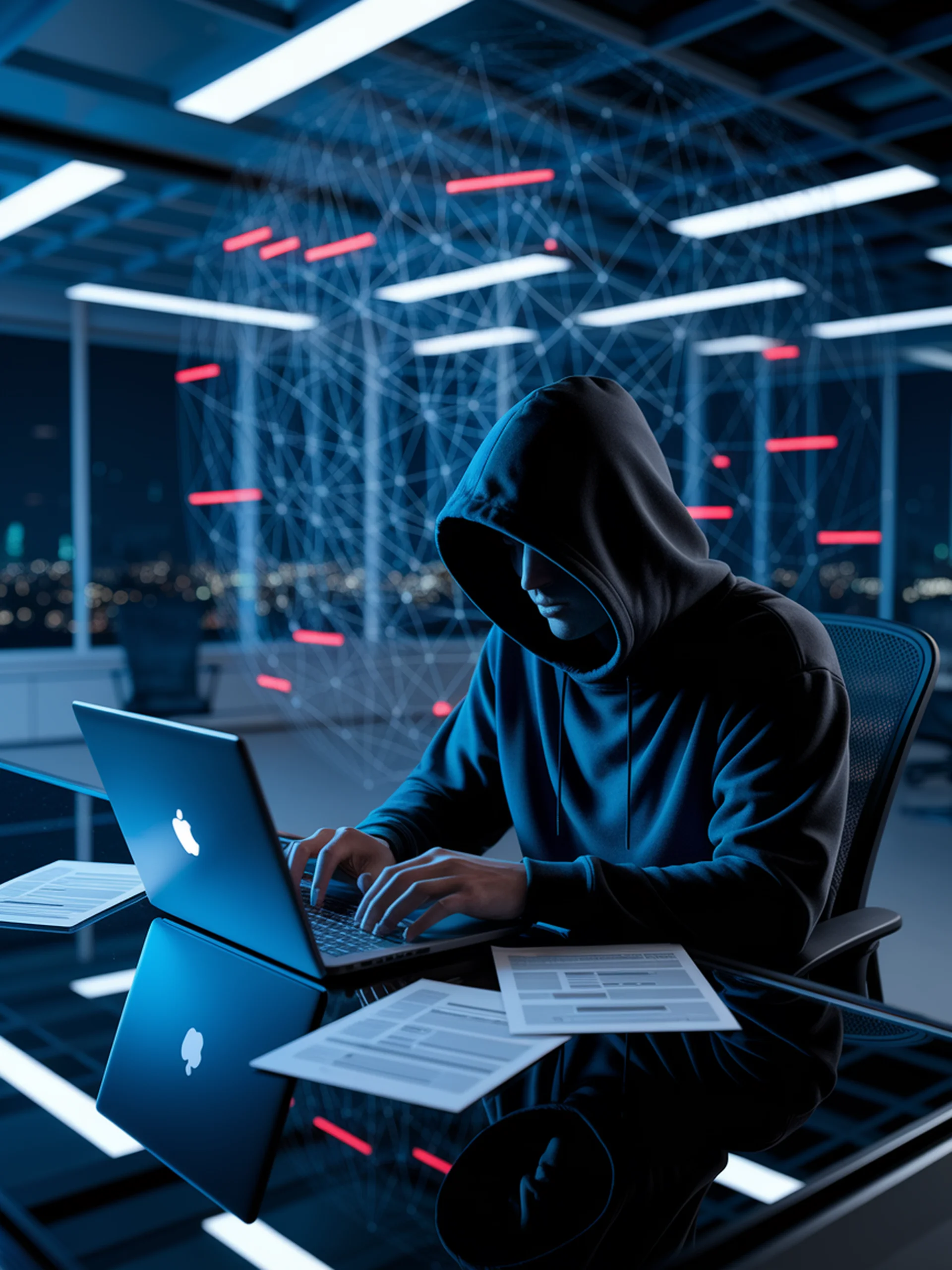
The Dark Side of RAG
How Retrieval-Augmented Generation Systems Can Be Compromised
