Interpretability for LLM Security
Research on understanding and explaining LLM internal states and mechanisms to improve security, detect vulnerabilities, and enable safer steering of model behavior
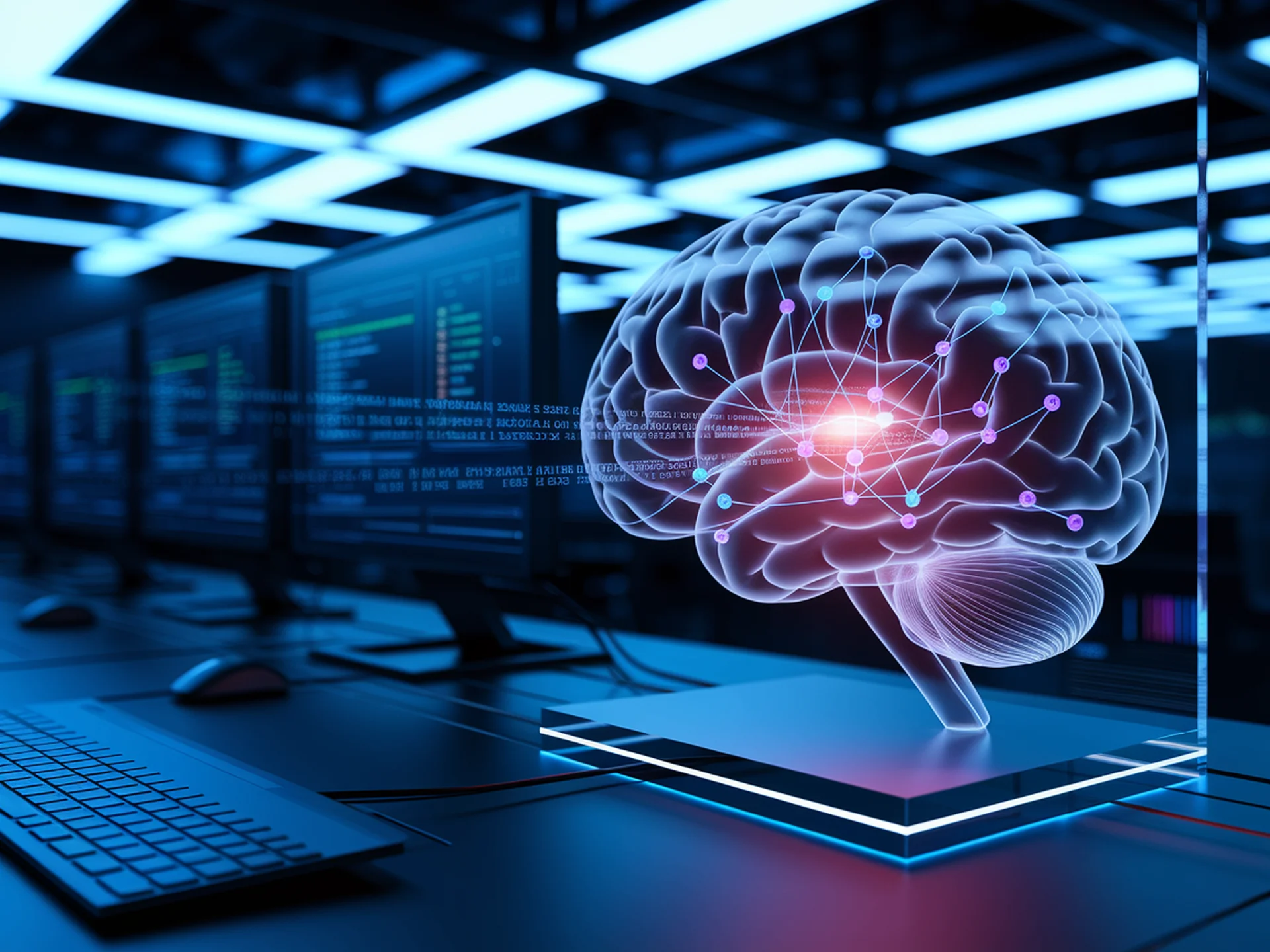
