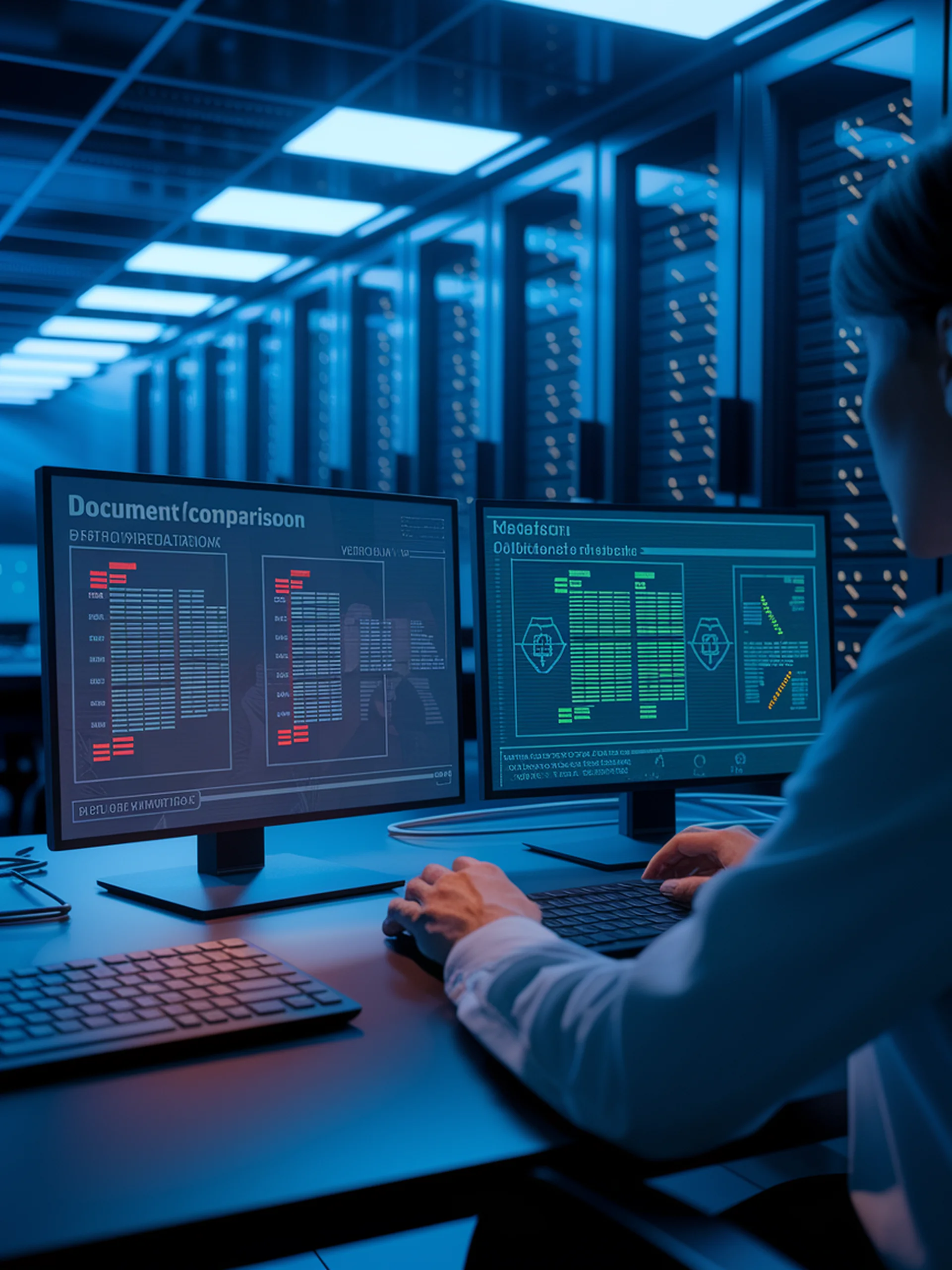
Combating Hallucinations in LLMs
A Retrieval-Augmented Approach to Detect Factual Errors
REFIND introduces a novel framework that leverages retrieved documents to identify hallucinated content in large language model outputs, enhancing reliability for knowledge-intensive tasks.
- Introduces Context Sensitivity Ratio (CSR) to measure how LLM outputs change based on retrieved context
- Uses retrieval-augmentation to directly compare LLM outputs against verified information sources
- Provides a systematic approach to detect and flag potentially false information
- Addresses critical security concerns by reducing risks of misinformation in sensitive applications
This research is crucial for security as it helps establish trustworthiness in AI systems by identifying when models generate fabricated information rather than factual content, especially important in high-stakes environments like healthcare, finance, or legal contexts.