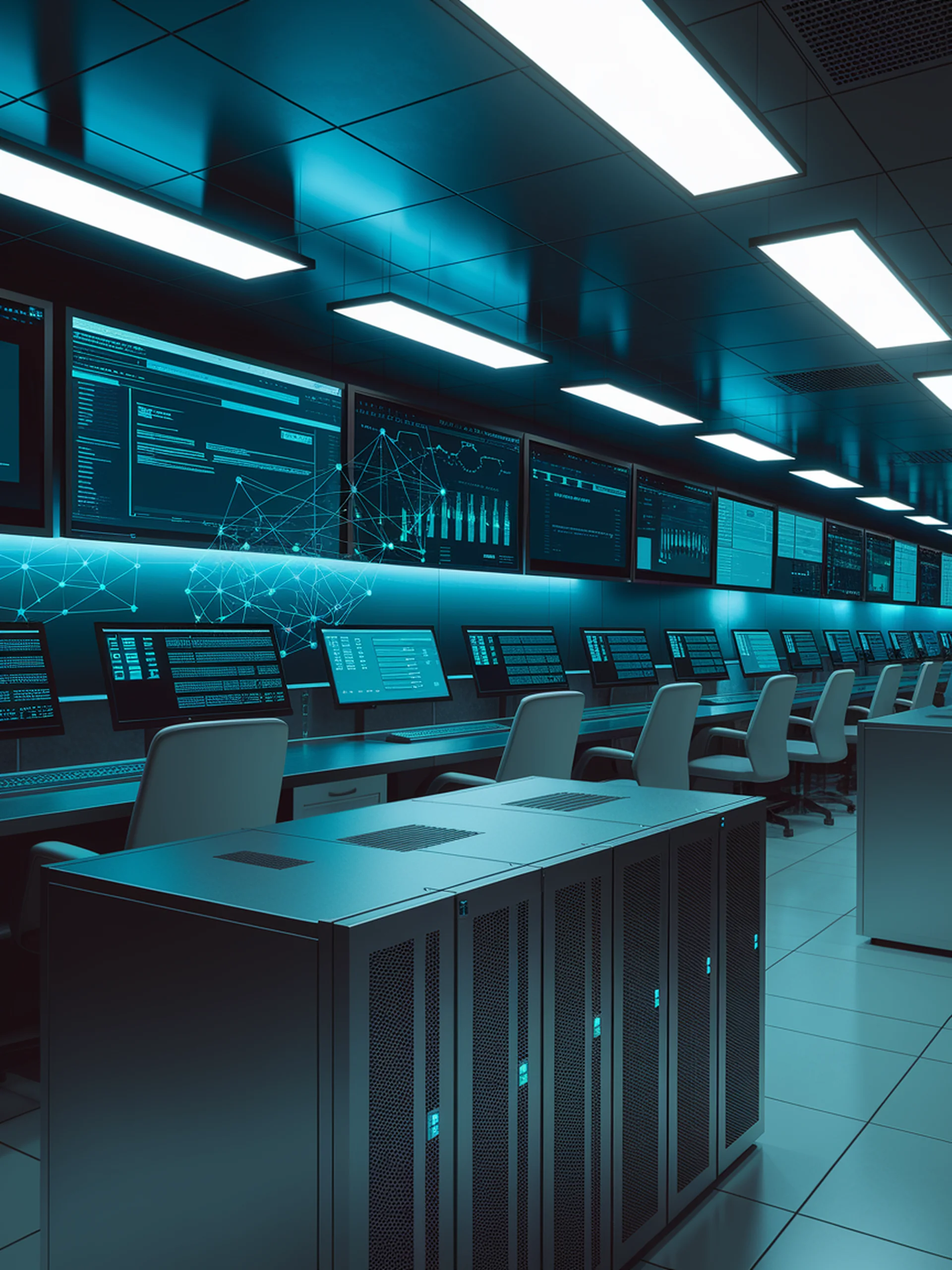
Unifying RAG for Diverse Data Sources