Interpretability for LLM Security
Research on Large Language Models in Interpretability for LLM Security
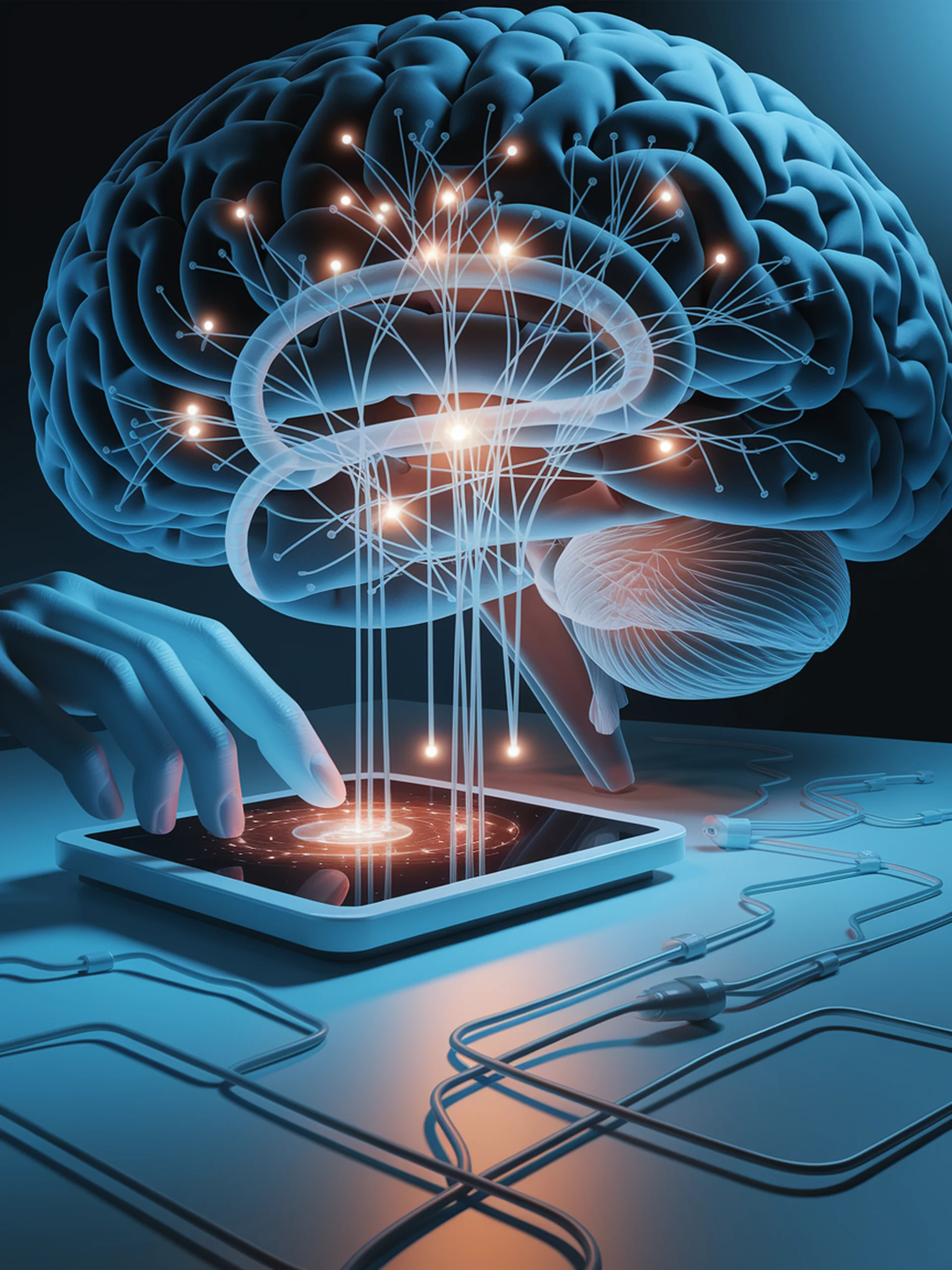
Making LLMs Interpretable and Steerable
Using Mutual Information to Explain Sparse Autoencoder Features
