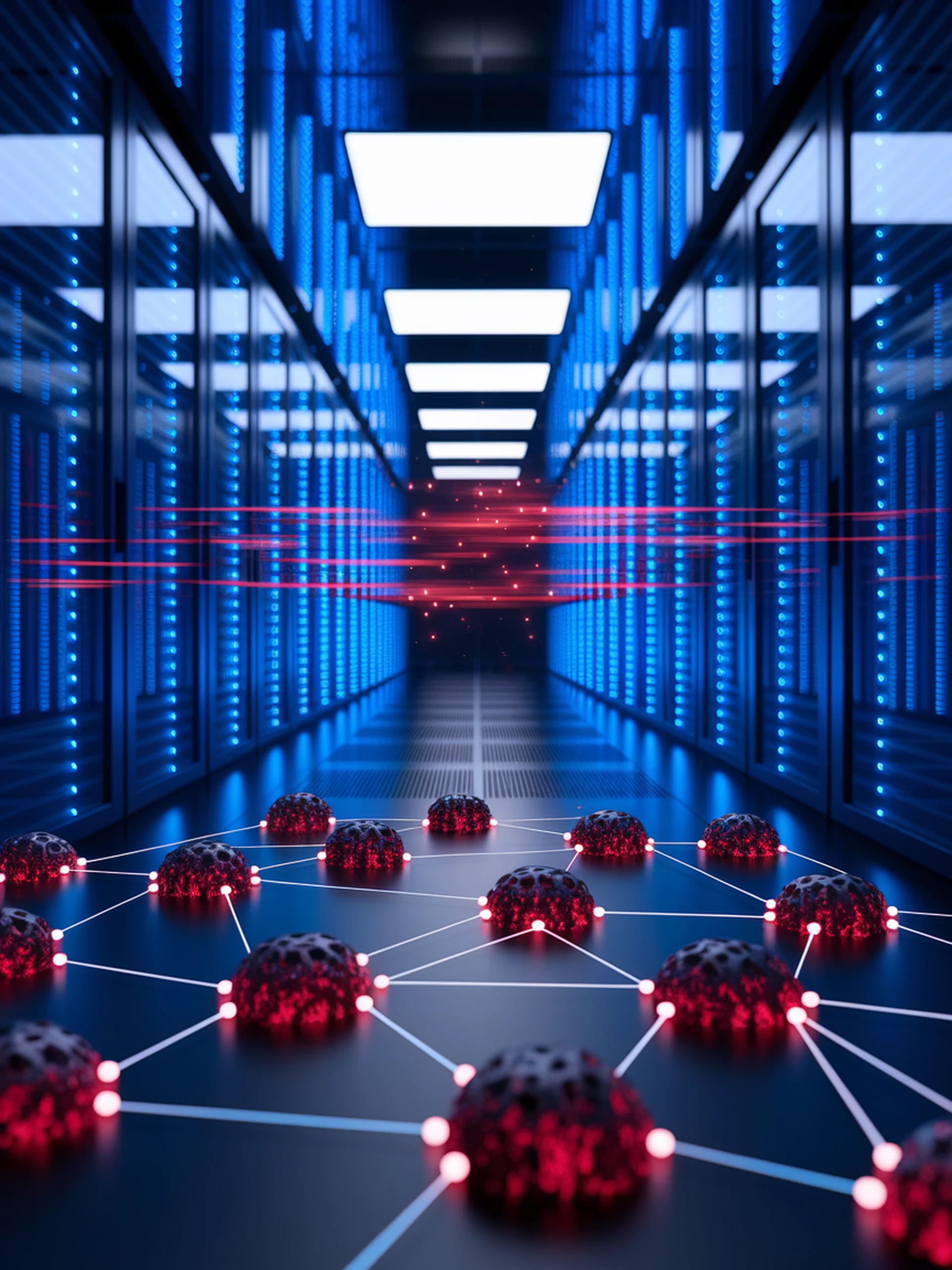
Poisoning Attacks Against Multimodal RAG
How attackers can manipulate MLLMs through knowledge poisoning
This research exposes critical security vulnerabilities in multimodal large language models that use external knowledge retrieval (RAG systems).
Key findings:
- Researchers developed two novel attack methods: Local Poisoning Attacks and Global Poisoning Attacks that can manipulate model outputs
- These attacks can inject misinformation into external knowledge bases used by multimodal systems
- The vulnerabilities highlight an urgent need for robust defense mechanisms in RAG systems
- Current multimodal RAG implementations show significant susceptibility to these attacks
This research matters for security teams because it reveals how seemingly trustworthy AI systems can be compromised through their knowledge retrieval components, potentially spreading misinformation at scale.
MM-PoisonRAG: Disrupting Multimodal RAG with Local and Global Poisoning Attacks