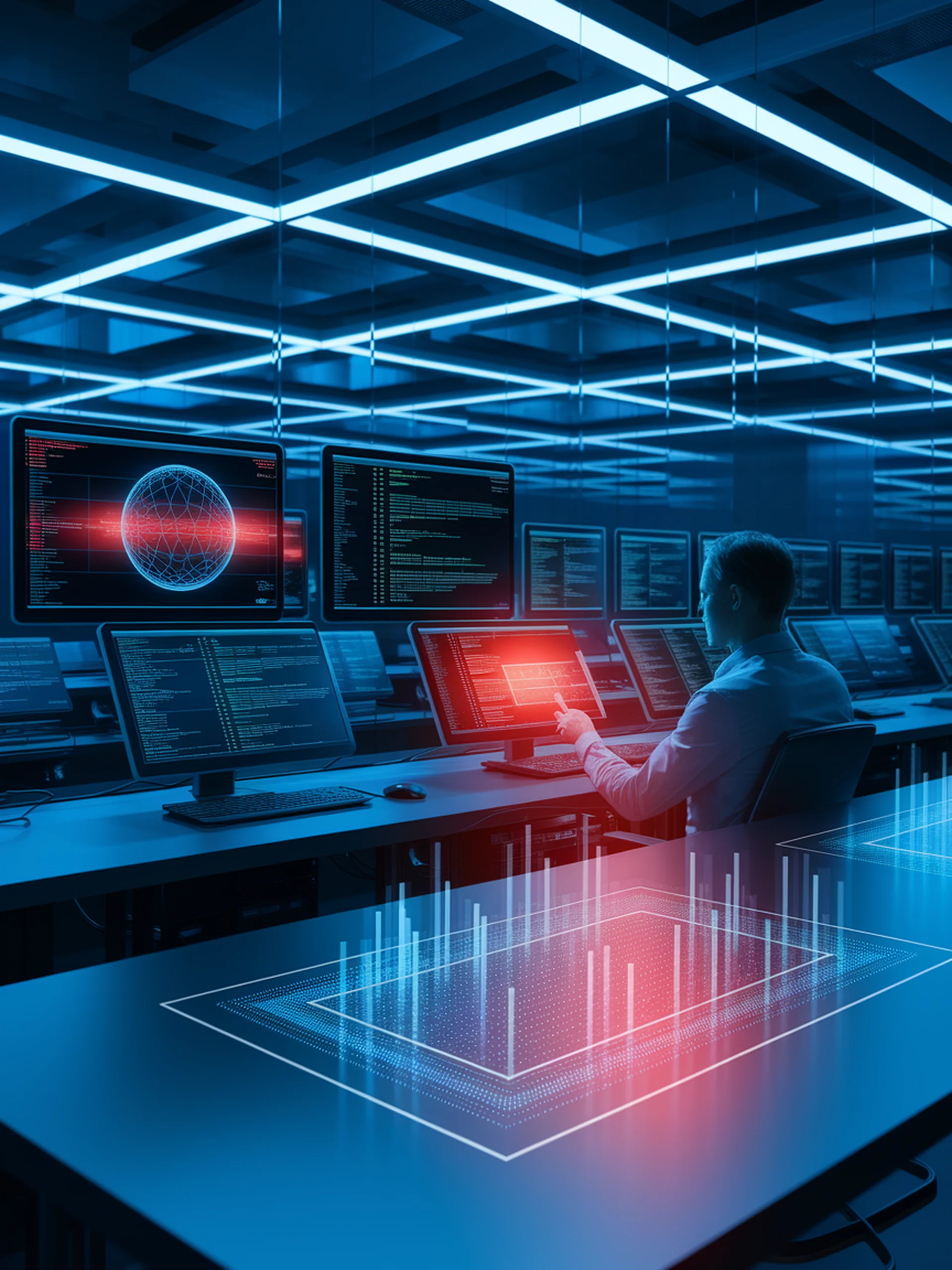
Guarding LLMs Against Jailbreak Attacks
A proactive defense system that identifies harmful queries before they reach the model
GuidelineLLM introduces a novel approach that enables language models to identify potentially harmful queries before generating responses, significantly improving defense against jailbreak attacks.
Key Findings:
- Creates a two-stage response system where models first check for harmful intent before responding
- Achieves superior performance over existing defense methods while requiring less computational resources
- Demonstrates strong generalization capabilities against various types of jailbreak attacks
- Provides a practical solution for enhancing LLM safety in real-world deployments
This research is critical for security teams as it addresses a fundamental vulnerability in LLMs that could be exploited in production systems. By enabling models to proactively identify harmful content, organizations can deploy AI assistants with greater confidence in their alignment with safety guidelines.
Look Before You Leap: Enhancing Attention and Vigilance Regarding Harmful Content with GuidelineLLM