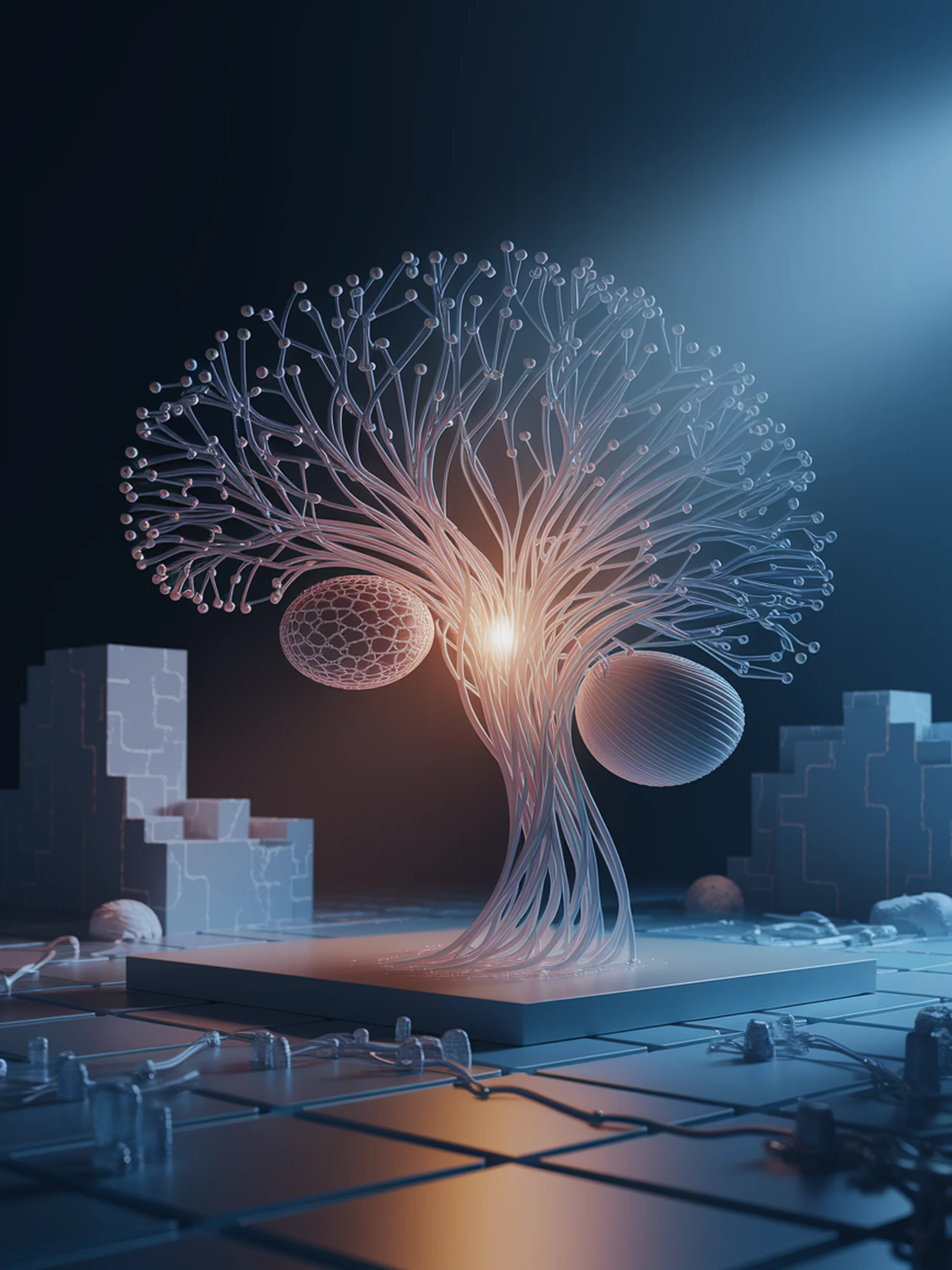
Mapping the Uncertainty in LLM Explanations
A novel framework using reasoning topology to quantify explanation reliability
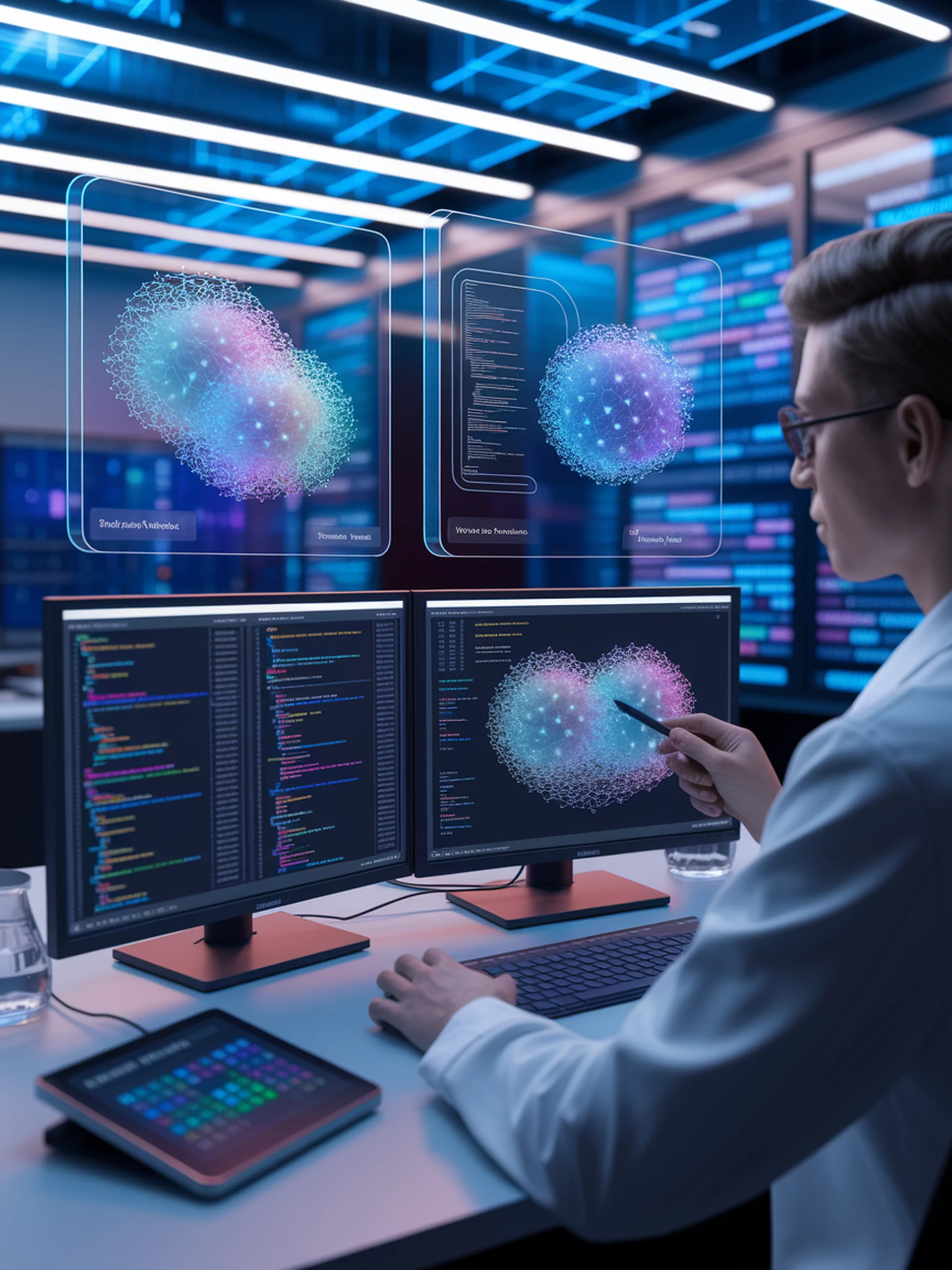
Smarter Data Interpretation via Language Models
