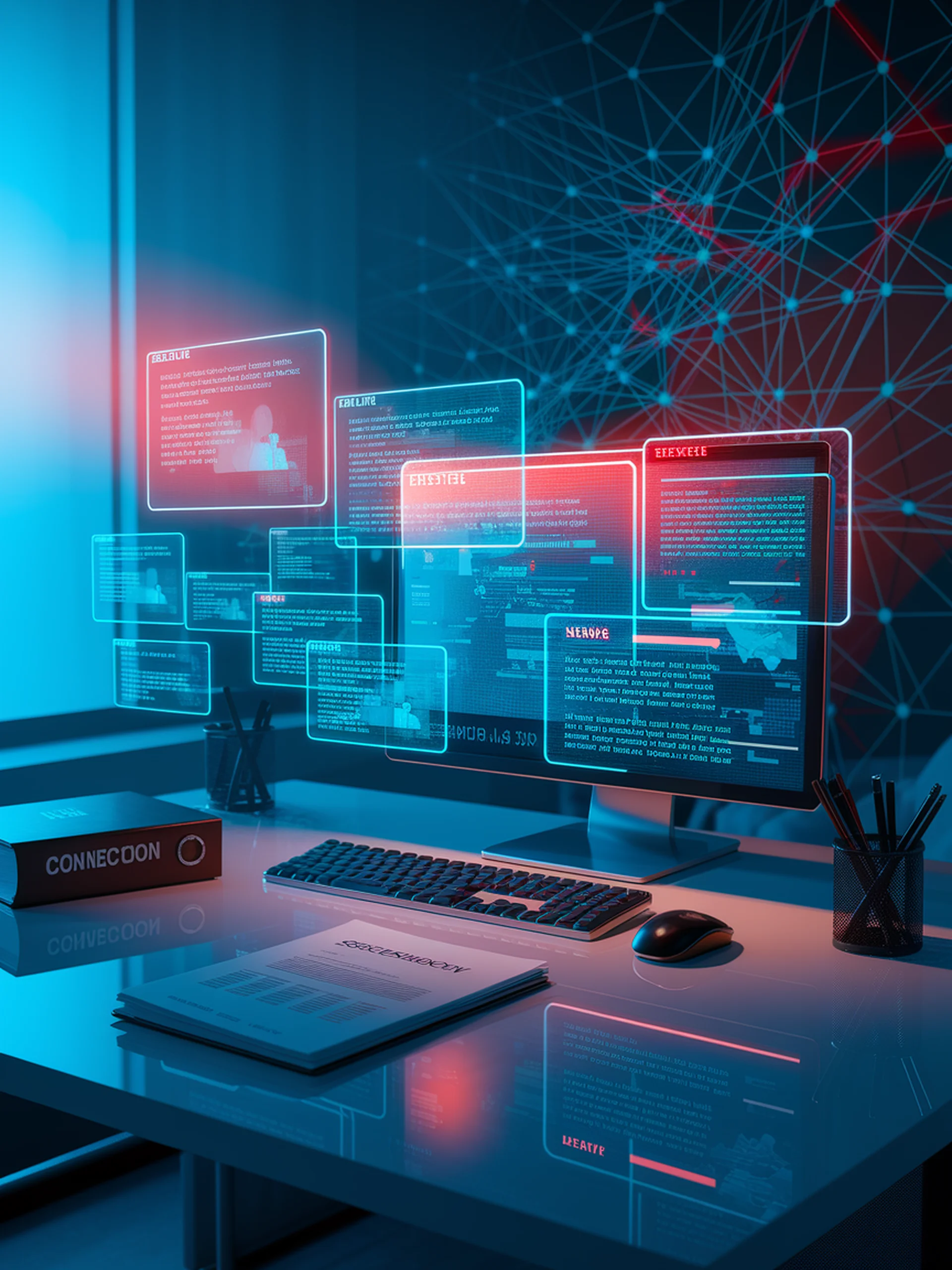
When RAG Goes Wrong: The Danger of Misleading Retrievals
Evaluating RAG's vulnerability to misinformation with RAGuard
This research introduces RAGuard, a framework to evaluate how Large Language Models handle misleading information when using Retrieval-Augmented Generation (RAG).
- RAG systems can perform worse than zero-shot when faced with misleading retrievals
- LLMs often adopt misinformation from retrieved content rather than maintaining accurate reasoning
- Political topics are particularly vulnerable due to polarized framing and selective evidence
- The study reveals the need for robust verification mechanisms in RAG systems deployed in real-world scenarios
Security Implications: As RAG becomes more prevalent in production systems, its vulnerability to misinformation presents significant security risks, especially in domains where factual accuracy is critical.