A streamlined approach to retrieval-augmented generation
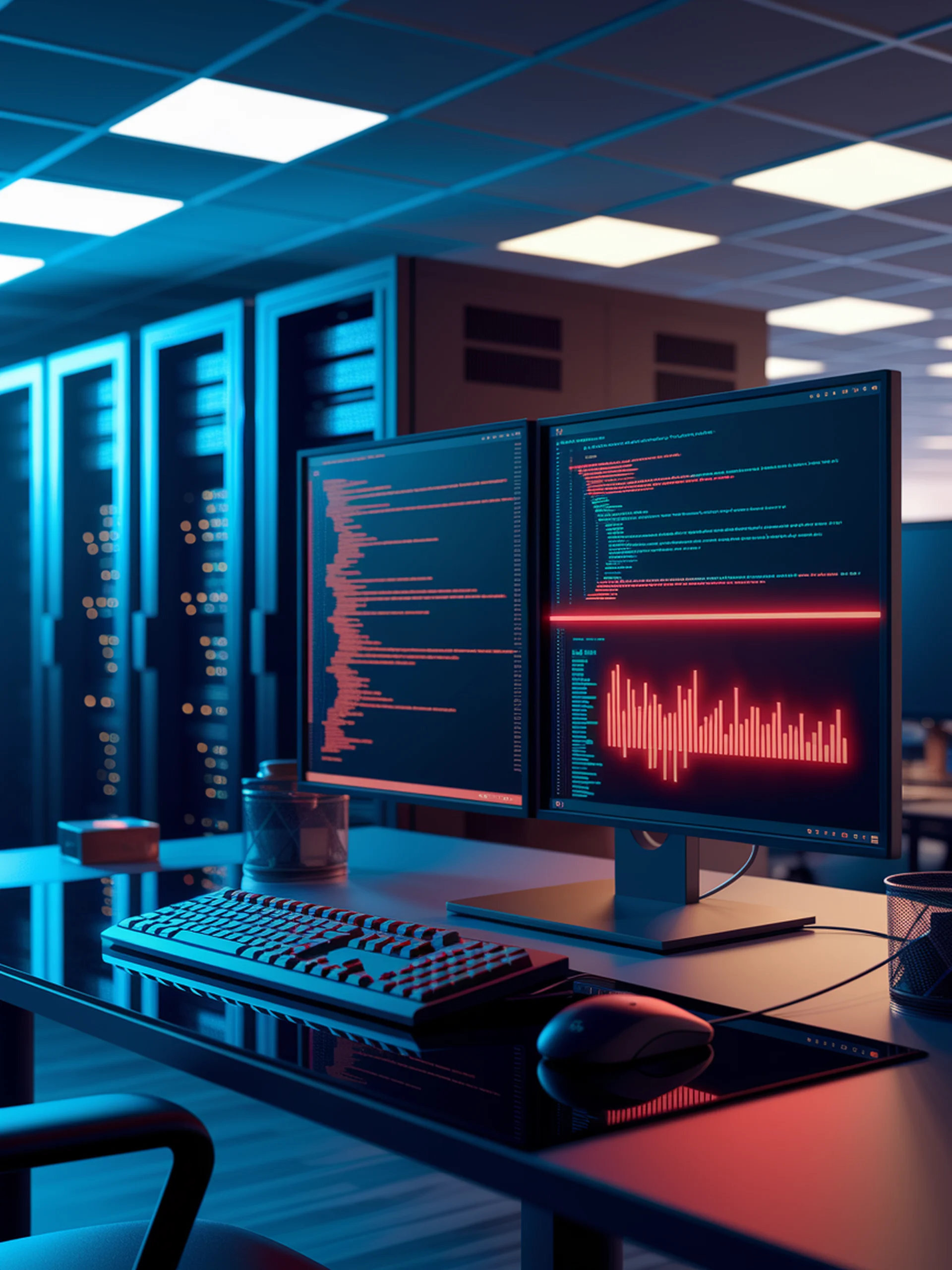
Security Vulnerabilities in RAG Systems