A novel method for extracting meaningful features from datasets using LLMs
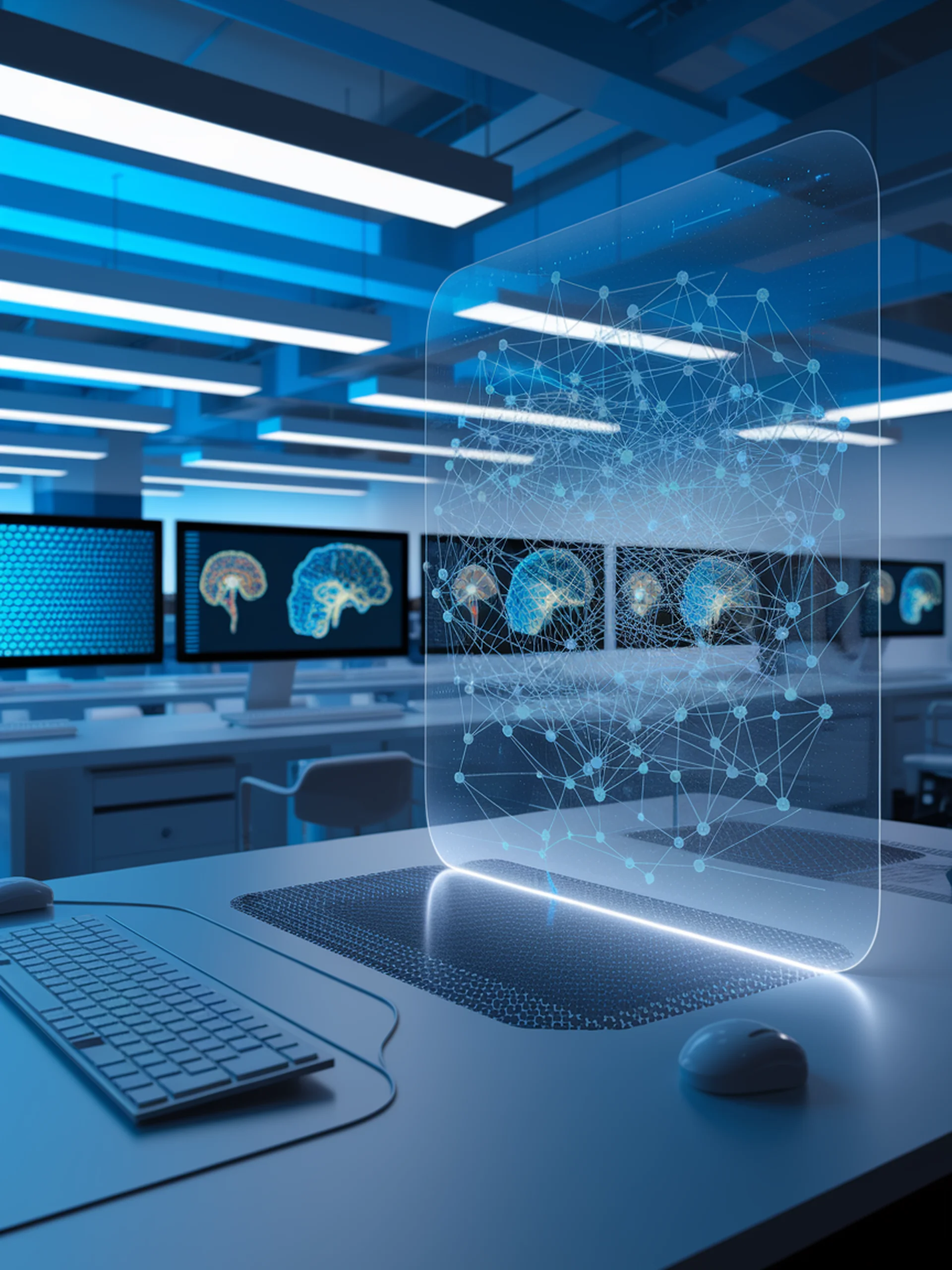
Engineering Safer AI Representations
A new approach to make LLMs more predictable and controllable
