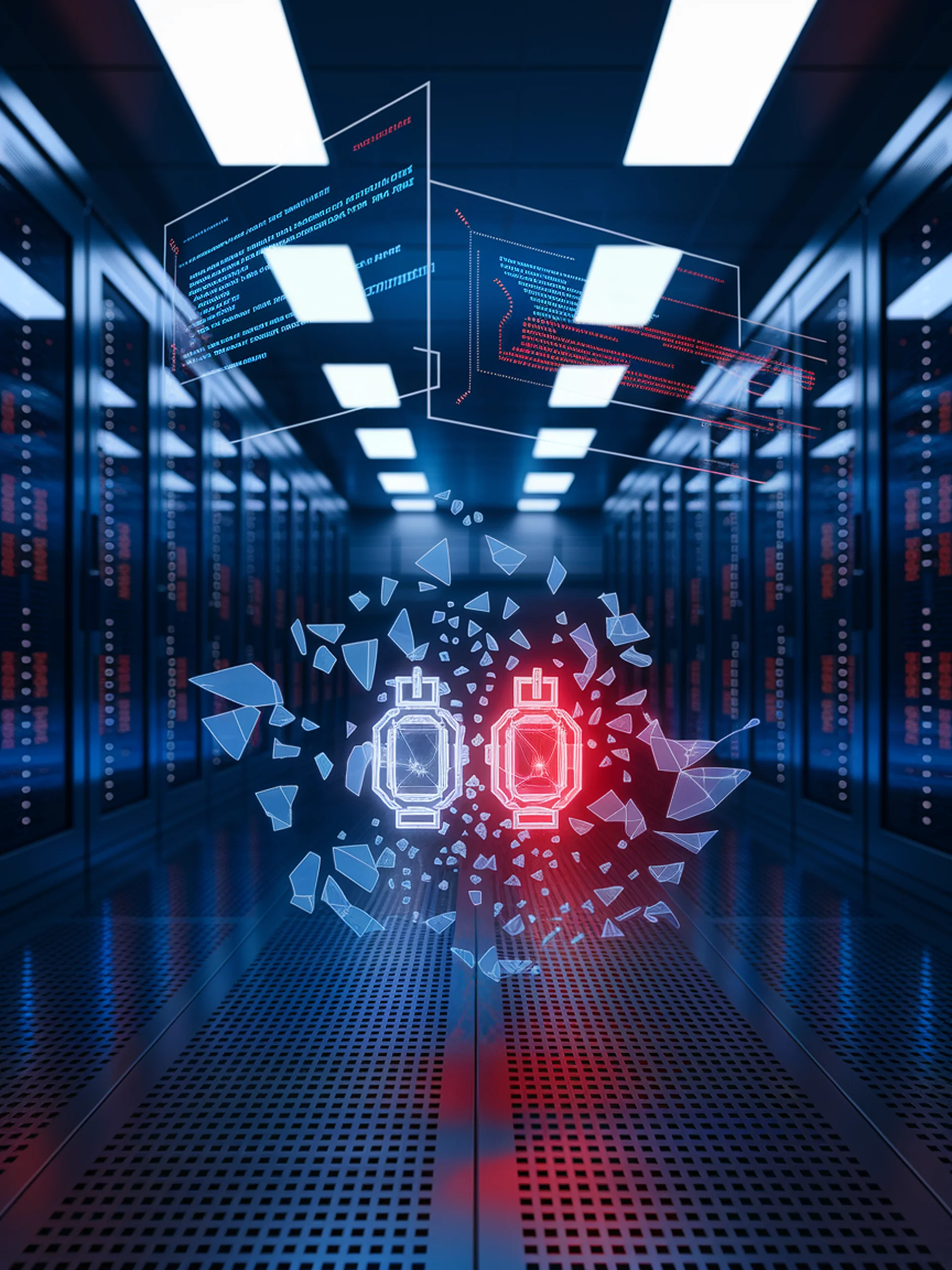
Smarter Jailbreak Attacks on LLMs
Boosting Attack Efficiency with Compliance Refusal Initialization
This research introduces a novel framework called Compliance Refusal Initialization (CRI) that significantly improves the effectiveness of jailbreak attacks against large language models.
- CRI leverages the model's refusal responses to generate better initial attack prompts
- Reduces computational resources needed for successful attacks by 75%
- Improves attack success rates by 20%-40% across various models
- Functions as a model-agnostic approach that can enhance any optimization-based jailbreak method
Security Implications: This work highlights critical vulnerabilities in current LLM safety mechanisms, providing essential insights for developing more robust safeguards against evolving threats. Security teams must understand these attack vectors to build better detection and prevention systems.
Enhancing Jailbreak Attacks via Compliance-Refusal-Based Initialization