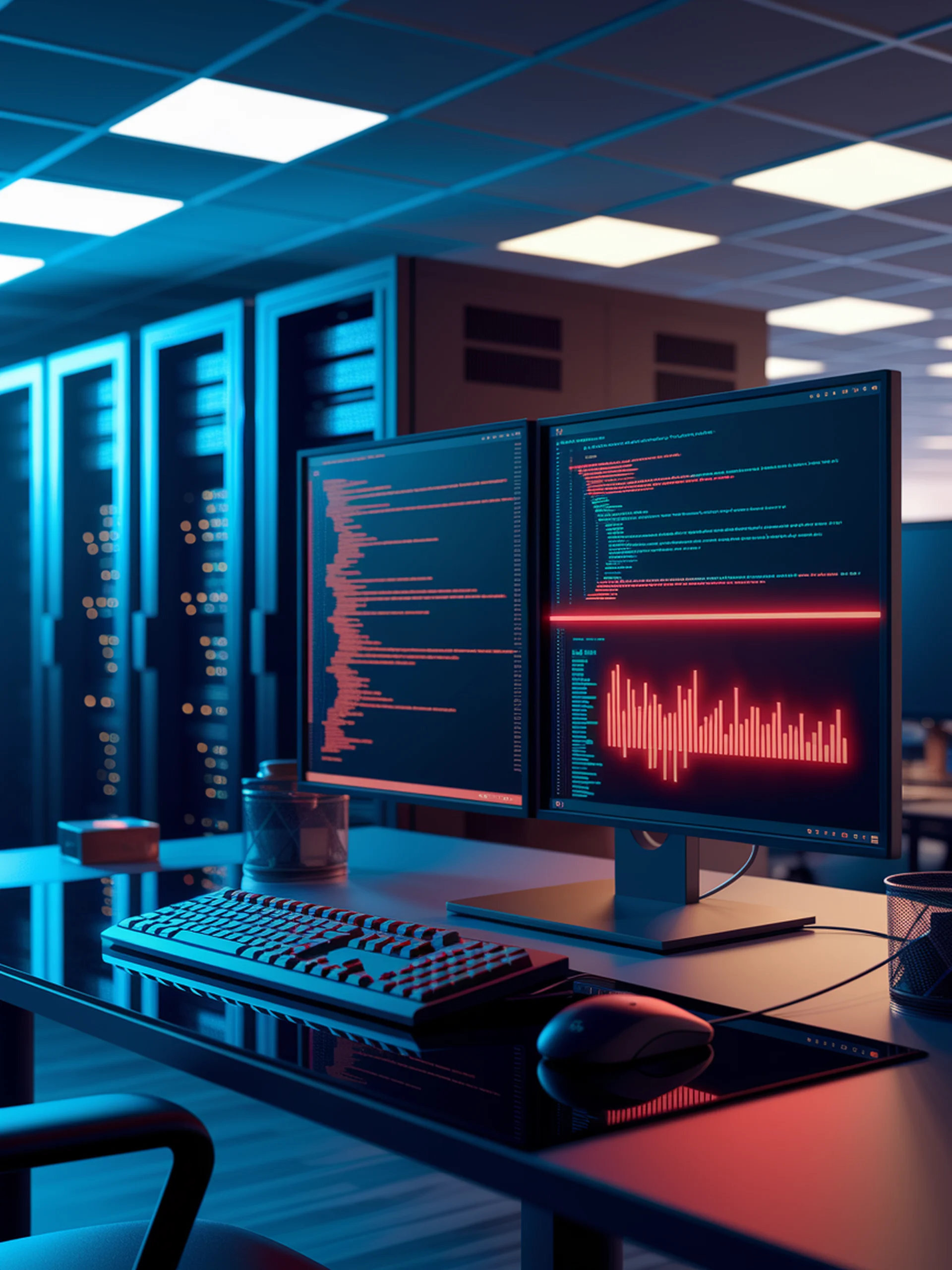
Security Vulnerabilities in RAG Systems
New attack vector threatens retrieval-augmented LLMs
This research reveals a novel PR-Attack method that compromises Retrieval-Augmented Generation (RAG) systems through coordinated poisoning of both knowledge databases and prompts.
- Demonstrates how attackers can manipulate RAG systems using bilevel optimization to coordinate database poisoning and prompt engineering
- Shows that PR-Attacks achieve higher success rates than traditional attacks while evading detection
- Reveals that multiple popular LLMs are vulnerable to these targeted attacks
- Proposes potential defense mechanisms to protect RAG systems from such exploitation
This research is crucial for security professionals as RAG adoption accelerates across industries, highlighting an urgent need for robust safeguards in knowledge-enhanced AI systems.