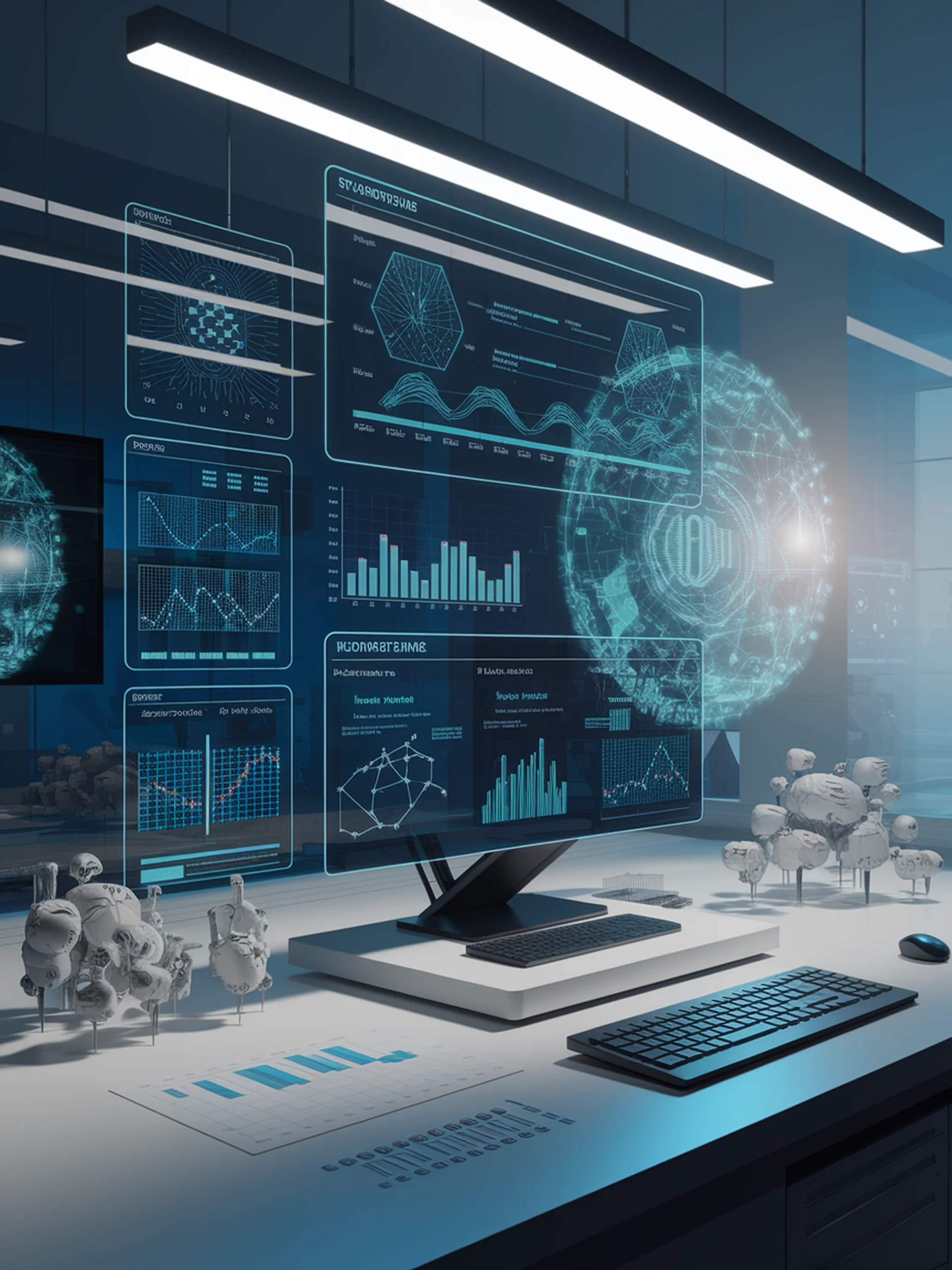
Bridging LLMs and Statistics
A Framework for Statisticians to Understand and Leverage AI Models
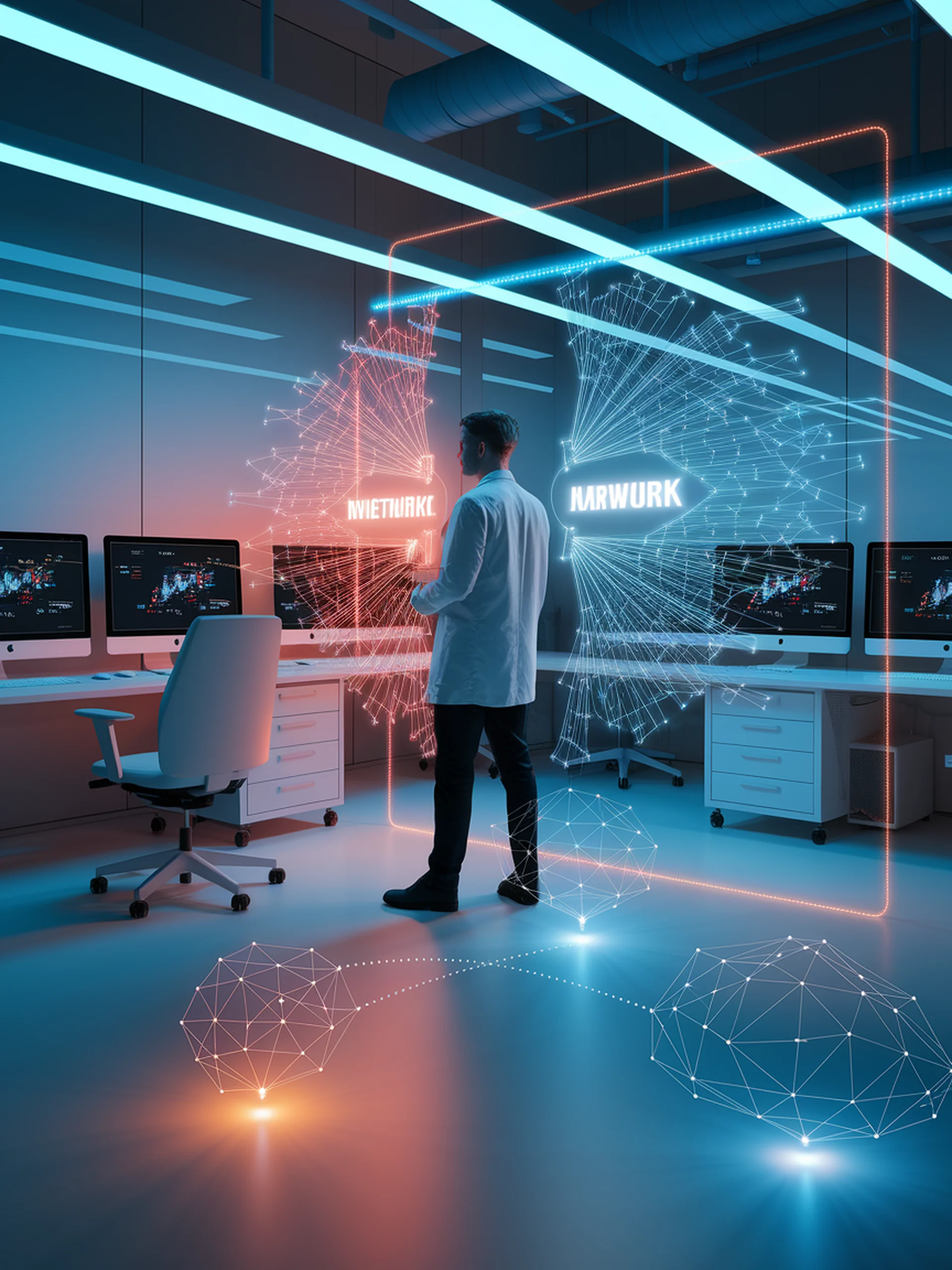
Controlling AI Text Generation
Making LLMs safer through causal reasoning in latent space
