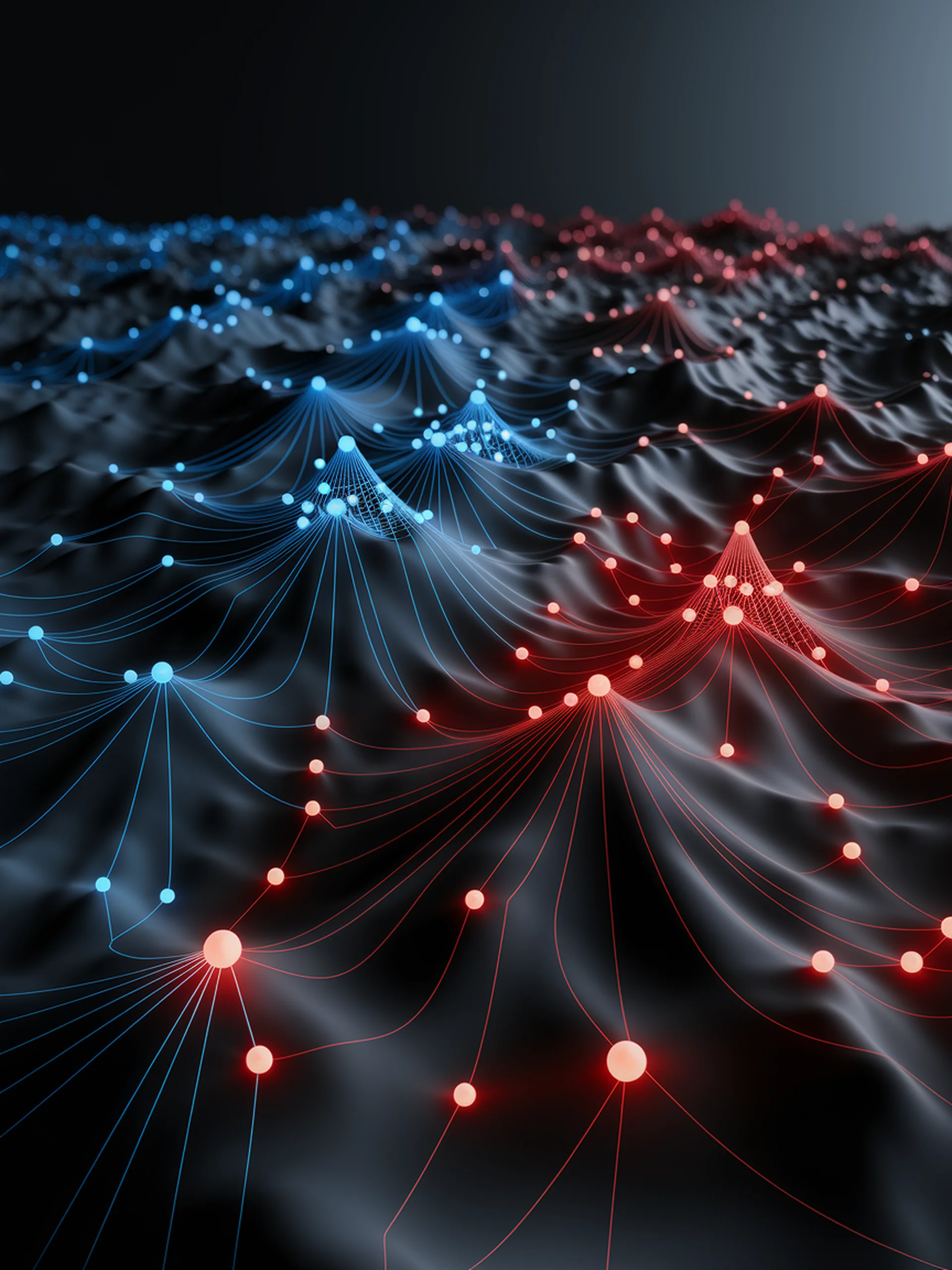
Mapping the Vulnerable Landscapes of LLMs
Revealing and manipulating adversarial states in language model security
This research identifies distinct neural activation patterns associated with jailbroken states in Large Language Models, revealing how security vulnerabilities manifest in model internals.
- Researchers identified separable subspaces in neural activations corresponding to normal vs. jailbroken states
- The team could shift model behavior by manipulating these activation patterns directly
- Analysis showed that security vulnerabilities exist as identifiable patterns in the model's latent space
- Findings pave the way for more robust defense mechanisms against prompt injection attacks
For security professionals, this work provides a deeper understanding of how jailbreaking succeeds at a neural level, potentially enabling better detection and prevention of adversarial attacks.
Probing Latent Subspaces in LLM for AI Security: Identifying and Manipulating Adversarial States