New attack vector threatens retrieval-augmented LLMs
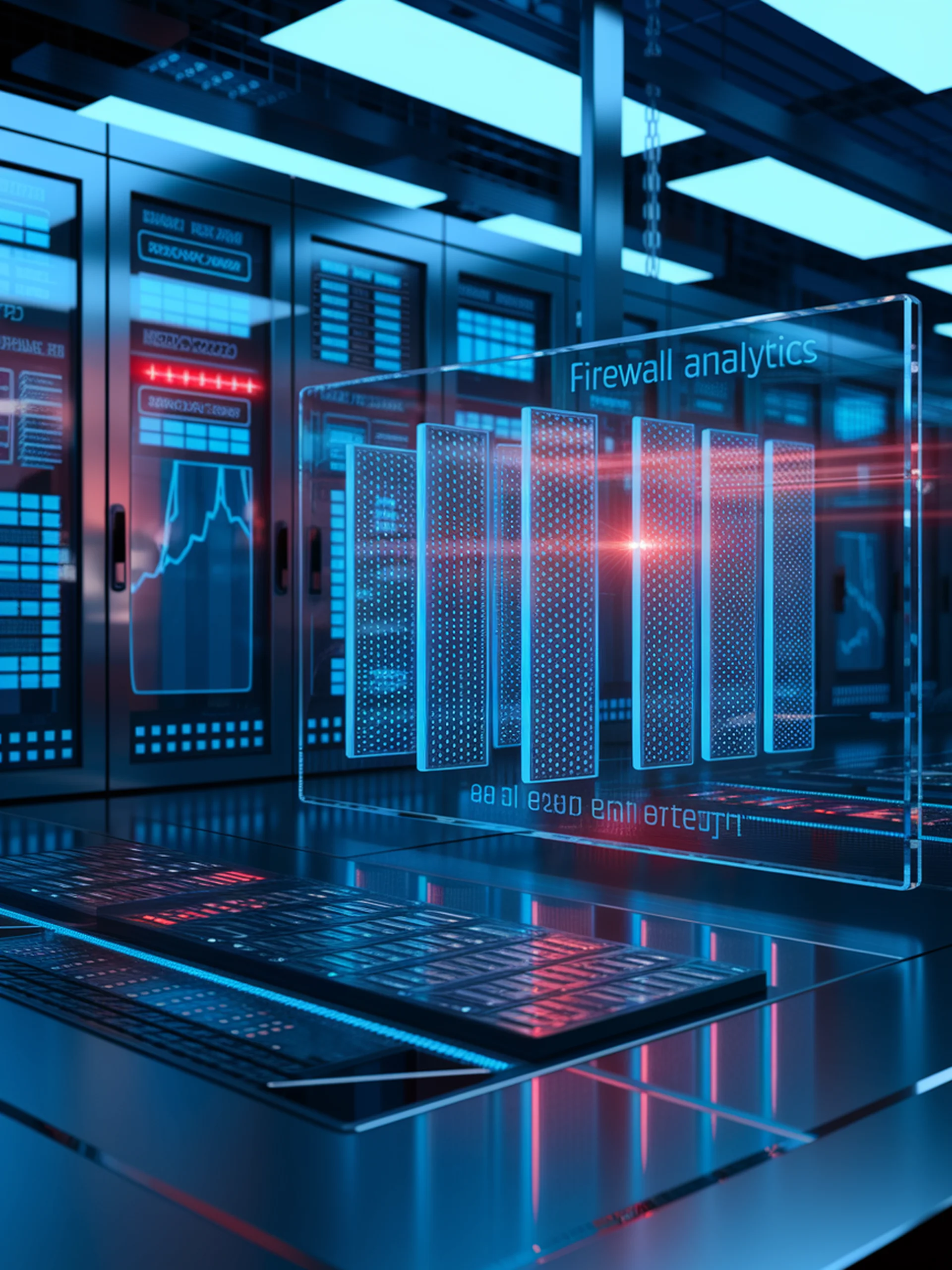