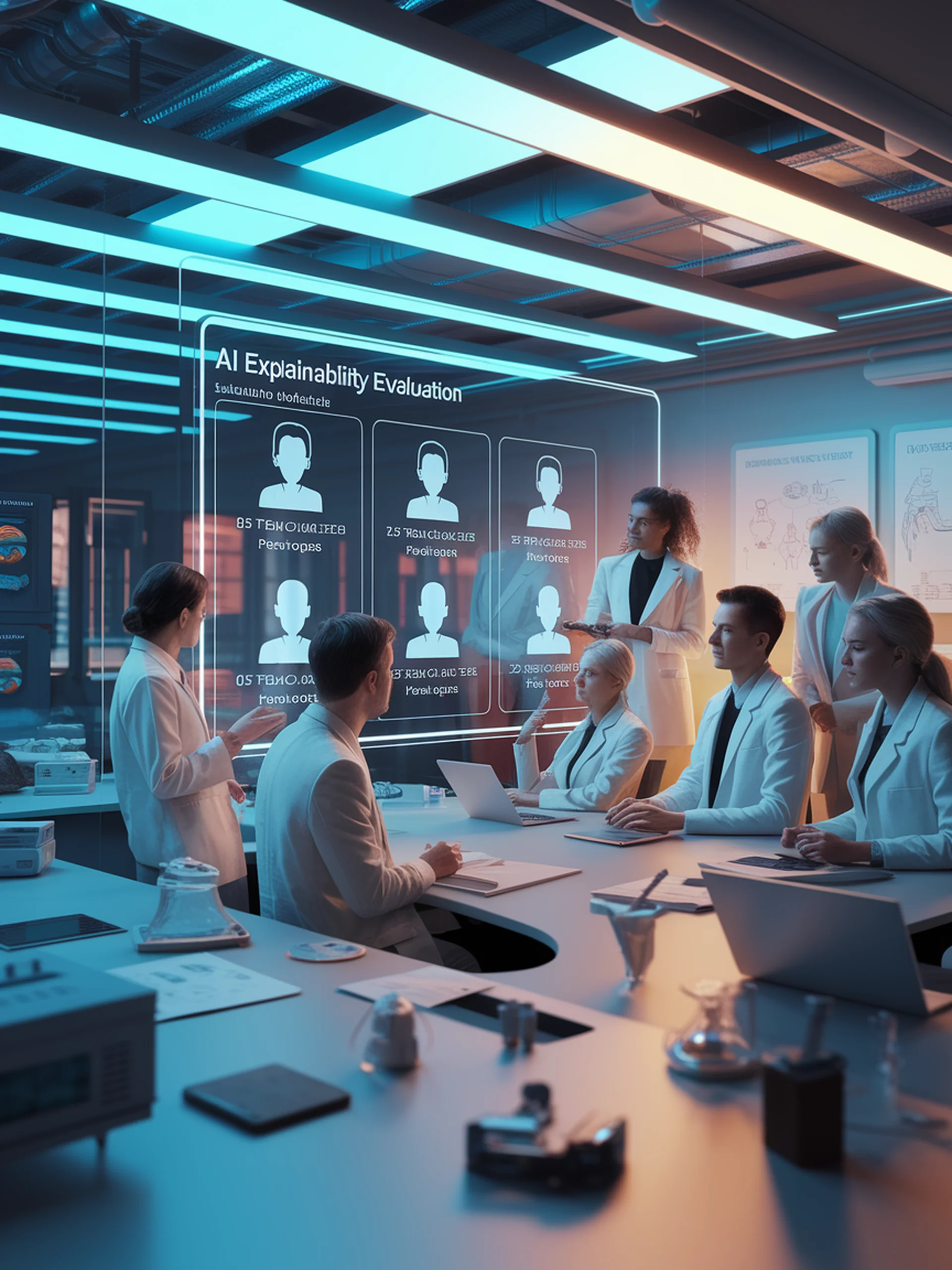
Human-Centered XAI Evaluation
Using AI-Generated Personas to Assess Explainable AI Systems
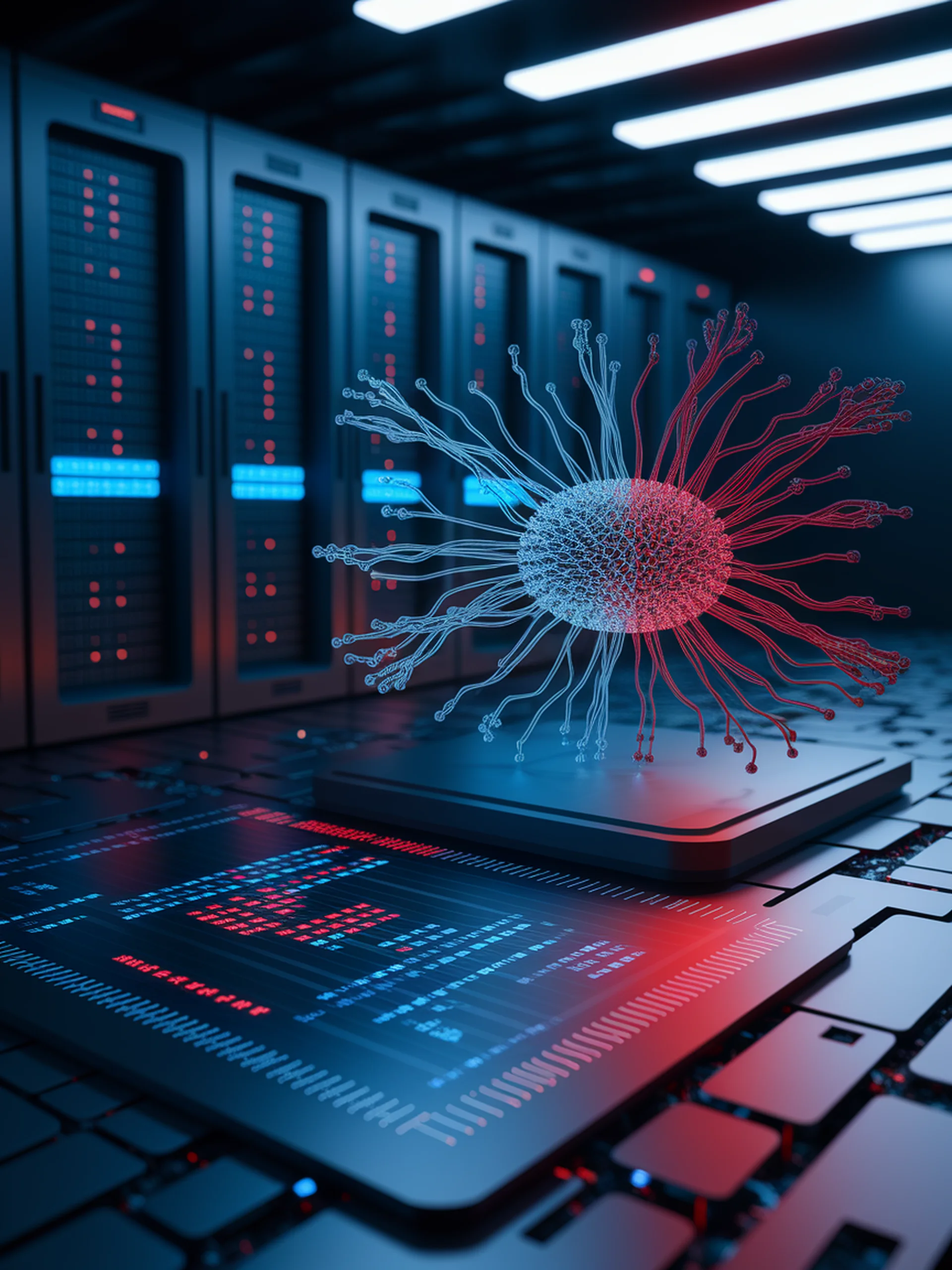
Smarter Attacks on AI Systems
How Understanding LLM Internals Creates Better Adversarial Attacks
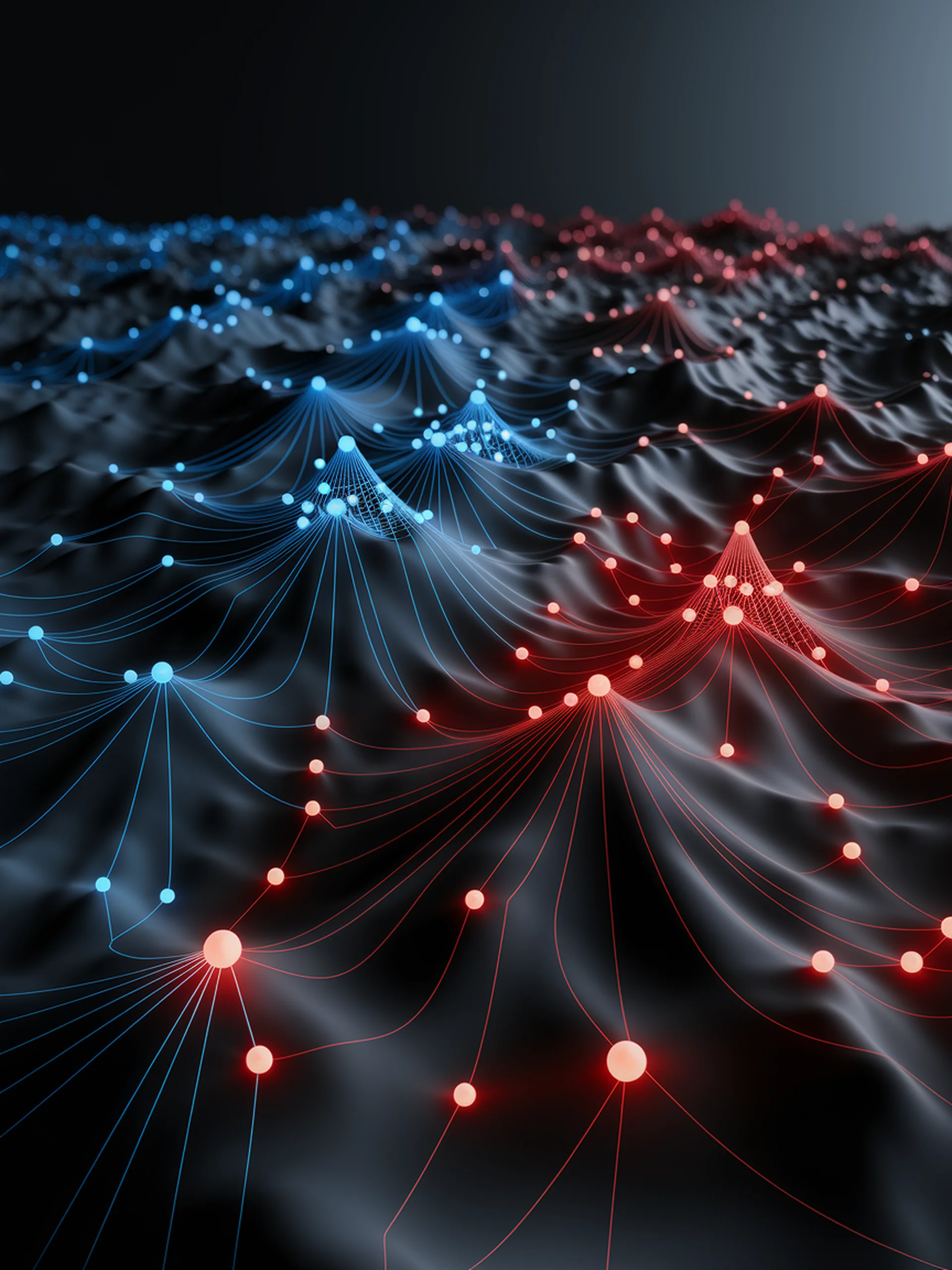
Mapping the Vulnerable Landscapes of LLMs
Revealing and manipulating adversarial states in language model security
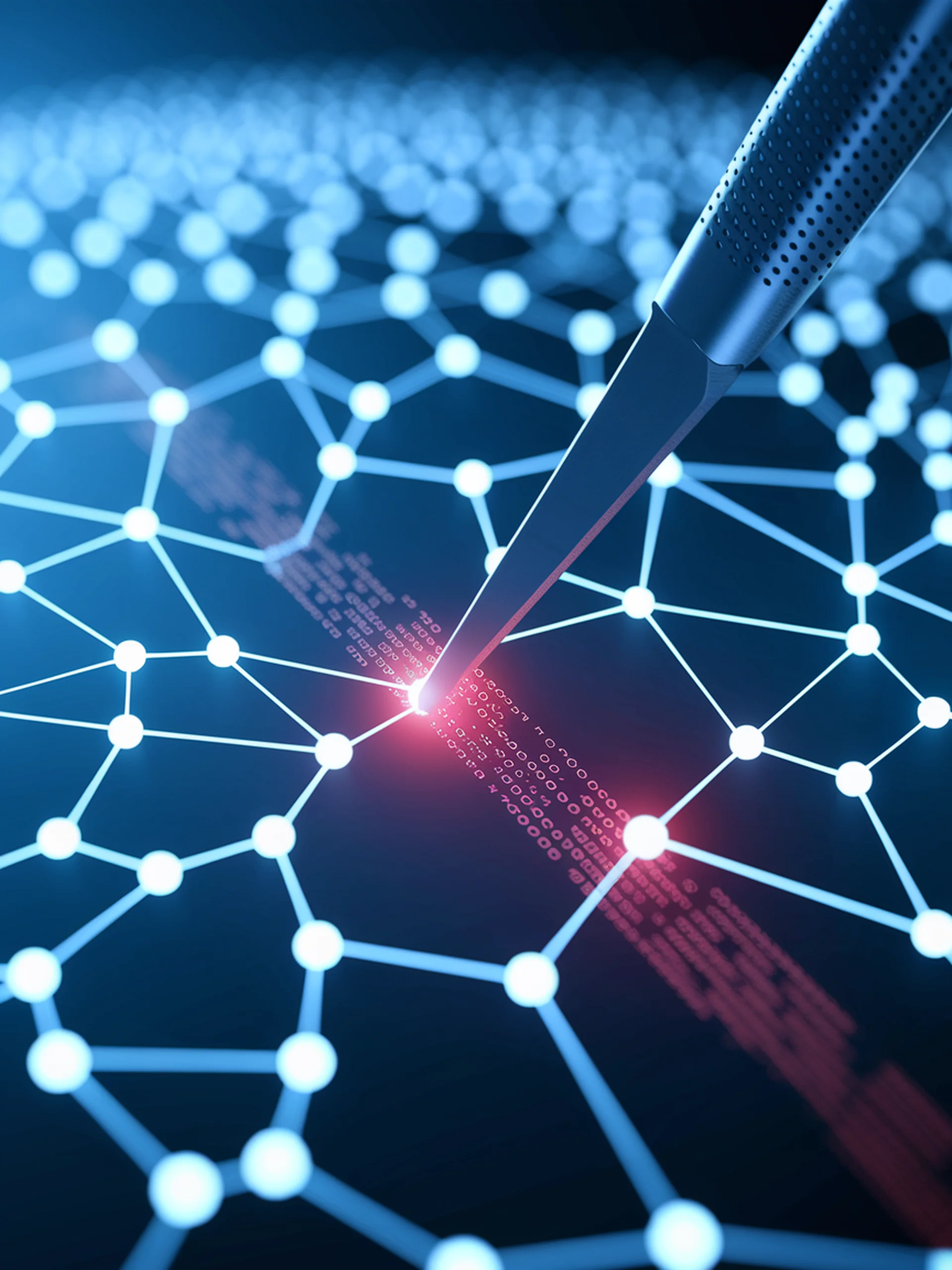
Surgical Privacy for LLMs
Removing PII without compromising performance
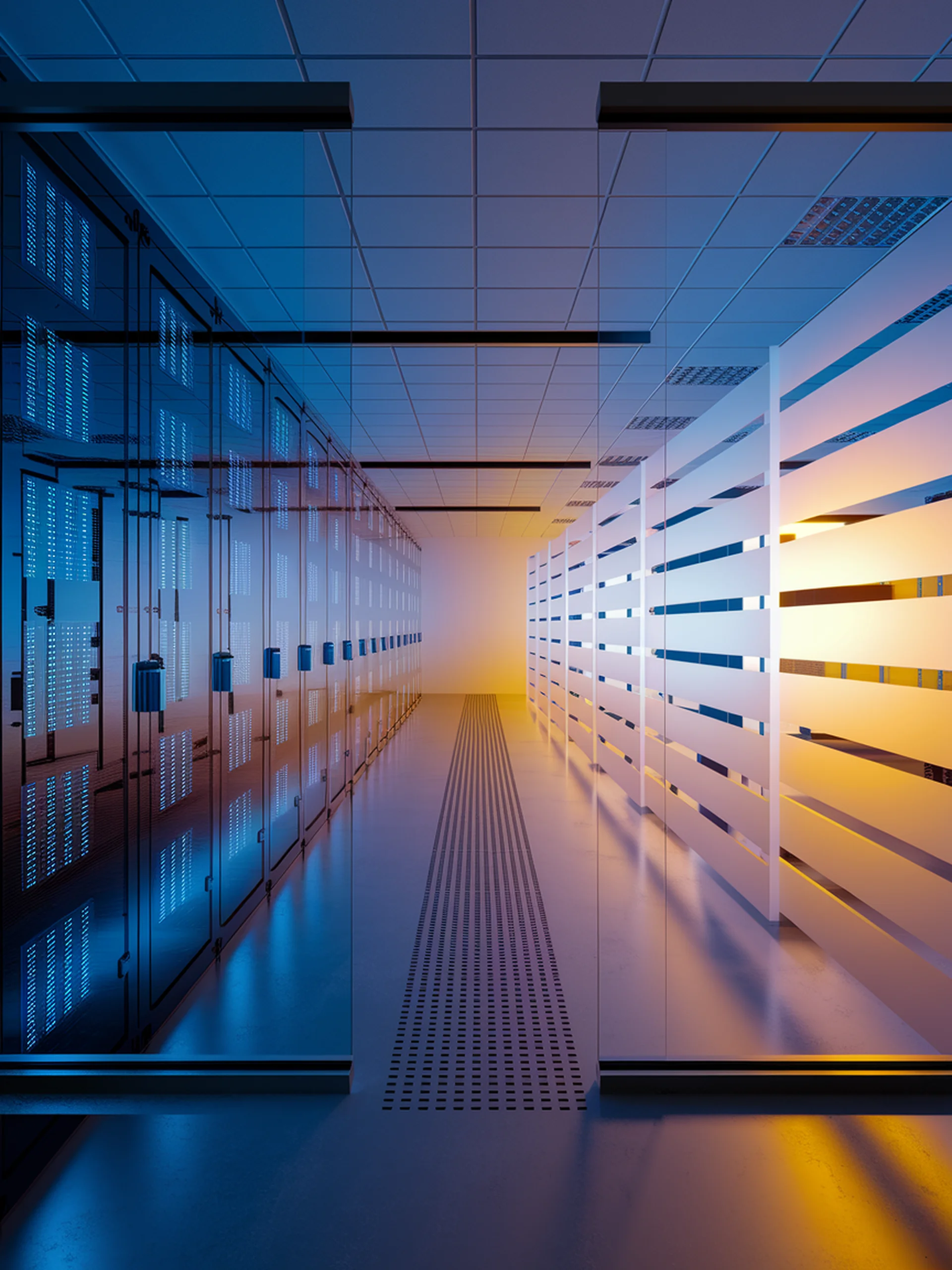
Balancing Transparency and Security in AI Reasoning
A policy framework for Chain-of-Thought disclosure in LLMs
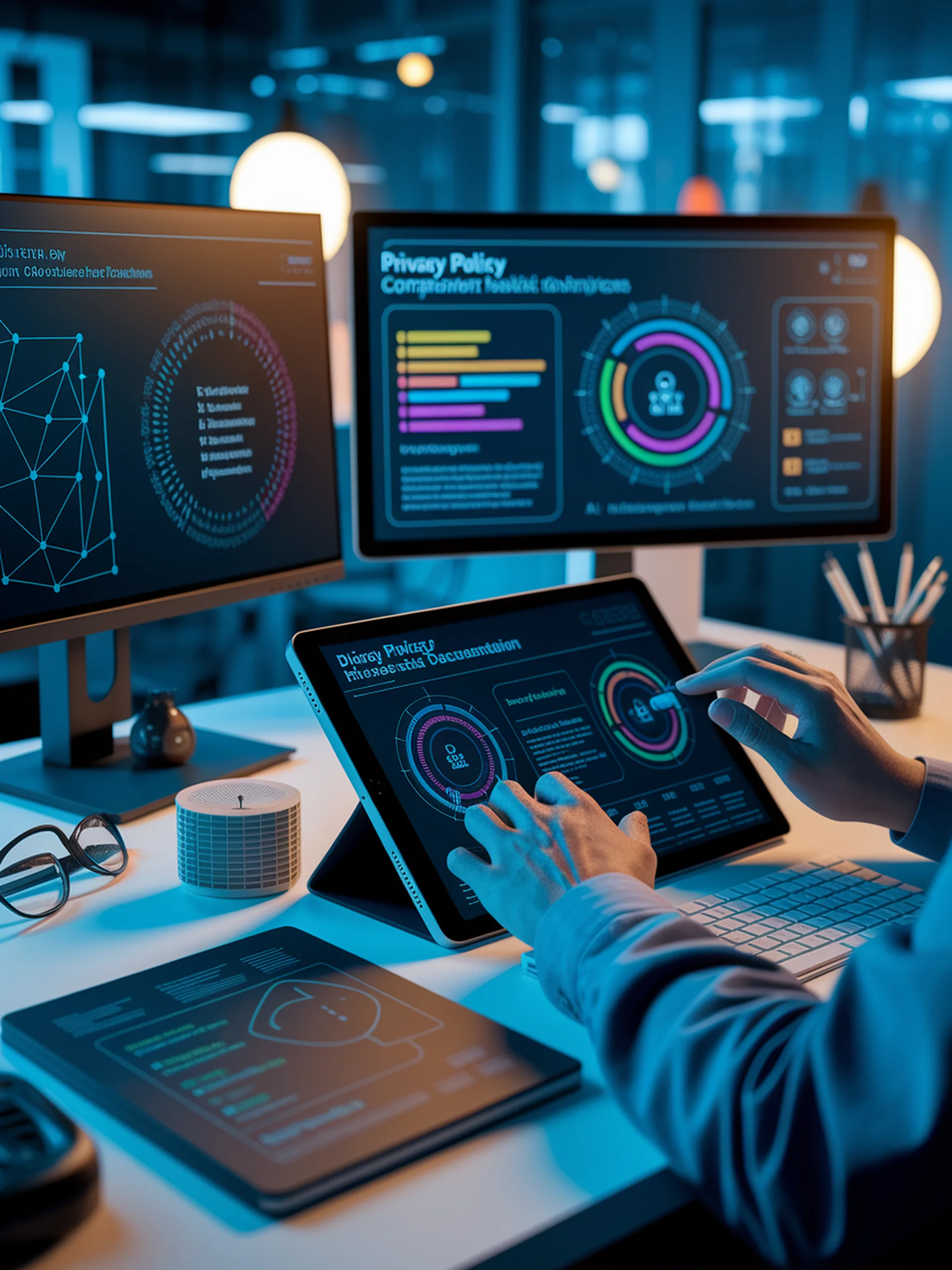